Can We Teach Functions to an Artificial Intelligence by Just Showing It Enough "Ground Truth"?
Lecture Notes in Mathematics(2023)
摘要
The term “artificial intelligence”, which in the past received very different interpretations, is nowadays being identified with deep learning. Deep neural networks bring the promise that, instead of hand-crafting data processing algorithms by mathematical reasoning based on formalized principles, we can simply feed enough data to a neural network, which will learn the right operator from it. In supervised learning, the data are either annotated by humans or obtained from a large set of observed pairs (xn, f(xn)). It is this association of an output f(xn) to an input xn in a learning dataset which is called a “ground truth”. The use of a “ground truth” annotated by humans raises a serious methodological problem, as humans are fallible. Worse even, the performance of these methods is evaluated and compared on subsets of the same annotations. Objective natural ground truths raise similar issues: raw data can be ambiguous or contradictory. In this paper, we shall examine two examples where machine learning methods were used to replicate aspects of human perception and logic: depth perception and the detection of straight lines or segments. We show that a strict control of the geometry in the learning data set, or a rigorous mathematical definition of the geometric task, lead to results widely different from those learned blindly from annotated datasets or from ground truths acquired in the wild. We conclude that a mathematical and principled analysis of learning datasets should precede their use. En gratitude à Catriona Byrne, mémorable, unique, et irremplaçable chef d’orchestre de l’édition mathématique.
更多查看译文
关键词
ground truth”,artificial intelligence,functions
AI 理解论文
溯源树
样例
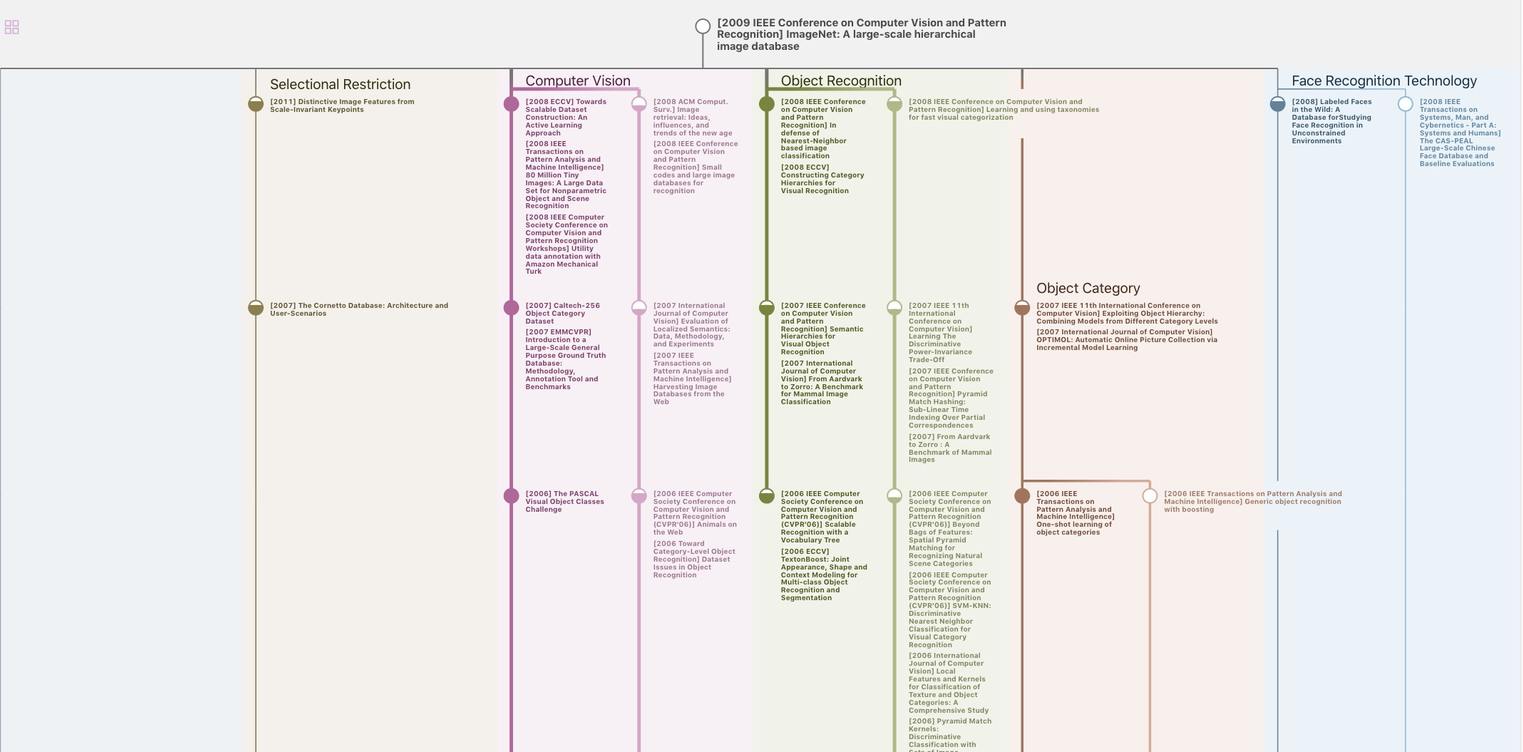
生成溯源树,研究论文发展脉络
Chat Paper
正在生成论文摘要