FocalFormer3D : Focusing on Hard Instance for 3D Object Detection
2023 IEEE/CVF International Conference on Computer Vision (ICCV)(2023)
Abstract
False negatives (FN) in 3D object detection, {\em e.g.}, missing predictions of pedestrians, vehicles, or other obstacles, can lead to potentially dangerous situations in autonomous driving. While being fatal, this issue is understudied in many current 3D detection methods. In this work, we propose Hard Instance Probing (HIP), a general pipeline that identifies \textit{FN} in a multi-stage manner and guides the models to focus on excavating difficult instances. For 3D object detection, we instantiate this method as FocalFormer3D, a simple yet effective detector that excels at excavating difficult objects and improving prediction recall. FocalFormer3D features a multi-stage query generation to discover hard objects and a box-level transformer decoder to efficiently distinguish objects from massive object candidates. Experimental results on the nuScenes and Waymo datasets validate the superior performance of FocalFormer3D. The advantage leads to strong performance on both detection and tracking, in both LiDAR and multi-modal settings. Notably, FocalFormer3D achieves a 70.5 mAP and 73.9 NDS on nuScenes detection benchmark, while the nuScenes tracking benchmark shows 72.1 AMOTA, both ranking 1st place on the nuScenes LiDAR leaderboard. Our code is available at \url{https://github.com/NVlabs/FocalFormer3D}.
MoreTranslated text
Key words
focalformer3d object detection,hard instance
AI Read Science
Must-Reading Tree
Example
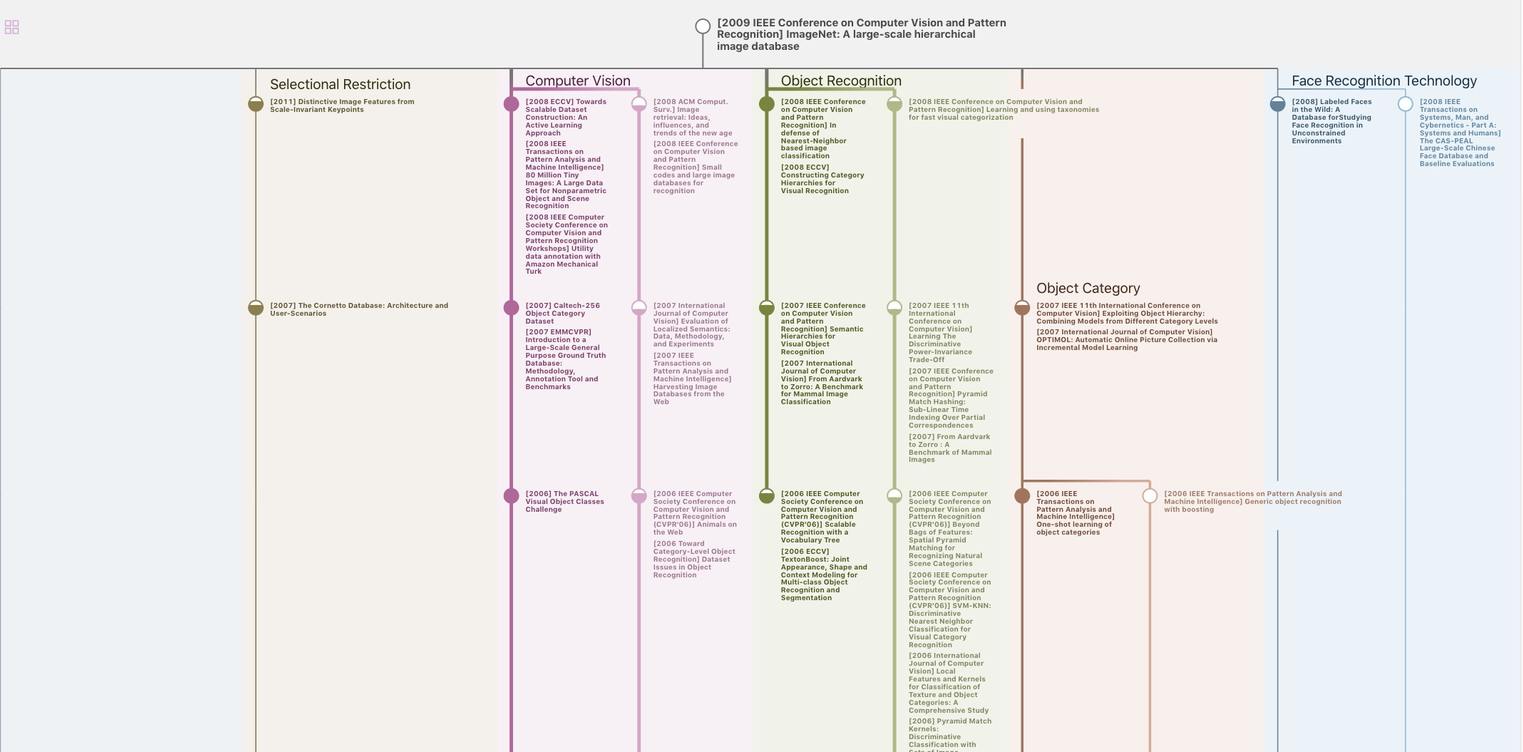
Generate MRT to find the research sequence of this paper
Chat Paper
Summary is being generated by the instructions you defined