Feature selection based on probability and mathematical expectation
INTERNATIONAL JOURNAL OF MACHINE LEARNING AND CYBERNETICS(2024)
摘要
Many kinds of information entropy are employed for feature selection, but they lack corresponding probabilities to interpret; Despite many statistical indicators utilized in feature selection, neither probability nor mathematical expectation was applied to perform feature selection directly. To address such two problems, this article redefines three kinds of probabilities and their corresponding mathematical expectations from the perspective of granular computing and investigates their properties. These novel probabilities and mathematical expectations extend the meanings of classical probability and mathematical expectation and provide statistical interpretation for their corresponding information entropy, and then, attribute reducts based on probabilities and mathematical expectations are defined, which are proved to be equivalent to those based on their corresponding information entropy. A framework of feature selection algorithms based on probabilities and mathematical expectations (ARME) is designed after the presentation of their properties. Moreover, a novel definition form for feature selection is proposed, and another feature selection algorithm based on the mathematical expectation of conditional probability (ARMEC) is designed to reduce negative features on classification. Theoretical analysis and experimental results show that probabilities and mathematical expectations have super efficiency than their corresponding information entropy when they are considered as criteria of feature selection. Therefore, the novel method has the advantage over many state-of-the-art algorithms.
更多查看译文
关键词
Granular computing,Rough sets,Feature selection,Probability,Mathematical expectation
AI 理解论文
溯源树
样例
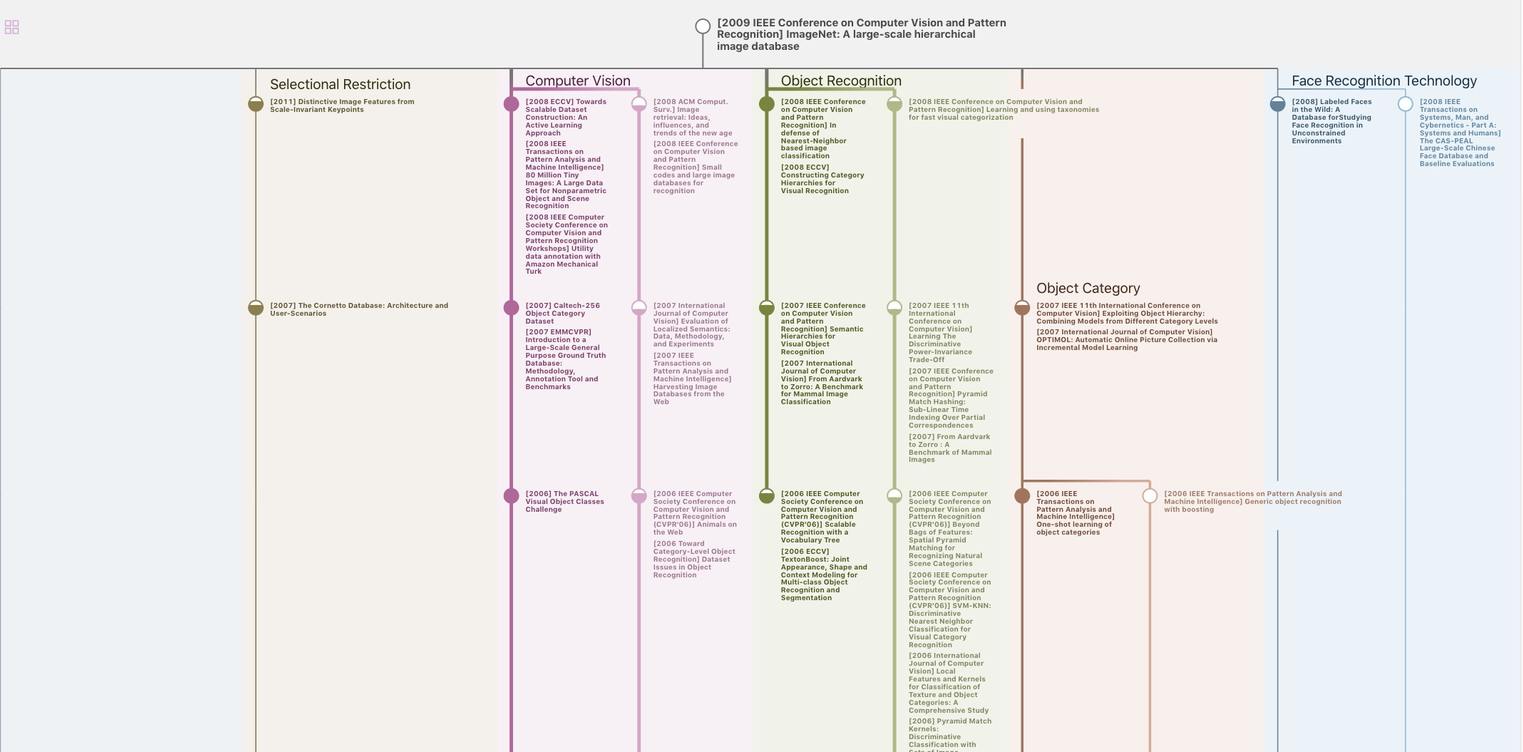
生成溯源树,研究论文发展脉络
Chat Paper
正在生成论文摘要