Generalized semi-supervised class incremental learning in presence of outliers
Multimedia Tools and Applications(2024)
摘要
In this work, we focus on addressing the challenging real-world problem of generalized semi-supervised class-incremental learning (GSS-CIL), which has received relatively little attention in the research community. This involves having a limited number of labeled samples from the new classes at each incremental step, along with a large number of unlabeled samples from these new-classes, previously seen (older-tasks) or completely unseen classes (outliers). Our contributions are three-fold: Firstly, we provide a comprehensive definition and motivation of the GSS-CIL protocol and evaluate the performance of existing state-of-the-art class incremental learning (CIL) methods under this protocol. Secondly, we propose a simple yet effective framework called the Expert-Suggested Pseudo-labelling Network (ESPN) to tackle the GSS-CIL problem by leveraging the information contained in the unlabeled training data. Finally, we use task-wise Harmonic Mean as an additional evaluation metric to capture performance on both new and older tasks. We conduct extensive experiments on three standard large-scale datasets to demonstrate the effectiveness of our proposed ESPN approach, which can serve as a strong baseline for future research in this challenging real-world scenario.
更多查看译文
关键词
Class-incremental learning,Semi-supervised learning,Selective pseudo-labelling
AI 理解论文
溯源树
样例
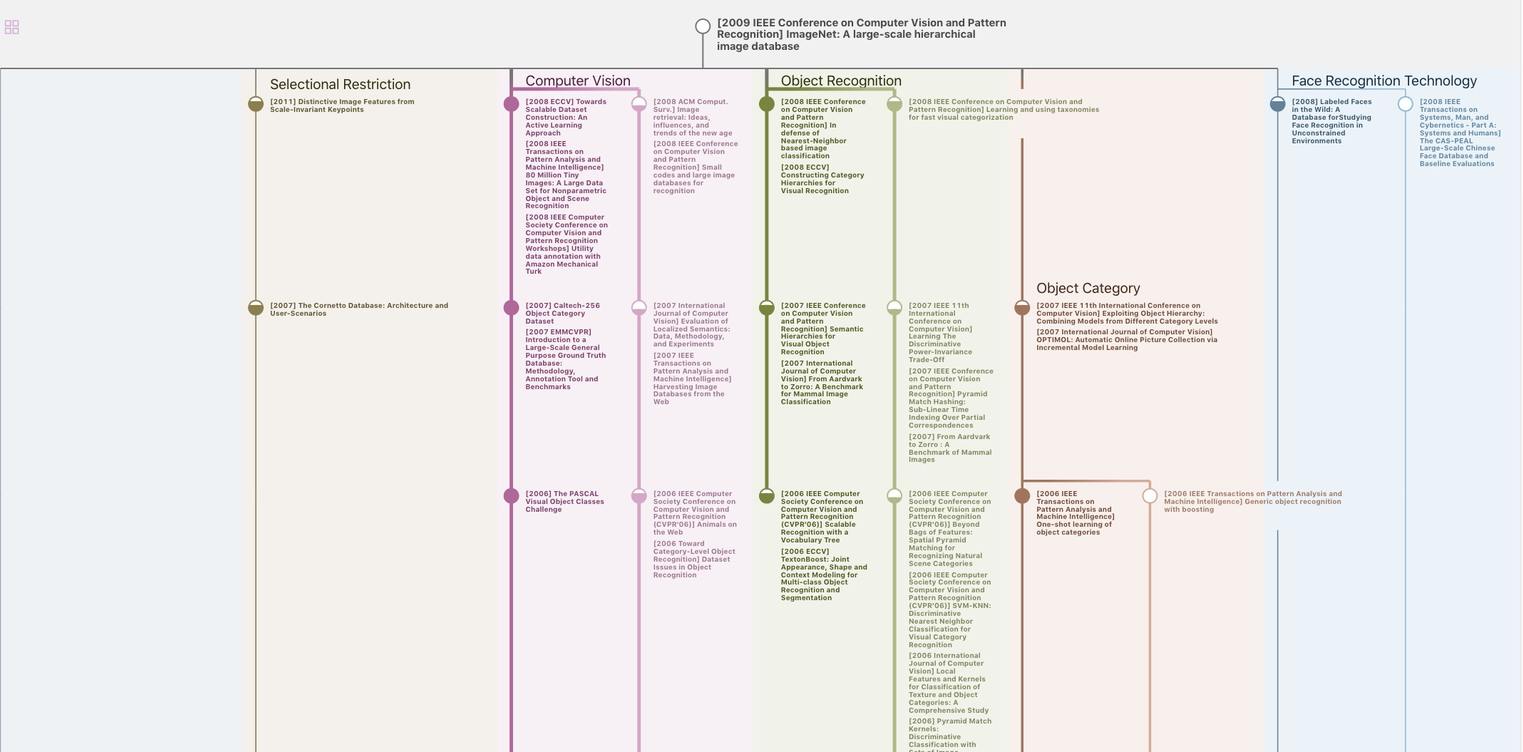
生成溯源树,研究论文发展脉络
Chat Paper
正在生成论文摘要