Learning consistent subcellular landmarks to quantify changes in multiplexed protein maps
NATURE METHODS(2023)
摘要
Highly multiplexed imaging holds enormous promise for understanding how spatial context shapes the activity of the genome and its products at multiple length scales. Here, we introduce a deep learning framework called CAMPA (Conditional Autoencoder for Multiplexed Pixel Analysis), which uses a conditional variational autoencoder to learn representations of molecular pixel profiles that are consistent across heterogeneous cell populations and experimental perturbations. Clustering these pixel-level representations identifies consistent subcellular landmarks, which can be quantitatively compared in terms of their size, shape, molecular composition and relative spatial organization. Using high-resolution multiplexed immunofluorescence, this reveals how subcellular organization changes upon perturbation of RNA synthesis, RNA processing or cell size, and uncovers links between the molecular composition of membraneless organelles and cell-to-cell variability in bulk RNA synthesis rates. By capturing interpretable cellular phenotypes, we anticipate that CAMPA will greatly accelerate the systematic mapping of multiscale atlases of biological organization to identify the rules by which context shapes physiology and disease.
更多查看译文
关键词
consistent subcellular landmarks,protein,learning,maps
AI 理解论文
溯源树
样例
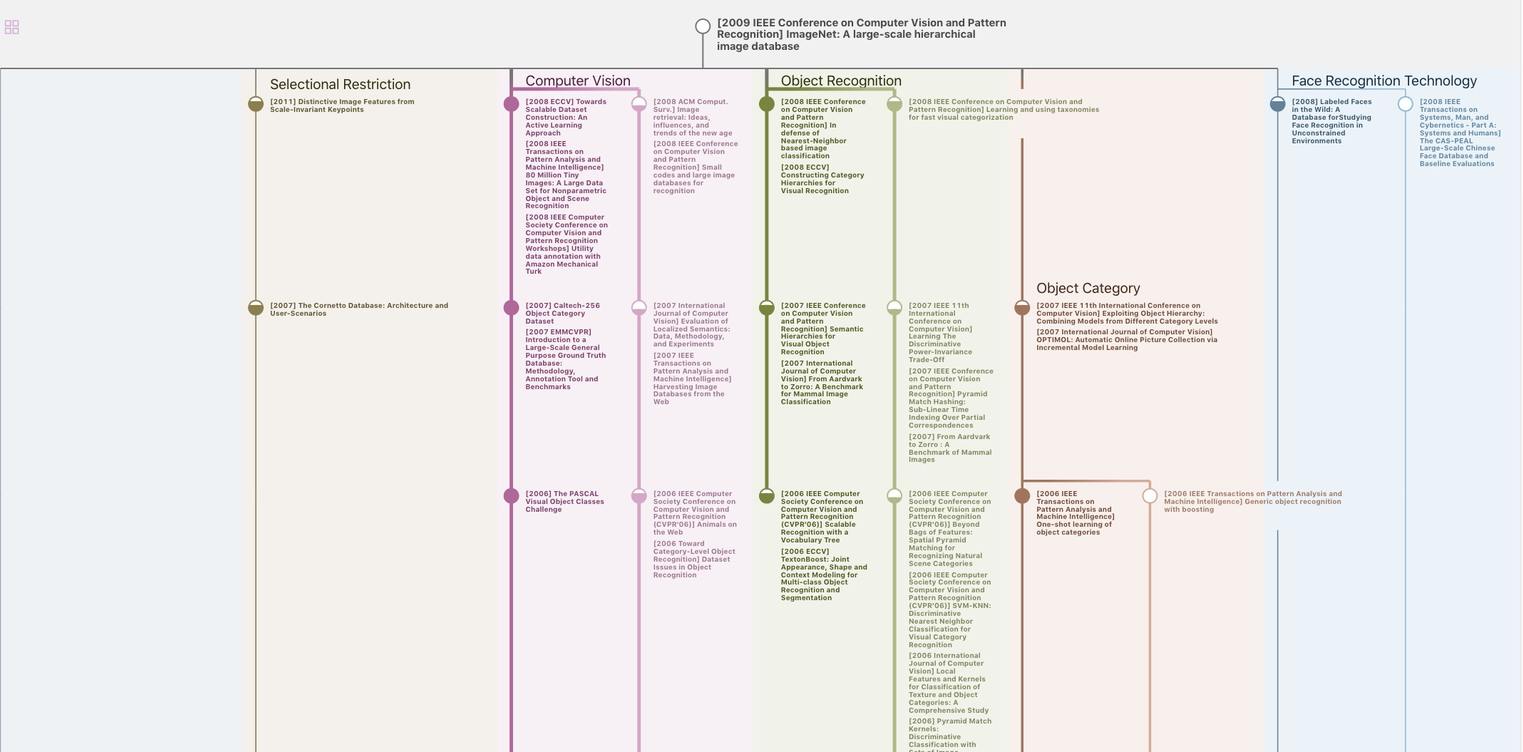
生成溯源树,研究论文发展脉络
Chat Paper
正在生成论文摘要