A bidirectional-a-star-based ant colony optimization algorithm for big-data-driven taxi route recommendation
Multimedia Tools and Applications(2024)
摘要
To address the critical problems of high fuel consumption and severe traffic congestion of brought by blindly cruising in a vast transportation system, we propose a Bidirectional-A-star-based Ant Colony Optimization (BiA*-ACO) algorithm to recommend the fastest route for taxicabs in a complex urban road network with passenger prediction results in this paper. More specifically, the cost estimation function of the Bidirectional A-star (BiA*) algorithm is employed to optimize the heuristic function of the Ant Colony (AC) algorithm for enhancing the global searching ability of ACO. Furthermore, the optimal route obtained from each cycle is introduced to improve the pheromone updating rules of AC for accelerating the convergence speed of ACO. Finally, the BiA*-ACO algorithm is applied to recommend the fastest route successfully. The experimental results of real-world taxi GPS trajectory big data with an urban road network demonstrate that the BiA*-ACO algorithm is at least 47.05% more efficient than the traditional ACO algorithm when the data set is small. As the big GPS trajectory data grows exponentially, the BiA*-ACO algorithm is at least 49.81% more efficient than ACO, Dijkstra, and Bellman-Ford. In particular, compared with the A-star algorithm, the Acyclic algorithm, and the Gurobi algorithm, the fastest route length recommended by the BiA*-ACO algorithm is reduced by 102.73m, 73.27m, and 23.08m.
更多查看译文
关键词
Big data analytics,Image processing,Route recommendation,BiA*,Ant colony optimization,Big taxi trajectory data
AI 理解论文
溯源树
样例
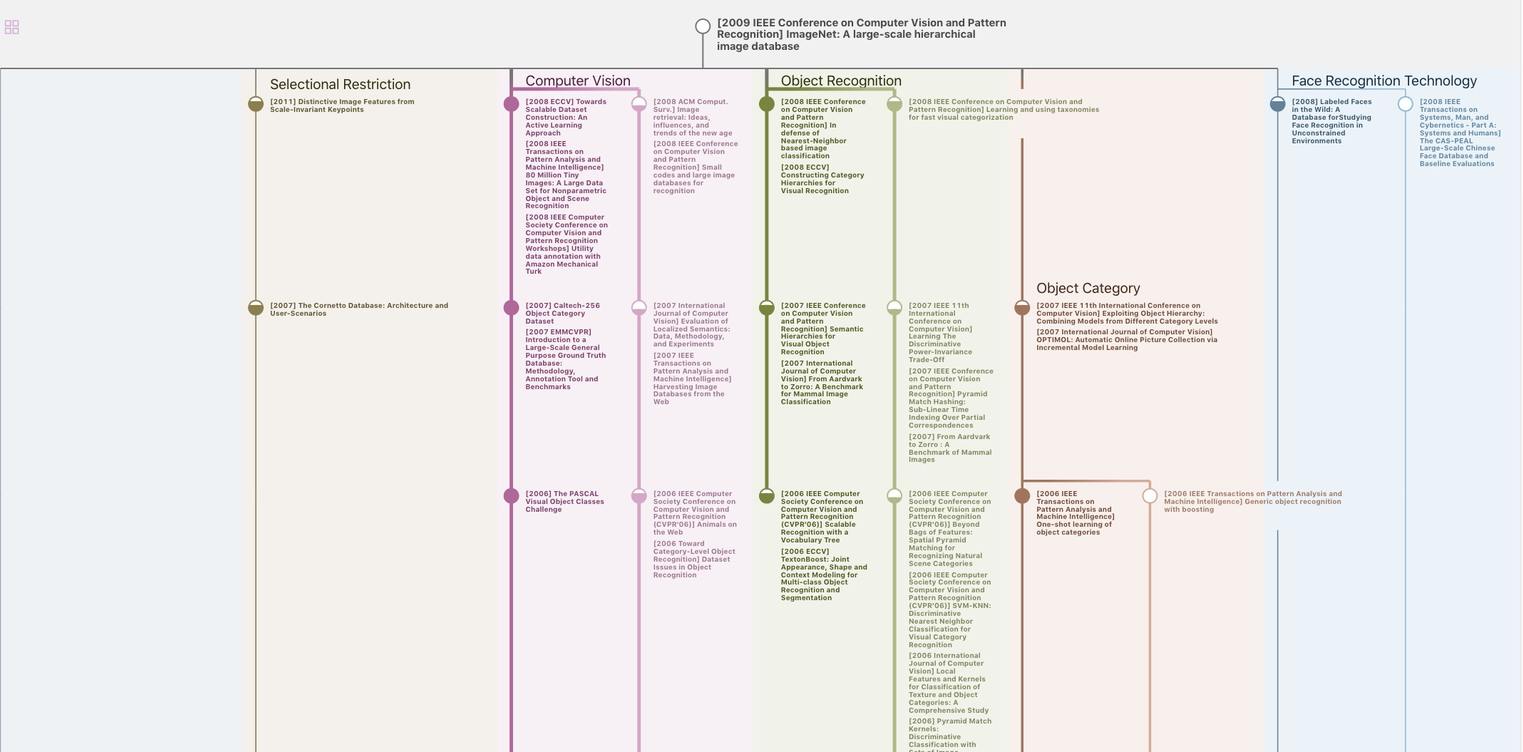
生成溯源树,研究论文发展脉络
Chat Paper
正在生成论文摘要