Convolutional long short-term memory-based approach for deepfakes detection from videos
Multimedia Tools and Applications(2024)
摘要
The great development in the area of Artificial Intelligence (AI) has introduced tremendous advancements in information technology. Moreover, the introduction of lightweight machine learning (ML) techniques allows the applications to work with limited storage and processing power. Deepfakes is among the most famous type of such applications of this era which generates a large amount of fake and modified audiovisual data. The creation of such fake data has introduced a serious risk to the security and confidentiality of humans all around the globe. Accurate detection and classification of actual and deepfakes content is a challenging task due to the progression of Generative adversarial networks (GANs) which produce such convincing manipulated content that it’s impossible for people to recognize it through their naked eyes. In this work, we have presented deep learning (DL)-based approach namely the convolutional long short-term memory (C-LSTM) method for deepfakes detection from videos. More specifically, the spatial information from the input sample is calculated by employing various pre-trained models like VGG16, VGG19, ResNet50, XceptionNet, and GoogleNet, DenseNet. Further, we have proposed a novel feature descriptor called the Dense-Swish-Net121. Whereas the Bi-LSTM model is utilized to compute the temporal information. Lastly, the results are predicted based on both the frame level and temporal level information to make the final decision. A detailed comparison of all CNN models with the Bi-LSTM approach is performed and has confirmed through the reported results that the proposed Dense-Swish-Net121 with Bi-LSTM approach performs well for deepfakes detection.
更多查看译文
关键词
CNN,Deepfakes,Bi-LSTM,Deep learning,Multimedia forensic
AI 理解论文
溯源树
样例
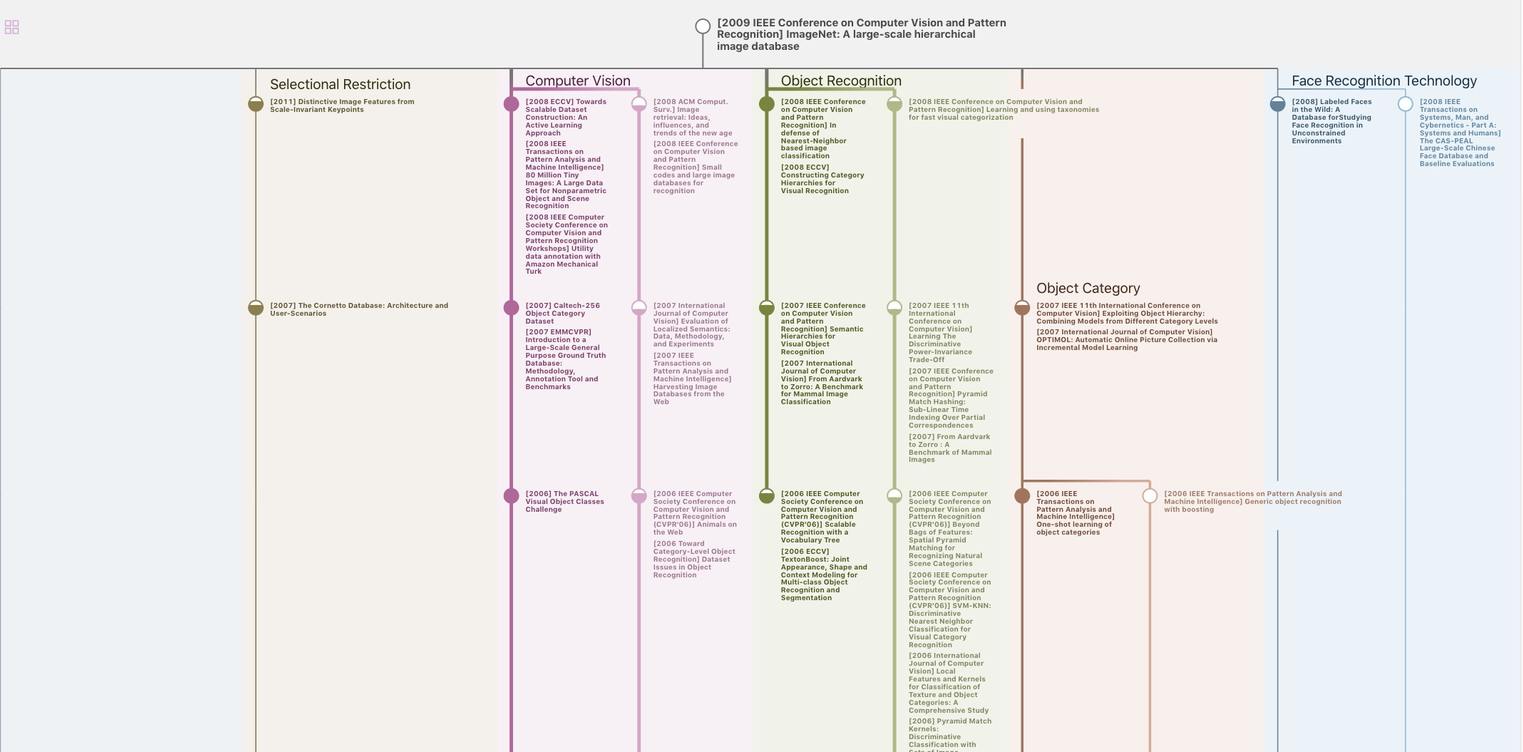
生成溯源树,研究论文发展脉络
Chat Paper
正在生成论文摘要