No Length Left Behind: Enhancing Knowledge Tracing for Modeling Sequences of Excessive or Insufficient Lengths
PROCEEDINGS OF THE 32ND ACM INTERNATIONAL CONFERENCE ON INFORMATION AND KNOWLEDGE MANAGEMENT, CIKM 2023(2023)
摘要
Knowledge tracing (KT) aims to predict students' responses to practices based on their historical question-answering behaviors. However, most current KT methods focus on improving overall AUC, leaving ample room for optimization in modeling sequences of excessive or insufficient lengths. As sequences get longer, computational costs will increase exponentially. Therefore, KT methods usually truncate sequences to an acceptable length, which makes it difficult for models on online service systems to capture complete historical practice behaviors of students with too long sequences. Conversely, modeling students with short practice sequences using most KT methods may result in overfitting due to limited observation samples. To address the above limitations, we propose a model called Sequence-Flexible Knowledge Tracing (SFKT). Specifically, to flexibly handle long sequences, SFKT introduces a total-term encoder to effectively model complete historical practice behaviors of students at an affordable computational cost. Additionally, to improve the prediction accuracy of students with short practice sequences, we introduce a contrastive learning task and data augmentation schema to improve the generality of modeling short sequences by constructing more learning objectives. Extensive experimental results show that SFKT achieves significant improvements over multiple benchmarks, demonstrating the value of exploring the modeling of sequences of excessive or insufficient lengths. Our code is available at https://github.com/zmy-9/SFKT.
更多查看译文
关键词
Long and Short Sequence Modeling,Knowledge Tracing,Educational Data Mining,Self-supervised Learning
AI 理解论文
溯源树
样例
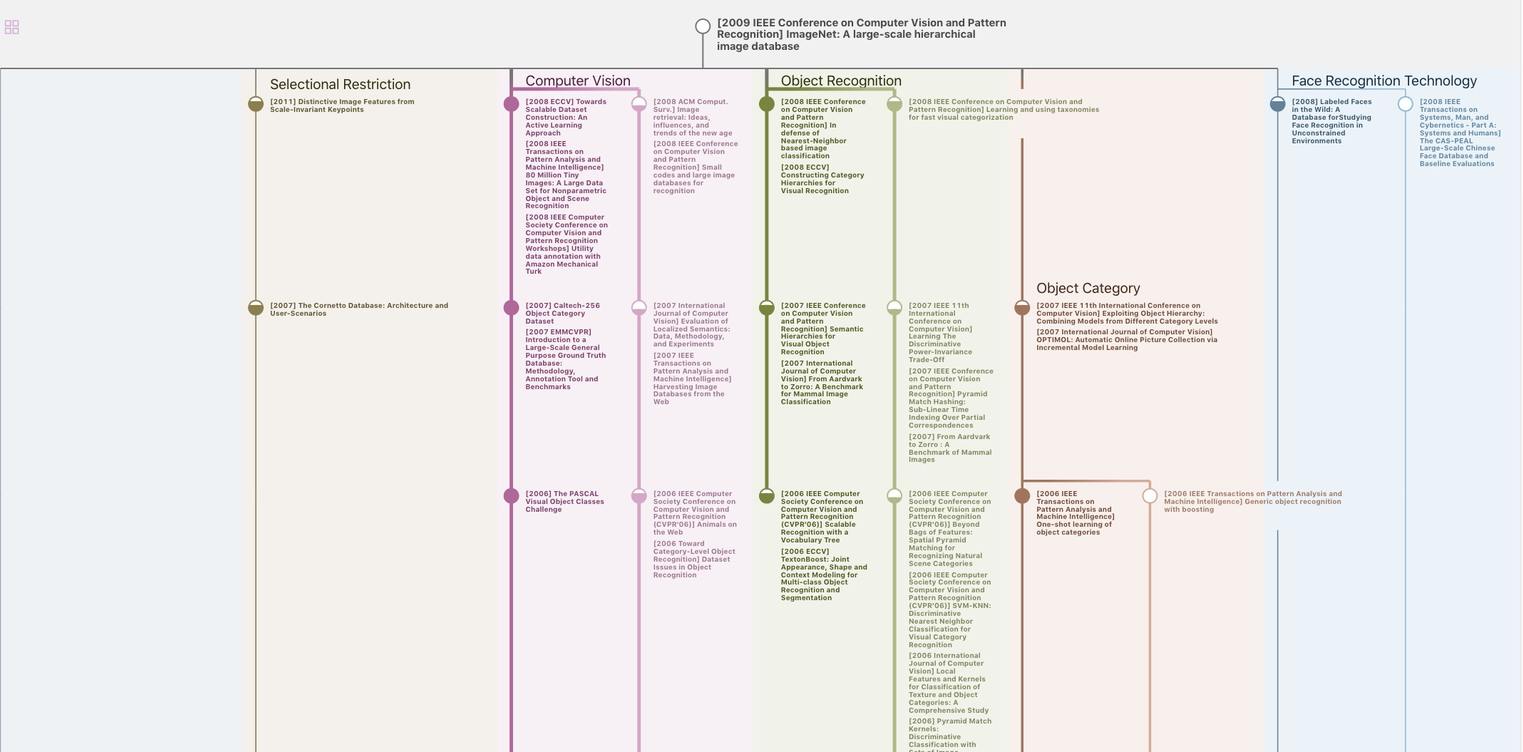
生成溯源树,研究论文发展脉络
Chat Paper
正在生成论文摘要