Semi-Supervised Tree Species Classification for Multi-Source Remote Sensing Images Based on a Graph Convolutional Neural Network
FORESTS(2023)
摘要
As a current research hotspot, graph convolution networks (GCNs) have provided new opportunities for tree species classification in multi-source remote sensing images. To solve the challenge of limited label information, a new tree species classification model was proposed by using the semi-supervised graph convolution fusion method for hyperspectral images (HSIs) and multispectral images (MSIs). In the model, the graph-based attribute features and pixel-based features are fused to deepen the correlation of multi-source images to improve accuracy. Firstly, the model employs the canonical correlation analysis (CCA) method to maximize the correlation of multi-source images, which explores the relationship between information from various sources further and offers more valuable insights. Secondly, convolution calculations were made to extract features and then map graph node fusion, which not only reduces redundancy features but also enhances compelling features. Finally, the relationship between representative descriptors is captured through the use of hyperedge convolution in the training process, and the dominant features on the graph are fully mined. The tree species are classified through two fusion feature operations, leading to improved classification performance compared to state-of-the-art methods. The fusion strategy can produce a complete classification map of the study areas.
更多查看译文
关键词
hypergraph convolution, data fusion, classification of tree species
AI 理解论文
溯源树
样例
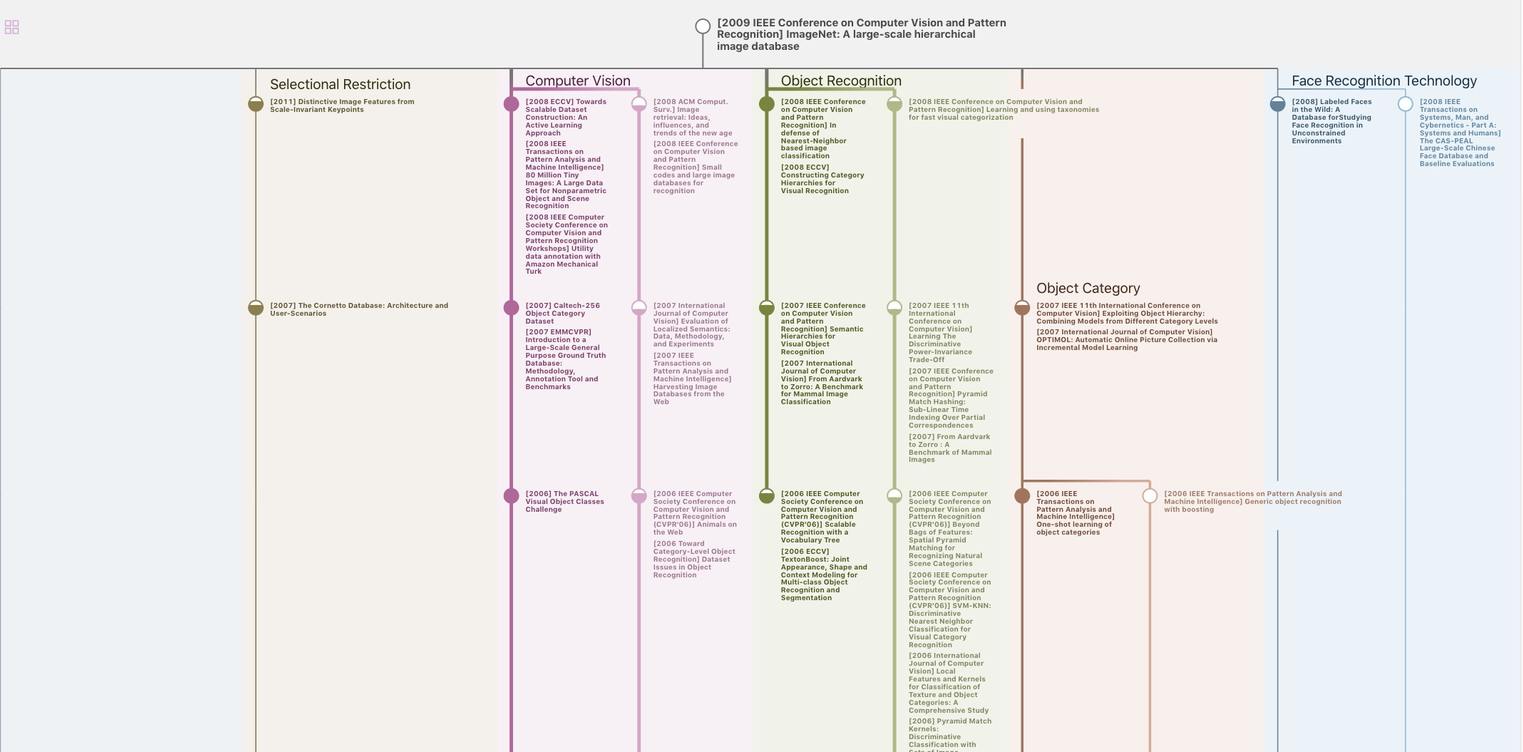
生成溯源树,研究论文发展脉络
Chat Paper
正在生成论文摘要