Fastformer: Transformer-Based Fast Reasoning Framework
FOURTEENTH INTERNATIONAL CONFERENCE ON GRAPHICS AND IMAGE PROCESSING, ICGIP 2022(2022)
摘要
Video action recognition is a vital task in the field of computer vision. A great deal of redundant information is generated along with original video data in the process of depth computation. In order to solve this problem, most existing methods improve recognition speed at the cost of recognition accuracy. In this paper, we propose a new framework: Fastformer which is a transformer-based structure for fast inference video classification to further improve model inference speed while maintaining accuracy. To achieve the balance of speed and accuracy, we solve the inter-frame and intra-frame redundancy of video and design a new self-attention network, which uses the improved highway network to make the model realize the same function as the traditional self-attention module, while greatly reducing the amount of calculation and the number of required parameters. We conduct experiments to verify the effect of our model. Overall, Fastformer significantly outperforms existing vision transformers with regard to the speed versus accuracy trade-off. For example, at 76.4% Keyframes-400 accuracy, Fastformer is 28% faster than TimeSformer.
更多查看译文
关键词
Action recognition,highway network,self-attention,transformer
AI 理解论文
溯源树
样例
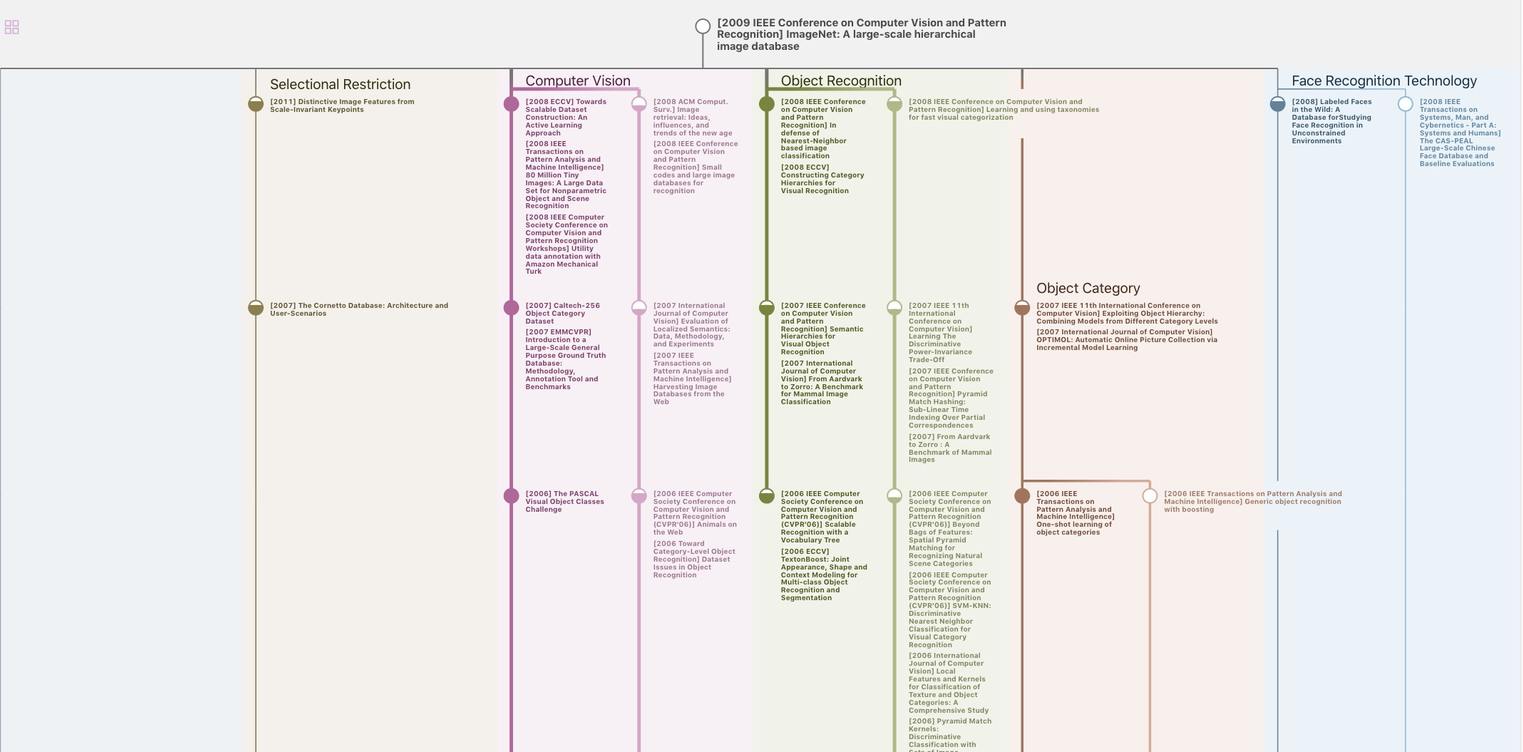
生成溯源树,研究论文发展脉络
Chat Paper
正在生成论文摘要