Quantitative interpretation of geoelectric inverted data with a robust probabilistic approach
GEOPHYSICS(2023)
摘要
The nonuniqueness of the solution to the geophysical inverse problem can lead to misinterpretation while characterizing the subsurface. To tackle this situation, ground-truth information from excavations and wells can be used to improve, calibrate, and interpret inverted models. We refer to quantitative interpre-tation as the decision analysis based on probability theory, which is focused on solving a classification problem. First, we present a probabilistic approach to classify the different types of materials or "categories" observed in borehole logs using multiple data sources: inverted 2D electrical resistivity tomography and in-duced polarization data and the positions (x, z) of these boreholes. Then, using the Bayes' rule and permanence of ratios, we com-pute the joint conditional probabilities of each category, given all data sources in the whole inverted model domain. We validate this approach with synthetic data modeling for a complex anthropogenic-geologic scenario and using real data from an old landfill. Afterward, we assess the performance of the probabilistic approach for classification and compare it with the machine learn-ing algorithm of multilayer perceptron (MLP). In addition, we analyze the effect that the different data sources and the number of boreholes (and their distribution) have on both approaches with the synthetic case. Our results indicate that the MLP performance is better for delineating the different categories where the lateral contrasts in the synthetic resistivity model are small. Never-theless, the classification obtained with the probabilistic approach using real data seems to provide a more geologically realistic distribution. We conclude that the probabilistic approach is robust for classifying categories when high spatial heterogeneity is ex-pected and when ground-truth data are limited or not sparsely distributed. Finally, this approach can be easily extended to inte-grate multiple geophysical methods and does not require the optimization of hyperparameters as for MLP.
更多查看译文
关键词
geoelectric
AI 理解论文
溯源树
样例
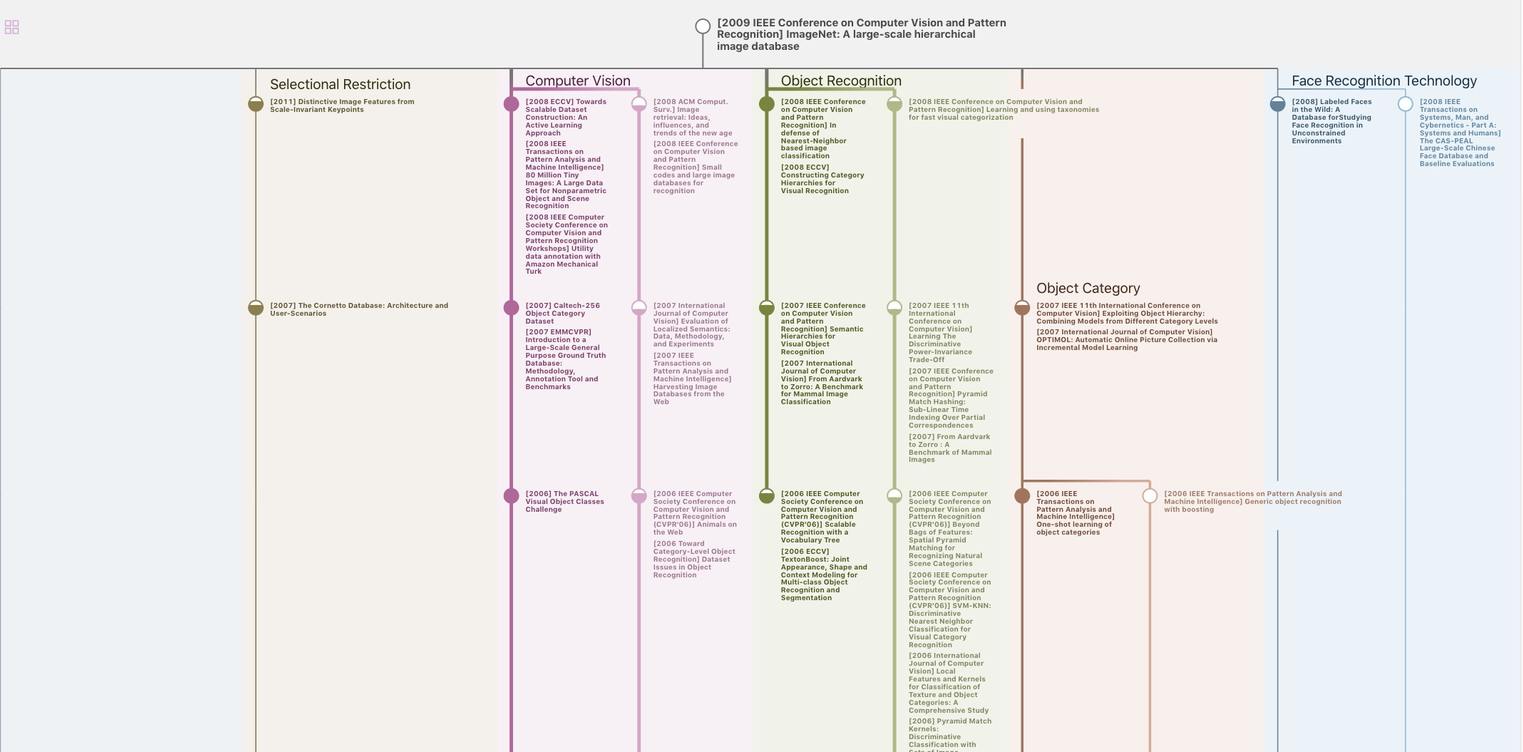
生成溯源树,研究论文发展脉络
Chat Paper
正在生成论文摘要