Nearly d-Linear Convergence Bounds for Diffusion Models via Stochastic Localization
ICLR 2024(2023)
摘要
Denoising diffusions are a powerful method to generate approximate samples
from high-dimensional data distributions. Recent results provide polynomial
bounds on their convergence rate, assuming L^2-accurate scores. Until now,
the tightest bounds were either superlinear in the data dimension or required
strong smoothness assumptions. We provide the first convergence bounds which
are linear in the data dimension (up to logarithmic factors) assuming only
finite second moments of the data distribution. We show that diffusion models
require at most Õ(d log^2(1/δ)/ε^2) steps to
approximate an arbitrary distribution on ℝ^d corrupted with Gaussian
noise of variance δ to within ε^2 in KL divergence. Our
proof extends the Girsanov-based methods of previous works. We introduce a
refined treatment of the error from discretizing the reverse SDE inspired by
stochastic localization.
更多查看译文
关键词
diffusion models,score-based generative models,convergence bounds,stochastic localization
AI 理解论文
溯源树
样例
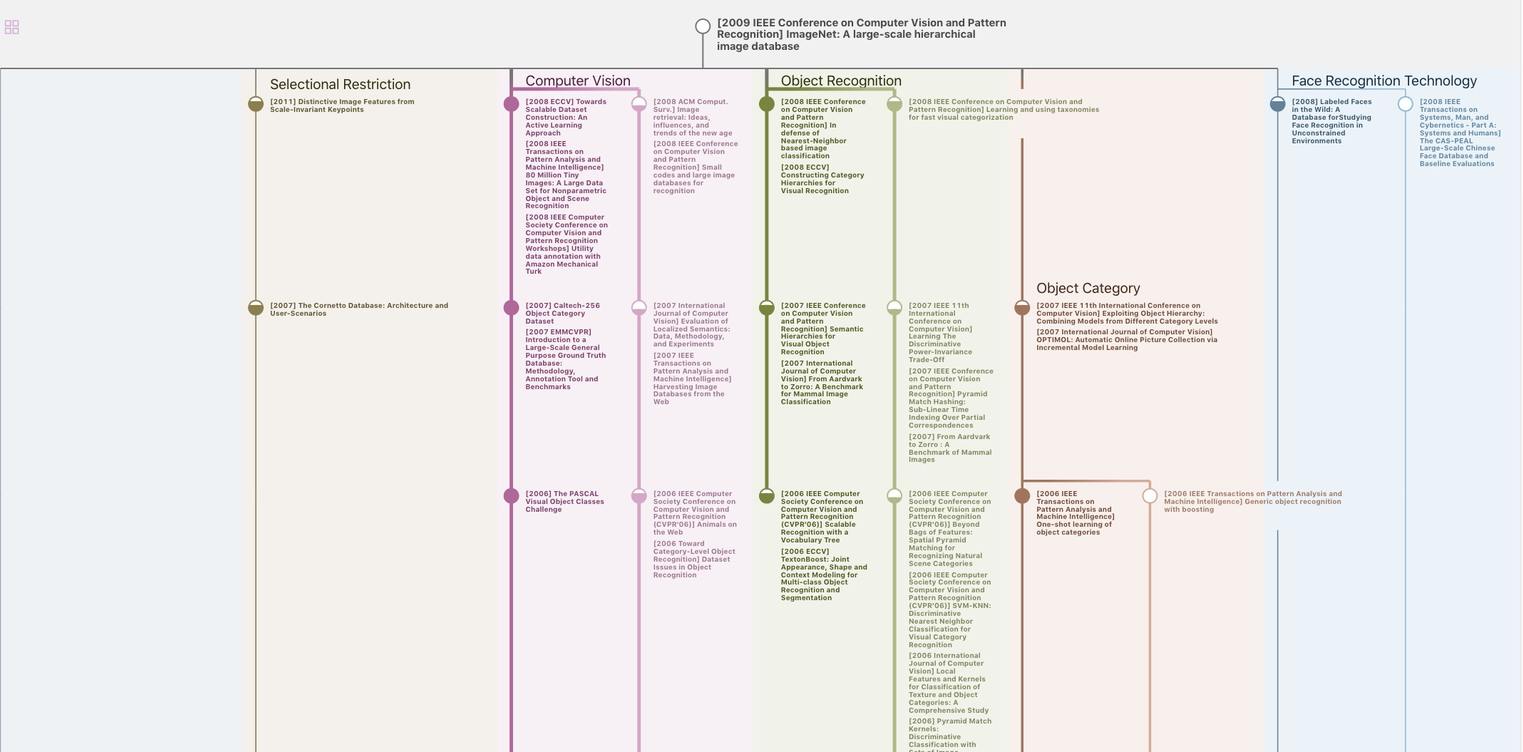
生成溯源树,研究论文发展脉络
Chat Paper
正在生成论文摘要