Towards Consistency Filtering-Free Unsupervised Learning for Dense Retrieval
CoRR(2023)
摘要
Domain transfer is a prevalent challenge in modern neural Information Retrieval (IR). To overcome this problem, previous research has utilized domain-specific manual annotations and synthetic data produced by consistency filtering to finetune a general ranker and produce a domain-specific ranker. However, training such consistency filters are computationally expensive, which significantly reduces the model efficiency. In addition, consistency filtering often struggles to identify retrieval intentions and recognize query and corpus distributions in a target domain. In this study, we evaluate a more efficient solution: replacing the consistency filter with either direct pseudo-labeling, pseudo-relevance feedback, or unsupervised keyword generation methods for achieving consistent filtering-free unsupervised dense retrieval. Our extensive experimental evaluations demonstrate that, on average, TextRank-based pseudo relevance feedback outperforms other methods. Furthermore, we analyzed the training and inference efficiency of the proposed paradigm. The results indicate that filtering-free unsupervised learning can continuously improve training and inference efficiency while maintaining retrieval performance. In some cases, it can even improve performance based on particular datasets.
更多查看译文
关键词
unsupervised learning,consistency,filtering-free
AI 理解论文
溯源树
样例
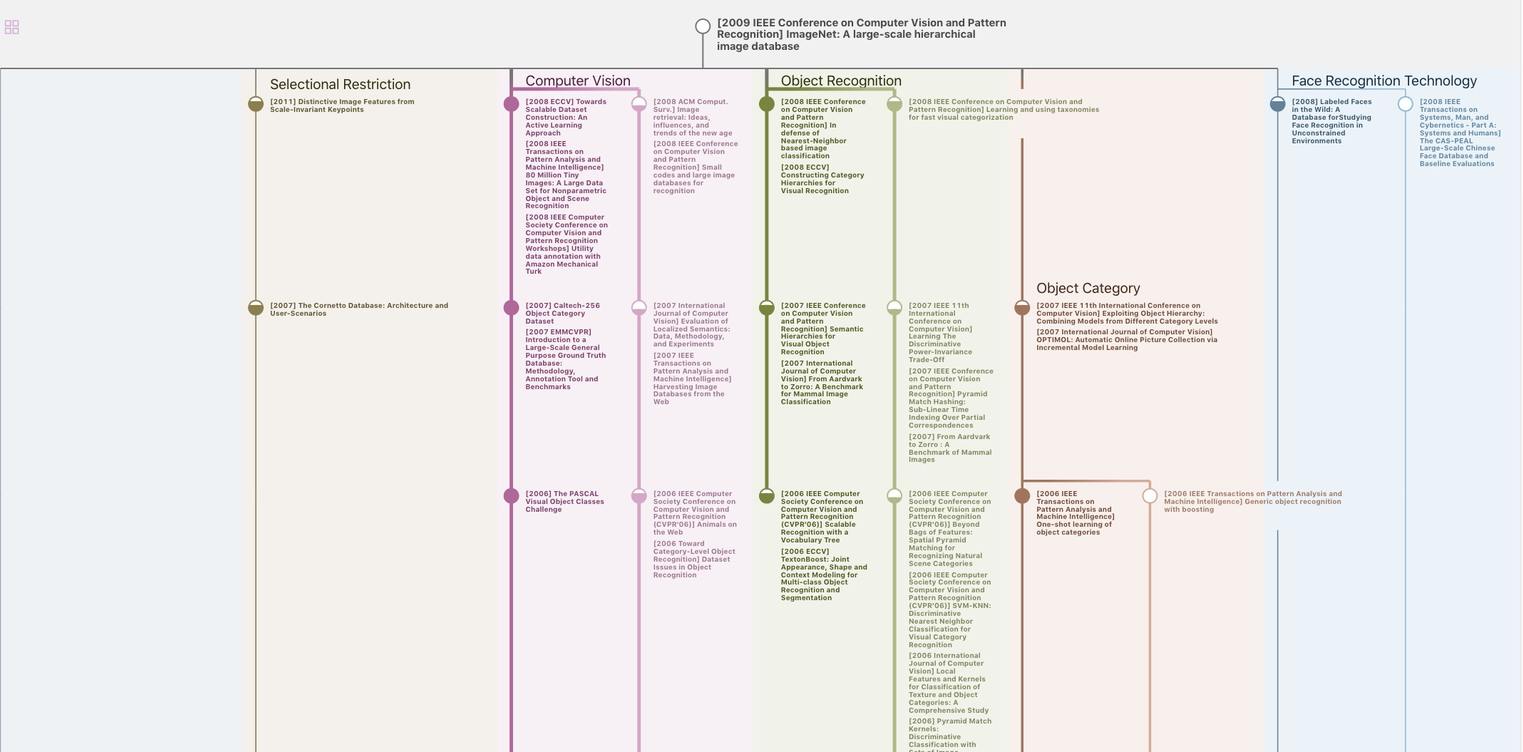
生成溯源树,研究论文发展脉络
Chat Paper
正在生成论文摘要