EV-Perturb: event-stream perturbation for privacy-preserving classification with dynamic vision sensors
Multimedia Tools and Applications(2024)
摘要
The dynamic vision sensors or event-cameras are bio-inspired vision platforms with independent and asynchronous pixels. Their unique design enables a number of advantages over traditional RGB cameras, including high temporal resolution for capturing high speed motion without blur and high dynamic range for sensing under challenging lighting conditions. As the outputs of the event-cameras are discrete and asynchronous events, termed as event-streams, rather than traditional high-quality video frames, they are regarded as low privacy-intrusive. However, research on reconstruction from events has revealed that event-streams can be converted to high quality video frames by sophisticated reconstruction algorithms, so that the claim on privacy-preserving does not hold anymore. In this paper, we focus on the privacy issue of event-streams used in EV-based classification tasks and propose, EV-Perturb , an event-stream perturbation mechanism to protect event-streams from reconstruction attacks. EV-Perturb flips polarities of events in a random manner and the theoretical proof shows that it provides differential-private guarantee on the perturbed event-streams. We also evaluate the utility (classification accuracy) and privacy (video reconstruction error) of EV-Perturb on EV-based classification tasks with multiple publicly available datasets using deep learning models. In summary, this work has several technical contributions. First, by proposing EV-Perturb , we consider the privacy issue of event-streams under reconstruction attack, which is the first piece of work focusing on solving this specific privacy issue. The approach is based on randomized response, which is both efficient and effective, shown as our evaluation. We also provide a theoretical proof that EV-Perturb is differential-private and derive the strict privacy guarantee with respect to the probability of change. Lastly, the results of the extensive evaluations show that EV-Perturb is can effectively protect event-streams from reconstruction attacks while preserving comparable accuracy on classification.
更多查看译文
关键词
Event camera,Differential privacy,Video reconstruction
AI 理解论文
溯源树
样例
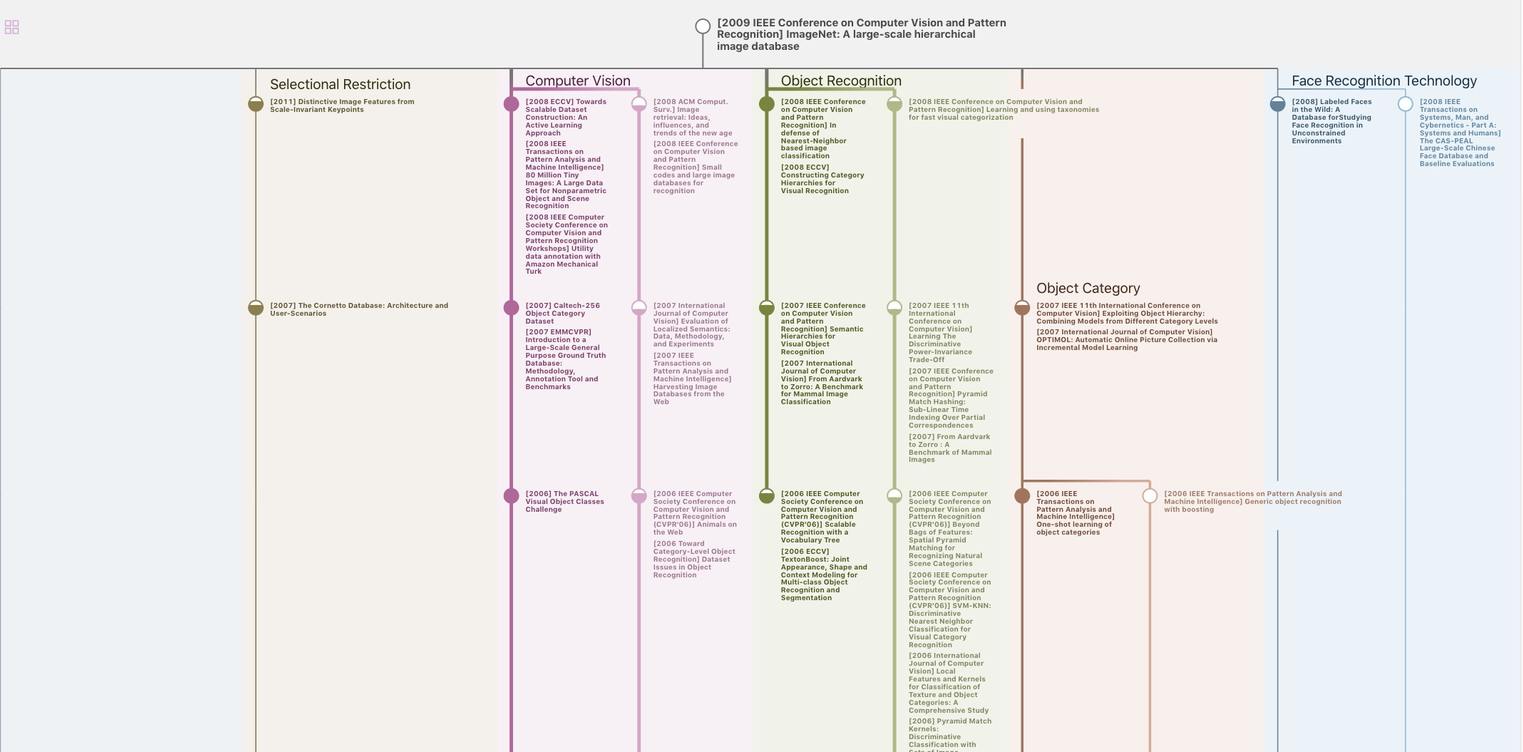
生成溯源树,研究论文发展脉络
Chat Paper
正在生成论文摘要