Decorrelating neurons using persistence
CoRR(2023)
摘要
We propose a novel way to improve the generalisation capacity of deep learning models by reducing high correlations between neurons. For this, we present two regularisation terms computed from the weights of a minimum spanning tree of the clique whose vertices are the neurons of a given network (or a sample of those), where weights on edges are correlation dissimilarities. We provide an extensive set of experiments to validate the effectiveness of our terms, showing that they outperform popular ones. Also, we demonstrate that naive minimisation of all correlations between neurons obtains lower accuracies than our regularisation terms, suggesting that redundancies play a significant role in artificial neural networks, as evidenced by some studies in neuroscience for real networks. We include a proof of differentiability of our regularisers, thus developing the first effective topological persistence-based regularisation terms that consider the whole set of neurons and that can be applied to a feedforward architecture in any deep learning task such as classification, data generation, or regression.
更多查看译文
关键词
neurons,persistence
AI 理解论文
溯源树
样例
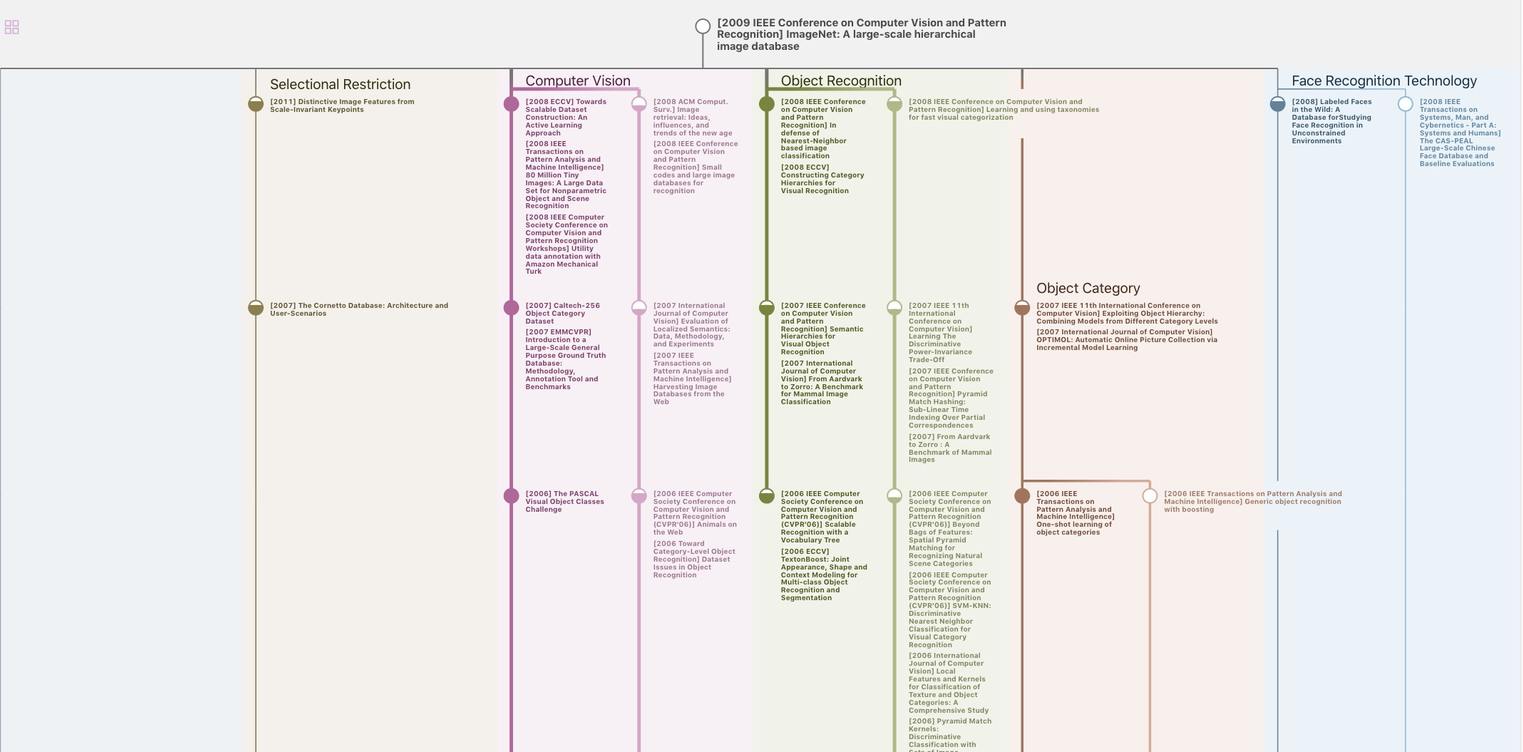
生成溯源树,研究论文发展脉络
Chat Paper
正在生成论文摘要