D2D Resource Allocation Based on Reinforcement Learning and QoS
Mobile Networks and Applications(2023)
摘要
Device-to-device (D2D) communications is designed to improve the overall network performance, including low latency, high data rates, and system capacity of the fifth-generation (5G) wireless networks. The system capacity can even be improved by reusing resources between D2D user equipments (DUEs) and cellular user equipments (CUEs) without causing harmful interference to the CUEs. A D2D resource allocation scheme is expected to have the characteristic that one CUE be allocated with a variable number of resource blocks (RBs), and the RBs be reused by more than one DUE. In this study, the Multi-Player Multi-Armed Bandit (MPMAB) reinforcement learning scheme is employed to model such a problem by establishing a preference matrix to facilitate greedy resource allocation. A fair resource allocation scheme is then proposed and shown to achieve fairness, prevent waste of resources, and alleviate starvation. Moreover, this scheme has better performance when there are not too many D2D pairs.
更多查看译文
关键词
Device-to-device (D2D),Resource allocation,Reinforcement learning,Multi-Player Multi-Armed Bandit (MPMAB),Dynamic resource allocation
AI 理解论文
溯源树
样例
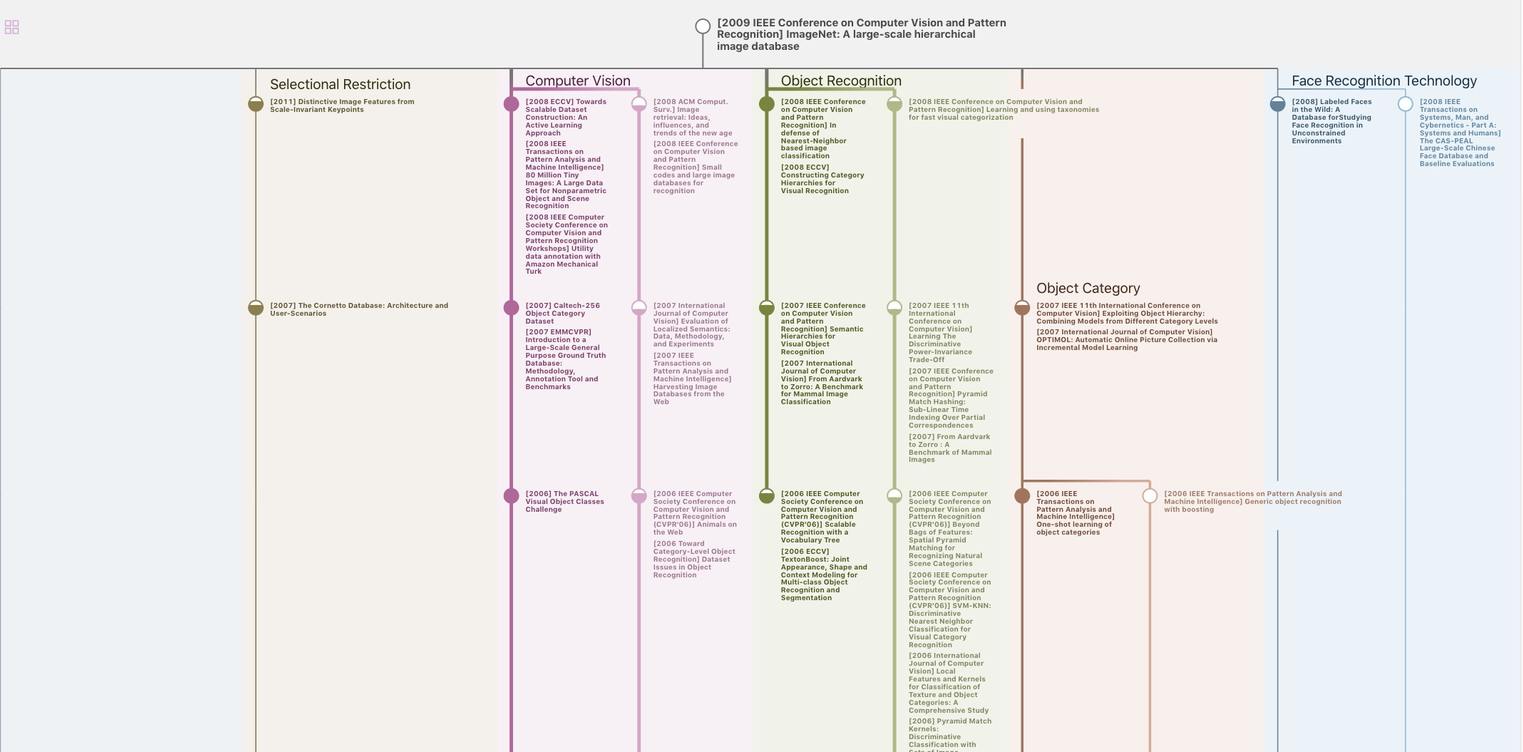
生成溯源树,研究论文发展脉络
Chat Paper
正在生成论文摘要