Research on Rolling Bearing Fault Diagnosis Based on DRS Frequency Spectrum Image and Deep Learning
INTERNATIONAL JOURNAL OF ACOUSTICS AND VIBRATION(2023)
摘要
Deep learning is gradually being widely used in fault diagnosis now, because deep learning networks are more advantageous in processing data, especially image data. However, research using frequency spectra image of fault signals as inputs to deep learning networks are extremely rare in the field of fault diagnosis. Therefore, a brandnew intelligent fault diagnosis method is proposed in this paper which combines discrete random separate (DRS) frequency spectrum images with deep learning networks (DRSFSI-DL). To investigate the fault diagnosis effects of the method mentioned above, several deep learning networks are utilized for comparisons, such as GoogLeNet, residual network, and Inception ResNet v2. The vibration fault frequency spectrum images processed by the DRS method are input to train several deep learning networks. Under the same circumstance of deep learning networks, the fault diagnosis using the DRS frequency spectrum image (DRSFSI), is also compared to the fault diagnosis using traditional frequency spectrum, including the power spectrum density (PSD) and cepstrum. The fault diagnosis results show that the proposed method has a better classification accuracy than the PSD image and cepstrum image, with the same deep learning networks. The fault diagnosis accuracy can reach up to 100.00% for some deep learning networks with better generalization performance than the PSD image and cepstrum image. Lastly, the proposed method is further verified using the brand-new bearing fault dataset, and excellent accuracy and generalization ability are achieved.
更多查看译文
关键词
Fault Diagnosis,Deep Learning,Bearing Faults,Vibration Analysis
AI 理解论文
溯源树
样例
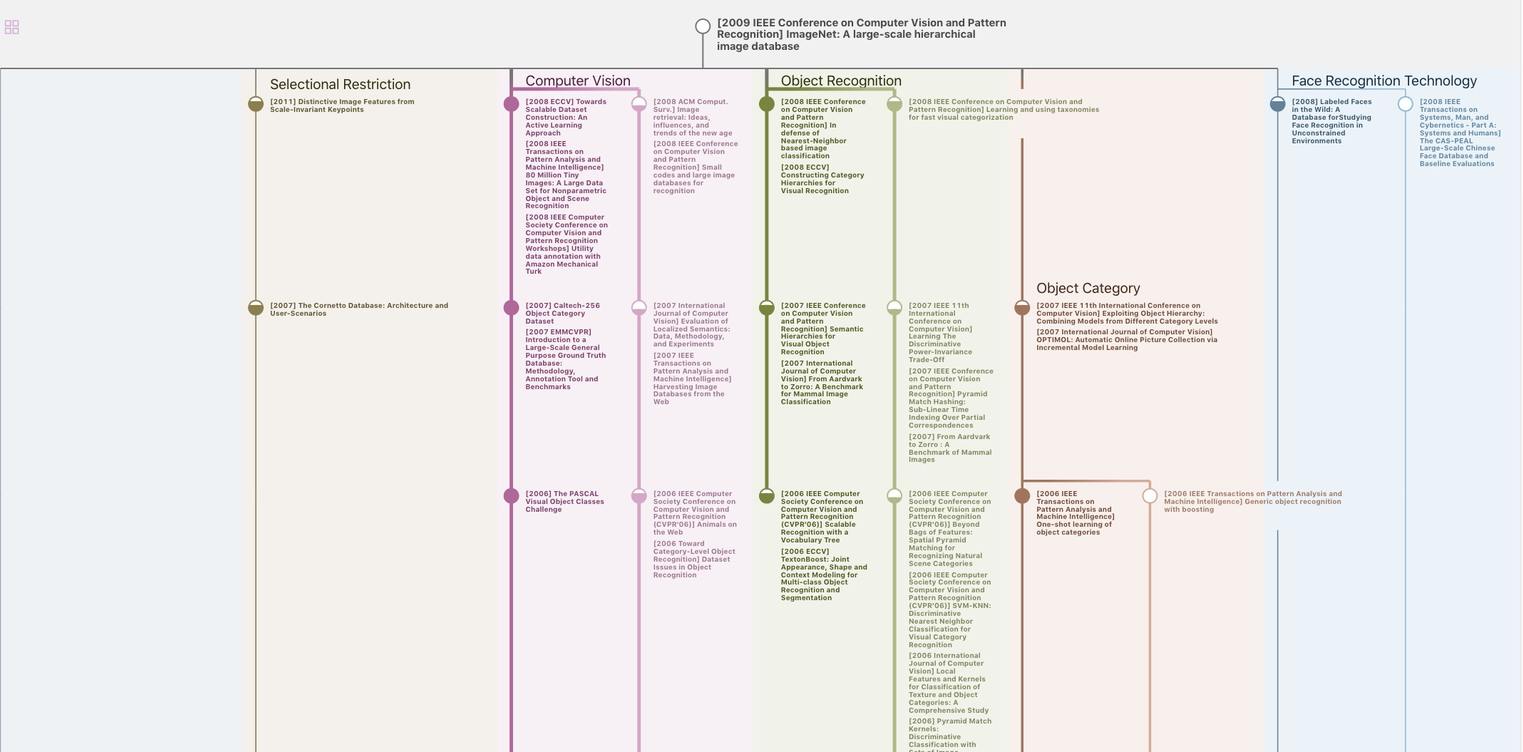
生成溯源树,研究论文发展脉络
Chat Paper
正在生成论文摘要