Iterative Training Attack: A Black-Box Adversarial Attack Via Perturbation Generative Network.
Journal of circuits, systems, and computers(2023)
摘要
Deep neural networks are vulnerable to adversarial examples. While there are many methods for generating adversarial examples using neural networks, creating such examples with high perceptual quality and improved training remains an area of active research. In this paper, we propose the Iterative Training Attack (ITA), a black-box attack based on a perturbation generative network for generating adversarial examples. ITA generates such examples by randomly initializing the perturbation generative network multiple times, iteratively training and optimizing a refined loss function. Compared to other neural network-based attacks, our proposed method generates adversarial examples with higher attack rates and within a small perturbation range even when the advanced defense is employed. Despite being a black-box attack, ITA outperforms gradient-based white-box attacks even under basic standards. The authors evaluated their method on a TRADES robust model trained with the MNIST dataset and achieved a robust accuracy of 92.46%, the highest among the evaluated methods.
更多查看译文
关键词
Adversarial attack,neural network security,adversarial examples
AI 理解论文
溯源树
样例
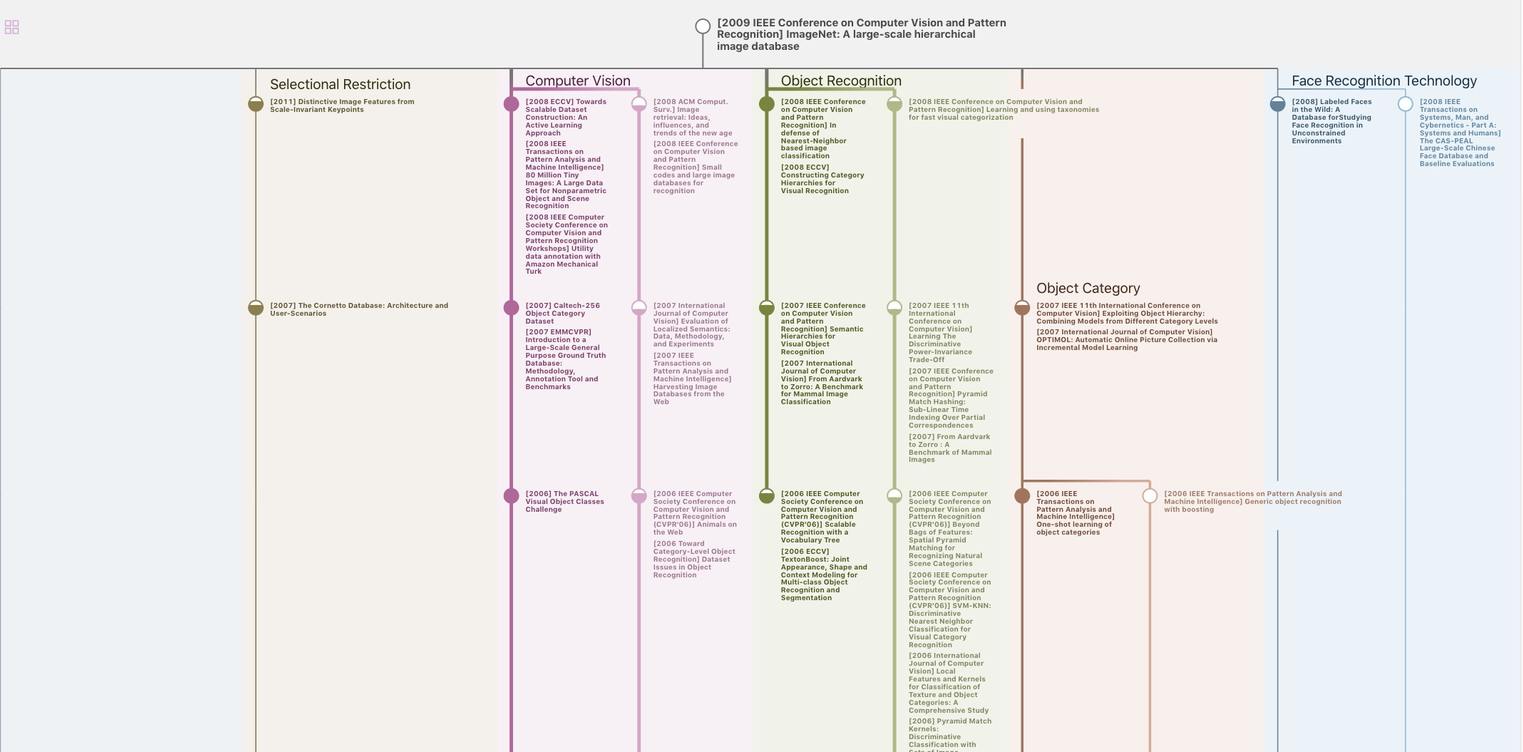
生成溯源树,研究论文发展脉络
Chat Paper
正在生成论文摘要