Average-Hard Attention Transformers are Constant-Depth Uniform Threshold Circuits
CoRR(2023)
摘要
Transformers have emerged as a widely used neural network model for various natural language processing tasks. Previous research explored their relationship with constant-depth threshold circuits, making two assumptions: average-hard attention and logarithmic precision for internal computations relative to input length. Merrill et al. (2022) prove that average-hard attention transformers recognize languages that fall within the complexity class TC0, denoting the set of languages that can be recognized by constant-depth polynomial-size threshold circuits. Likewise, Merrill and Sabharwal (2023) show that log-precision transformers recognize languages within the class of uniform TC0. This shows that both transformer models can be simulated by constant-depth threshold circuits, with the latter being more robust due to generating a uniform circuit family. Our paper shows that the first result can be extended to yield uniform circuits as well.
更多查看译文
关键词
circuits,attention,average-hard,constant-depth
AI 理解论文
溯源树
样例
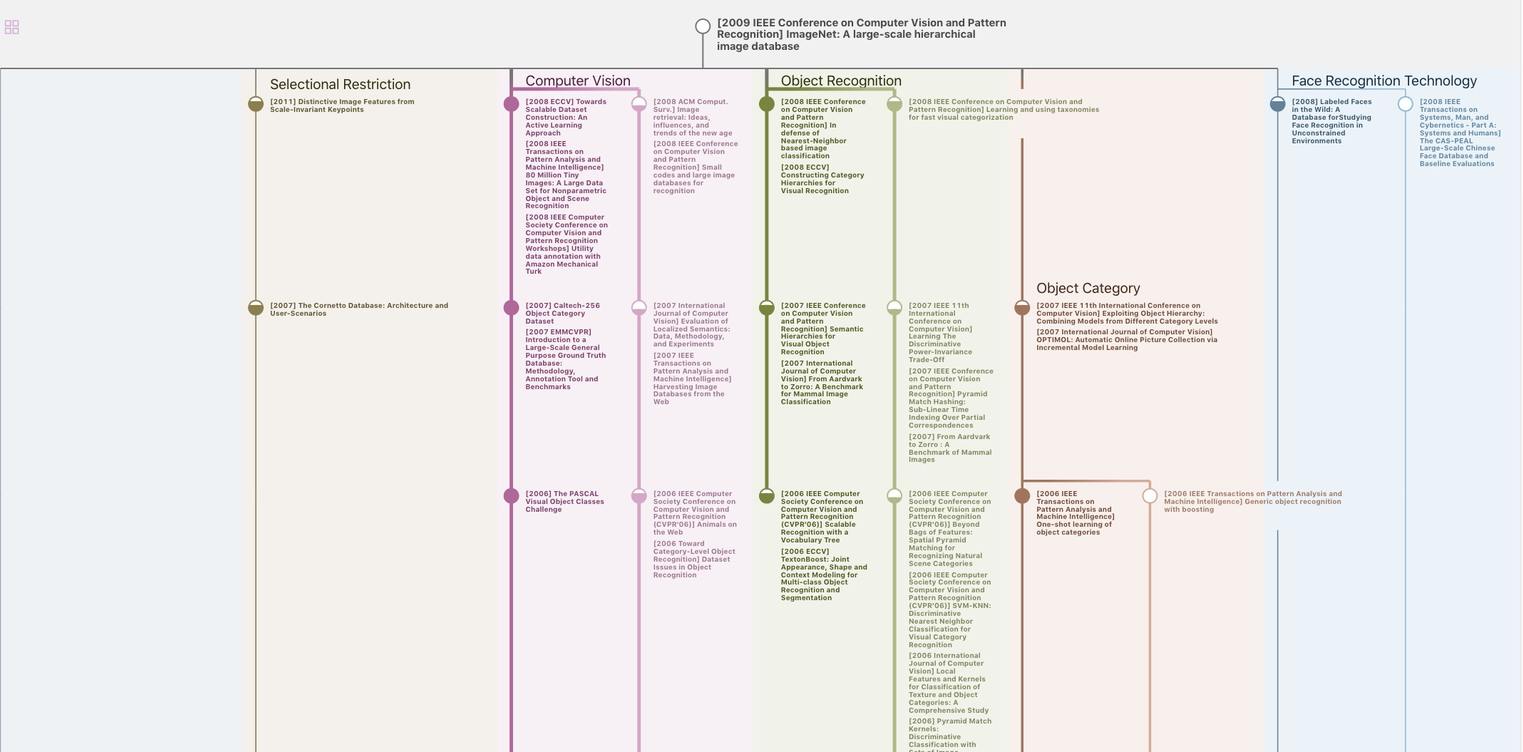
生成溯源树,研究论文发展脉络
Chat Paper
正在生成论文摘要