Generalized Early Stopping in Evolutionary Direct Policy Search
arXiv (Cornell University)(2023)
摘要
Lengthy evaluation times are common in many optimization problems such as direct policy search tasks, especially when they involve conducting evaluations in the physical world, e.g. in robotics applications. Often, when evaluating a solution over a fixed time period, it becomes clear that the objective value will not increase with additional computation time (for example, when a two-wheeled robot continuously spins on the spot). In such cases, it makes sense to stop the evaluation early to save computation time. However, most approaches to stop the evaluation are problem-specific and need to be specifically designed for the task at hand. Therefore, we propose an early stopping method for direct policy search. The proposed method only looks at the objective value at each time step and requires no problem-specific knowledge. We test the introduced stopping criterion in five direct policy search environments drawn from games, robotics, and classic control domains, and show that it can save up to 75% of the computation time. We also compare it with problem-specific stopping criteria and demonstrate that it performs comparably while being more generally applicable.
更多查看译文
关键词
early stopping,policy,search
AI 理解论文
溯源树
样例
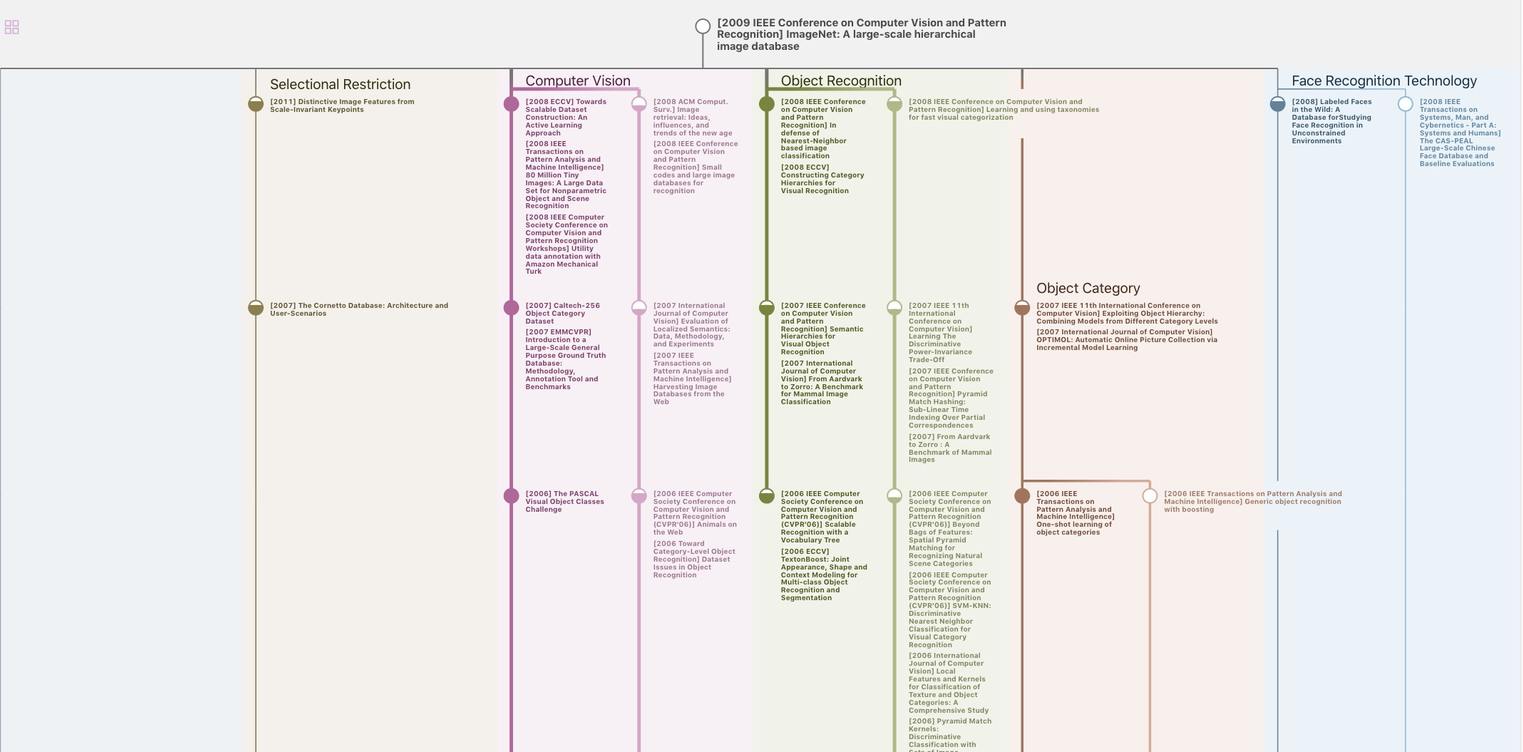
生成溯源树,研究论文发展脉络
Chat Paper
正在生成论文摘要