Binary Federated Learning with Client-Level Differential Privacy
IEEE CONFERENCE ON GLOBAL COMMUNICATIONS, GLOBECOM(2023)
摘要
Federated learning (FL) is a privacy-preserving collaborative learning framework, and differential privacy can be applied to further enhance its privacy protection. Existing FL systems typically adopt Federated Average (FedAvg) as the training algorithm and implement differential privacy with a Gaussian mechanism. However, the inherent privacy-utility trade-off in these systems severely degrades the training performance if a tight privacy budget is enforced. Besides, the Gaussian mechanism requires model weights to be of high-precision. To improve communication efficiency and achieve a better privacy-utility trade-off, we propose a communication-efficient FL training algorithm with differential privacy guarantee. Specifically, we propose to adopt binary neural networks (BNNs) and introduce discrete noise in the FL setting. Binary model parameters are uploaded for higher communication efficiency and discrete noise is added to achieve the client-level differential privacy protection. The achieved performance guarantee is rigorously proved, and it is shown to depend on the level of discrete noise. Experimental results based on MNIST and Fashion-MNIST datasets will demonstrate that the proposed training algorithm achieves client-level privacy protection with performance gain while enjoying the benefits of low communication overhead from binary model updates.
更多查看译文
关键词
Federated Learning,Differential Privacy,Neural Network,Model Parameters,Model Weights,Training Algorithm,Privacy Protection,Updated Model,Efficient Communication,Communication Overhead,MNIST Dataset,Binary Network,Privacy Guarantee,Loss Function,Training Data,Black Box,Output Layer,Gaussian Noise,Data Privacy,Stochastic Gradient Descent,Local Dataset,Individual Updates,Local Updates,Auxiliary Parameters,Threat Model,Federated Learning Framework,Training Round,Loss Of Privacy,Random Responses,Multi-party Computation
AI 理解论文
溯源树
样例
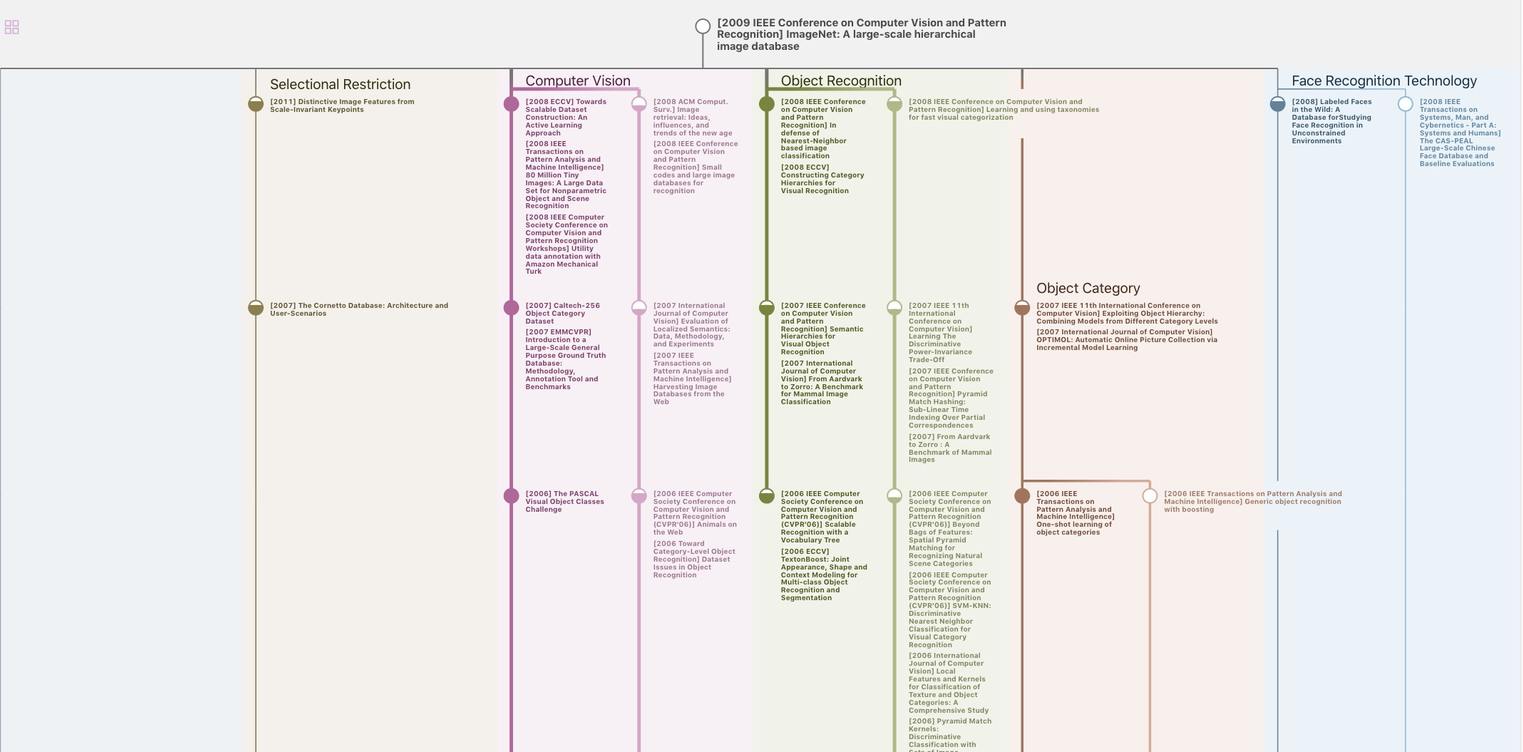
生成溯源树,研究论文发展脉络
Chat Paper
正在生成论文摘要