FOAD: a novel video anomaly detection focusing on objects
Multimedia Tools and Applications(2024)
摘要
With the popularity of surveillance video, people’s increased security awareness and demand for risk warning, video anomaly detection is gradually gaining widespread attention. Since the amount of data in the background is larger than that of the foreground object, the background information of video frames is more easily noticed by the model, while the abnormal behaviors that cannot be easily detected mostly occur in the foreground. At the same time, if the background information is processed separately from the foreground information, it will inevitably increase the complexity of the model. In this paper, we propose a novel video anomaly detection based on scene object. In the training phase, we put foreground objects on the canvas for training and the memory module remembers various normal patterns of the foreground objects, so that the model can be more sensitive to the anomalies in the foreground objects during detection. Our method is able to achieve AUC 74.01% and 30 FPS on ShanghaiTech dataset. At the same time, several advanced video anomaly detection algorithms are compared, which all demonstrate the superiority of our method.
更多查看译文
关键词
Video anomaly detection,Scene object,Autoencoder,Memory
AI 理解论文
溯源树
样例
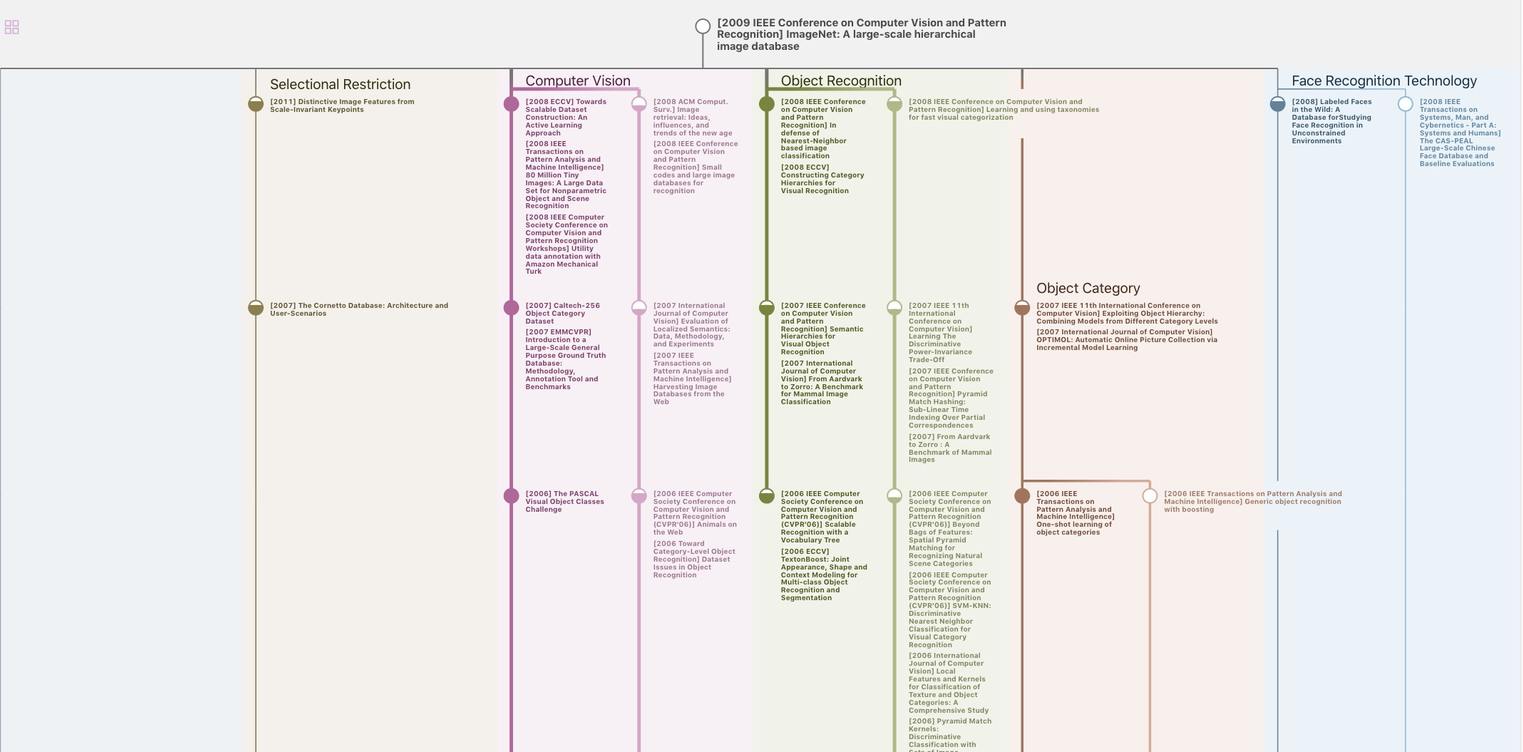
生成溯源树,研究论文发展脉络
Chat Paper
正在生成论文摘要