Aggregating Deep Features of Multi-CNN Models for Image Retrieval
NEURAL PROCESSING LETTERS(2023)
摘要
Convolutional neural network (CNN) models have certain advantages in various applications, including image retrieval and object recognition. However, aggregating deep features of multi-CNN models into a compact yet robust representation is challenging because background noise has a negative effect when encoding images into a robust descriptor, and can reduce the discriminative ability. To address this issue, we propose a novel image retrieval method: multi-models deep feature aggregation (MDFA), to represent image content, and utilize it for image retrieval. Its main highlights are as follows: (1) the deep features extracted from the two pre-trained CNN models are aggregated into a robust vector. This can leverage the complementary properties between the CNN models, which will help understand the image content and identify the target object. (2) An average mask (AVG-mask) is proposed to filter the feature maps of the pool5 layer in two pre-trained VGG models, which can effectively suppress the background noise and highlight the target object. (3) An effective aggregation method is proposed to aggregate deep features into a robust yet distinctive representation, which can improve the performance of image retrieval. Experimental results on five benchmark datasets demonstrated that the proposed method is better than some existing state-of-the-art methods in terms of the mAP metric, and it still performs well on large datasets. This method is versatile, simple, and efficient for describing image content.
更多查看译文
关键词
Image retrieval,Grey-level co-occurrence matrix,AVG-mask,Multi-models deep features aggregation
AI 理解论文
溯源树
样例
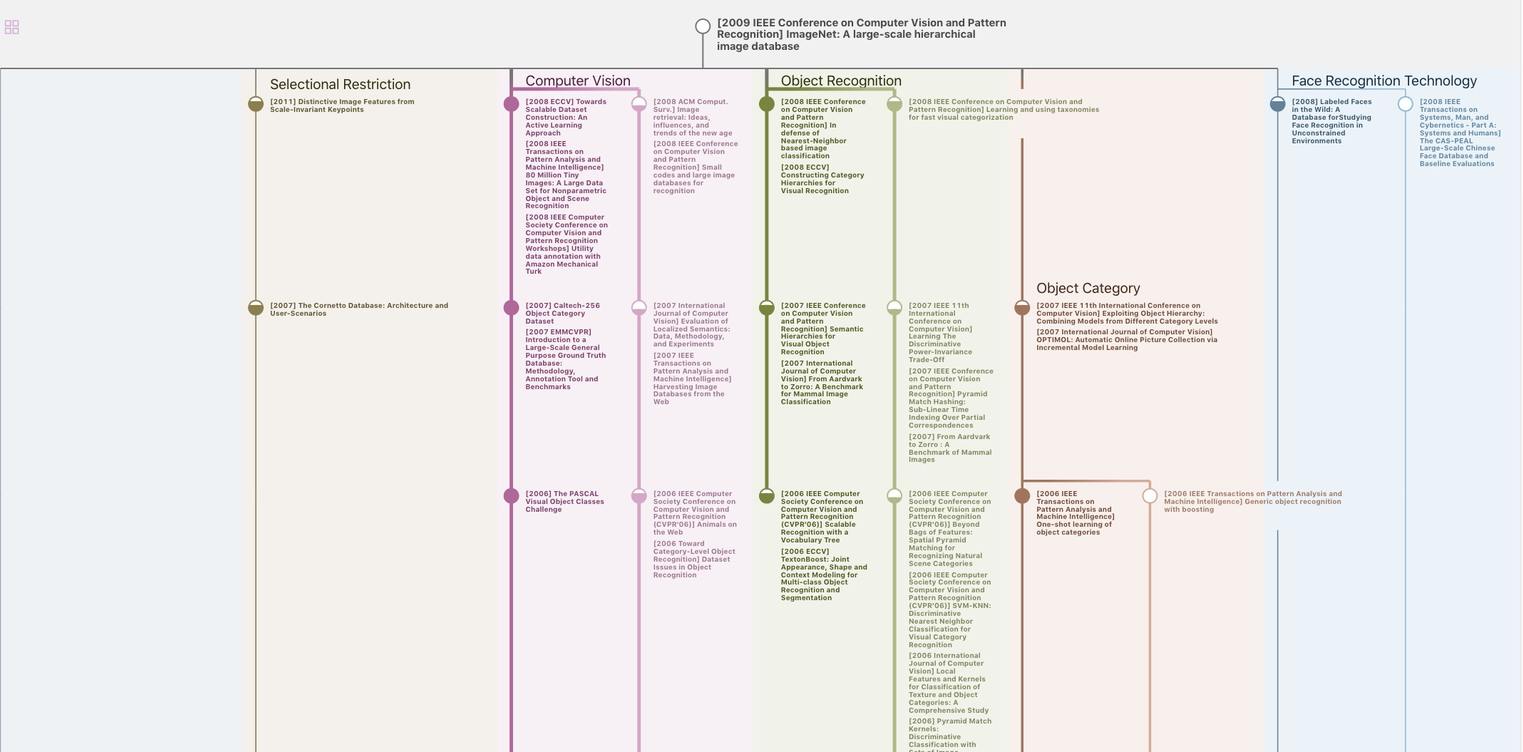
生成溯源树,研究论文发展脉络
Chat Paper
正在生成论文摘要