Combined High-Throughput DFT and ML Screening of Transition Metal Nitrides for Electrochemical CO2 Reduction
ACS CATALYSIS(2023)
摘要
Theelectrochemical reduction of CO2 (CO2RR) usingrenewable electricity has the potential to reduceatmosphericCO(2) levels while producing valuable chemicals and fuels.However, the practical implementation of this technology is limitedby the activity, selectivity, and stability of catalyst materials.In this study, we employ high-throughput density functional theory(DFT) calculations to screen & SIM;800 transition metal nitridesand identify potential catalysts for CO2RR. The stabilityand activity of the screened materials were thoroughly evaluated viathermodynamic analysis, revealing Co, Cr, and Ti transition metalnitrides as the most promising candidates. Additionally, we conducta feature importance analysis using machine learning (ML) regressionmodels for binding energy prediction and determine the primary factorsinfluencing the stability of catalysts. We show that the group numberof metals has a significant impact on the binding energy of *OH andthus on the stability of the catalysts. We anticipate that this combinedapproach of high-throughput DFT screening and design strategy derivedfrom ML regression analysis could effectively lead to the discoveryof improved energy materials.
更多查看译文
关键词
transition metal nitrides,metal nitrides,electrochemical combined,transition metal,dft,high-throughput
AI 理解论文
溯源树
样例
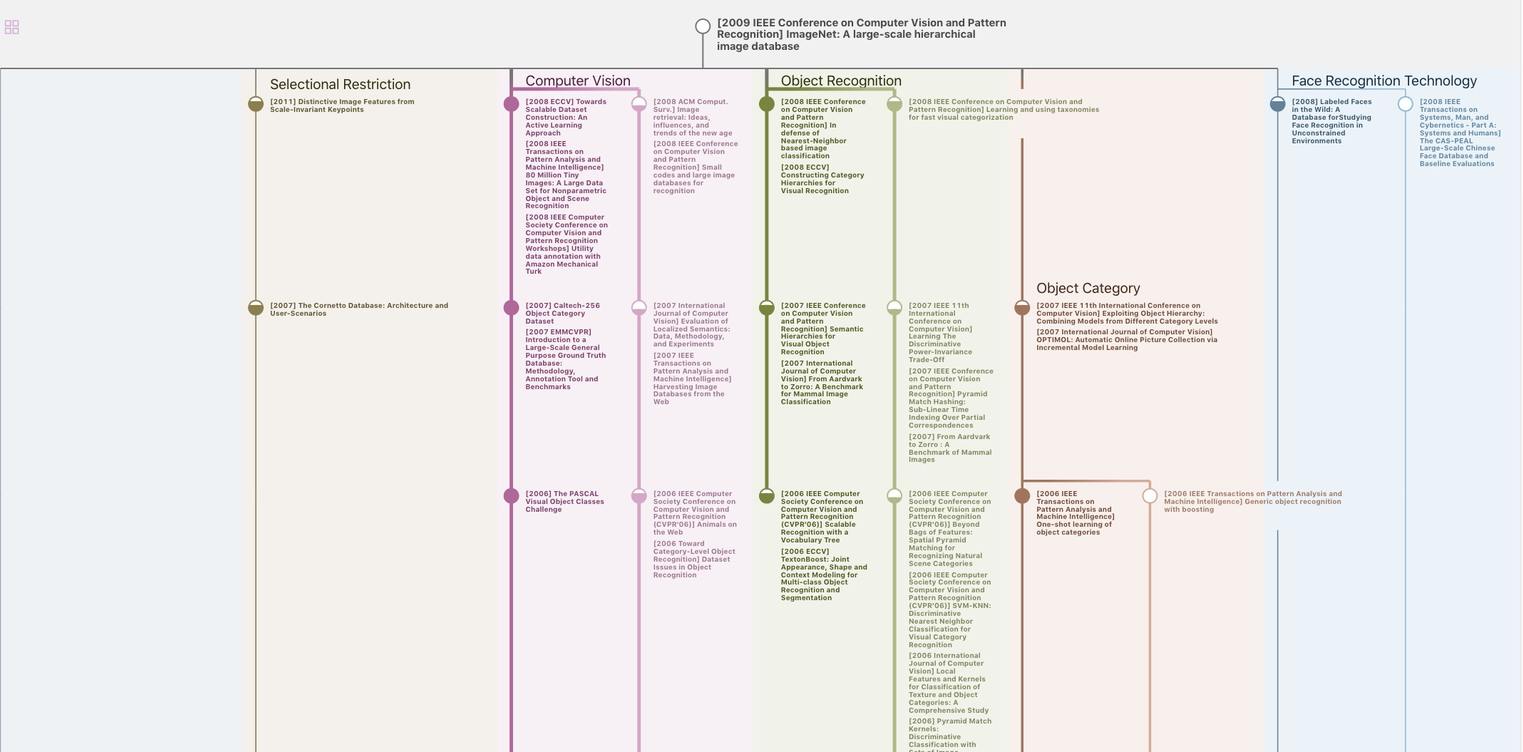
生成溯源树,研究论文发展脉络
Chat Paper
正在生成论文摘要