A Theory of Evidence Lower Bound and Its Application to Super-Resolution Data Assimilation (SRDA) Using Conditional Variational Autoencoders
arXiv (Cornell University)(2023)
摘要
This study proposes a framework that concurrently optimizes the assimilation and generation processes of observation data. This is implemented with a conditional variational autoencoder (CVAE) composed of an encoder and a decoder. The encoder performs assimilation by estimating the true state from the background state and observations at a given time, whereas the decoder serves as an observation operator and generates observations from the true state. The loss function for the unsupervised learning of CVAEs is regarded as an objective function for three-dimensional variational (3D-Var) assimilation, providing a theoretical basis for the use of CVAEs. Furthermore, by leveraging the arbitrariness of the prior distribution, the proposed framework is applied to super-resolution data assimilation (SRDA). Specifically, the background state is super-resolved by an SR model and then used in training the CVAE. This approach allows the encoder to execute SR and DA simultaneously. The effectiveness of the proposed SRDA is validated via numerical experiments on an idealized barotropic ocean jet system. The SR model can be trained using the observations as target data, despite most grid points being missing. This achievement is attributed to the translation equivariance of convolution. Comparisons with an ensemble Kalman filter show the superior performance and reduced computational cost of the SRDA. Importantly, the SRDA can infer high-resolution forecasts at any time step through the combination of the SR model and CVAE. This research provides a theoretical foundation and motivation for the use of deep generative models in DA.
更多查看译文
关键词
data assimilation,conditional variational autoencoders,srda,evidence lower bound,super-resolution
AI 理解论文
溯源树
样例
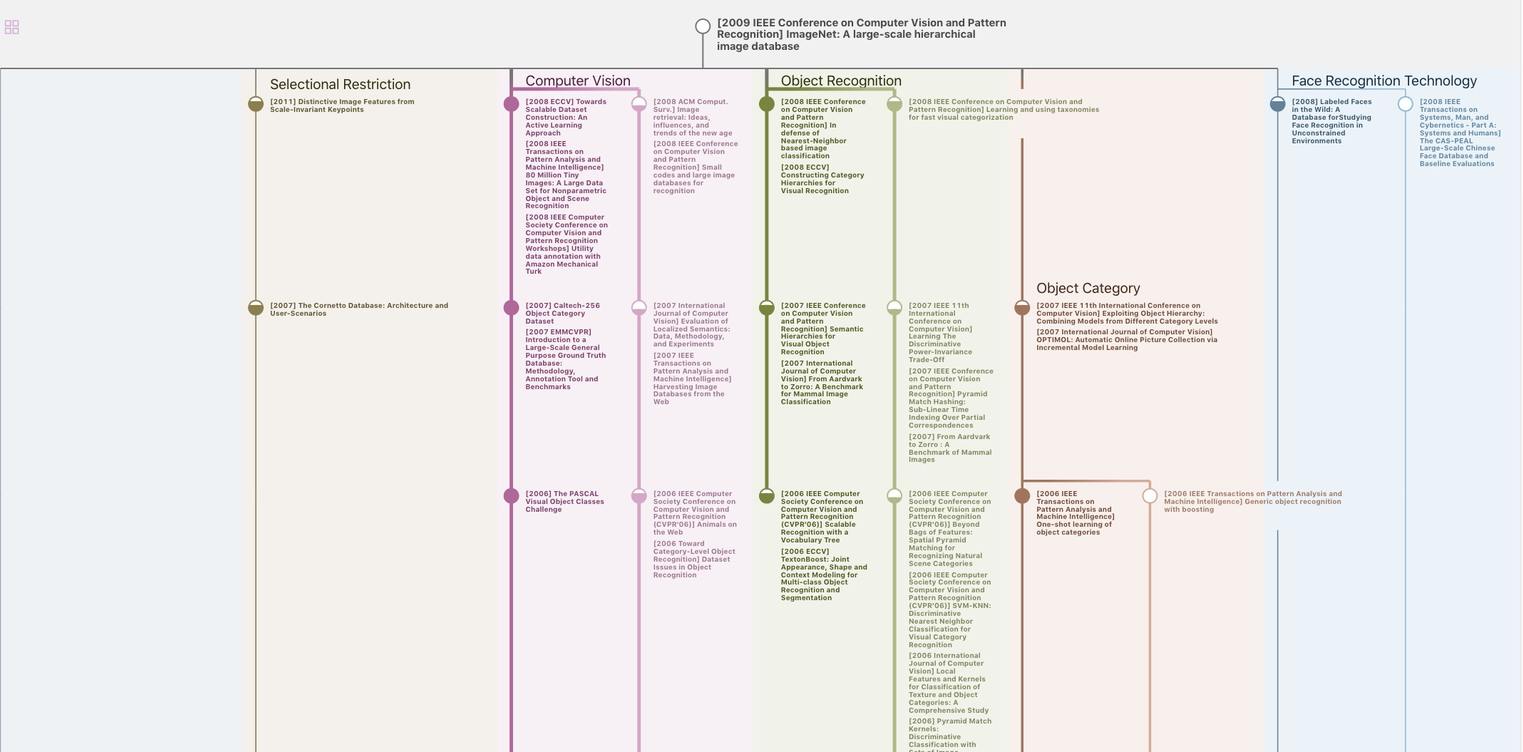
生成溯源树,研究论文发展脉络
Chat Paper
正在生成论文摘要