Application of Minnan Folk Light and Shadow Animation in Built Environment in Object Detection Algorithm
BUILDINGS(2023)
摘要
To resolve the problems of deep convolutional neural network models with many parameters and high memory resource consumption, a lightweight network-based algorithm for building detection of Minnan folk light synthetic aperture radar (SAR) images is proposed. Firstly, based on the rotating target detection algorithm R-centernet, the Ghost ResNet network is constructed to reduce the number of model parameters by replacing the traditional convolution in the backbone network with Ghost convolution. Secondly, a channel attention module integrating width and height information is proposed to enhance the network's ability to accurately locate salient regions in folk light images. Content-aware reassembly of features (CARAFE) up-sampling is used to replace the deconvolution module in the network to fully incorporate feature map information during up-sampling to improve target detection. Finally, the constructed dataset of rotated and annotated light and shadow SAR images is trained and tested using the improved R-centernet algorithm. The experimental results show that the improved algorithm improves the accuracy by 3.8%, the recall by 1.2% and the detection speed by 12 frames/second compared with the original R-centernet algorithm.
更多查看译文
关键词
light and shadow animation, convolutional neural network, mechanism of attention, Minnan folk image, Ghost convolution
AI 理解论文
溯源树
样例
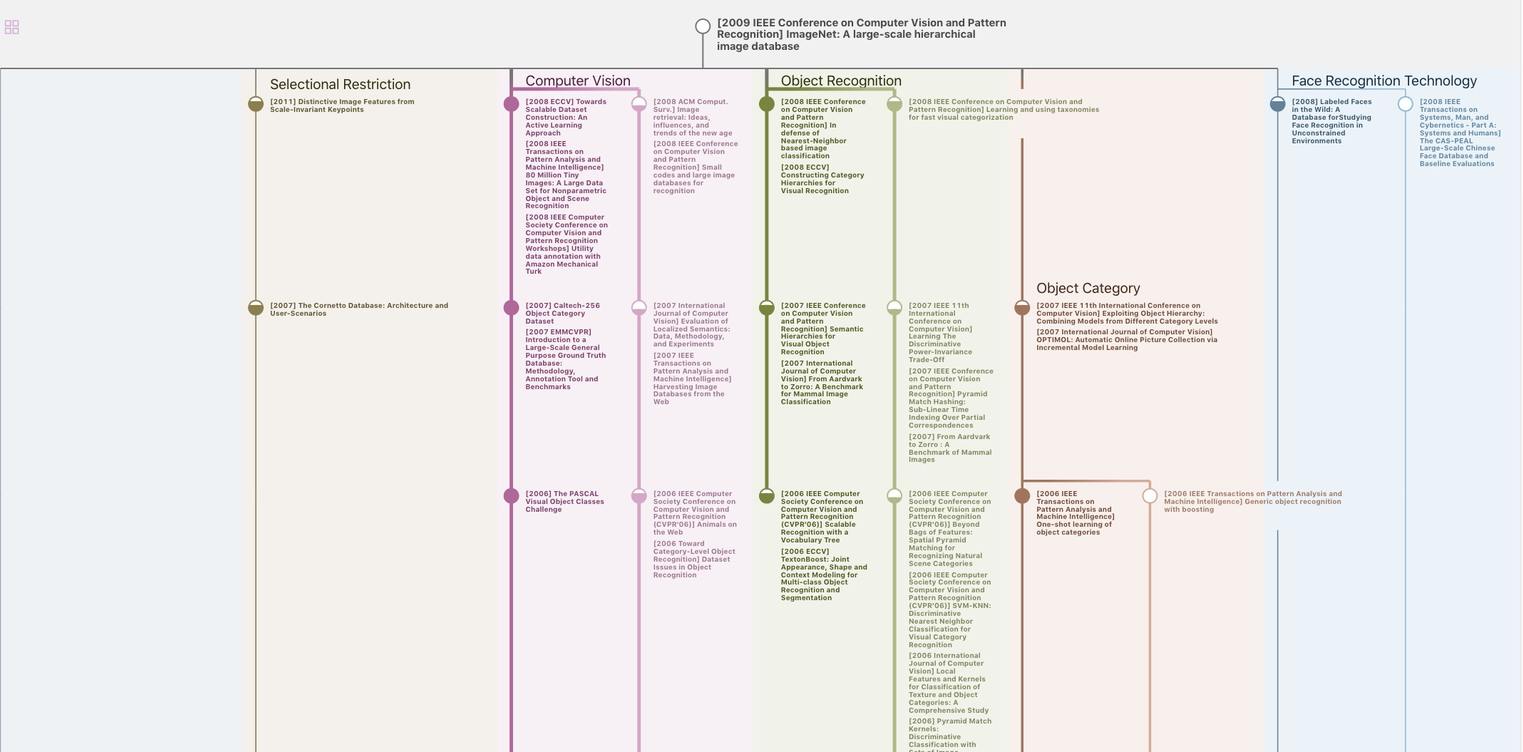
生成溯源树,研究论文发展脉络
Chat Paper
正在生成论文摘要