Adaptive learning Unet-based adversarial network with CNN and transformer for segmentation of hard exudates in diabetes retinopathy
IET IMAGE PROCESSING(2023)
摘要
Accurate segmentation of hard exudates in early non-proliferative diabetic retinopathy can assist physicians in taking appropriate treatment in a more targeted manner, in order to avoid more serious damage to vision caused by the deterioration of the disease in the later stages. Here, an Adaptive Learning Unet-based adversarial network with Convolutional neural network and Transformer (CT-ALUnet) is proposed for automatic segmentation of hard exudates, combining the excellent local modelling ability of Unet with the global attention mechanism of transformer. Firstly, multi-scale features are extracted through a CNN dual-branch encoder. Then, the information fusion of features at adjacent scale is realized and the fused features are selected adaptively to maintain the overall consistency of features by attention-guided multi-scale fusion blocks (AGMFB). After that, the high-level encoded features are input to transformer blocks to extract global contexts. Finally, these features are fused layer-by-layer to achieve accurate segmentation of hard exudates. In addition, adversarial training is incorporated into the above segmentation model, which improves Dice scores and MIoU scores by 7.5% and 3%, respectively. Experiments demonstrate that CT-ALUnet shows more reliable segmentation and stronger generalization ability than other SOTA methods, which lays a good foundation for computer-assisted diagnosis and assessment of efficacy.
更多查看译文
关键词
adversarial network,diabetes retinopathy,cnn,segmentation
AI 理解论文
溯源树
样例
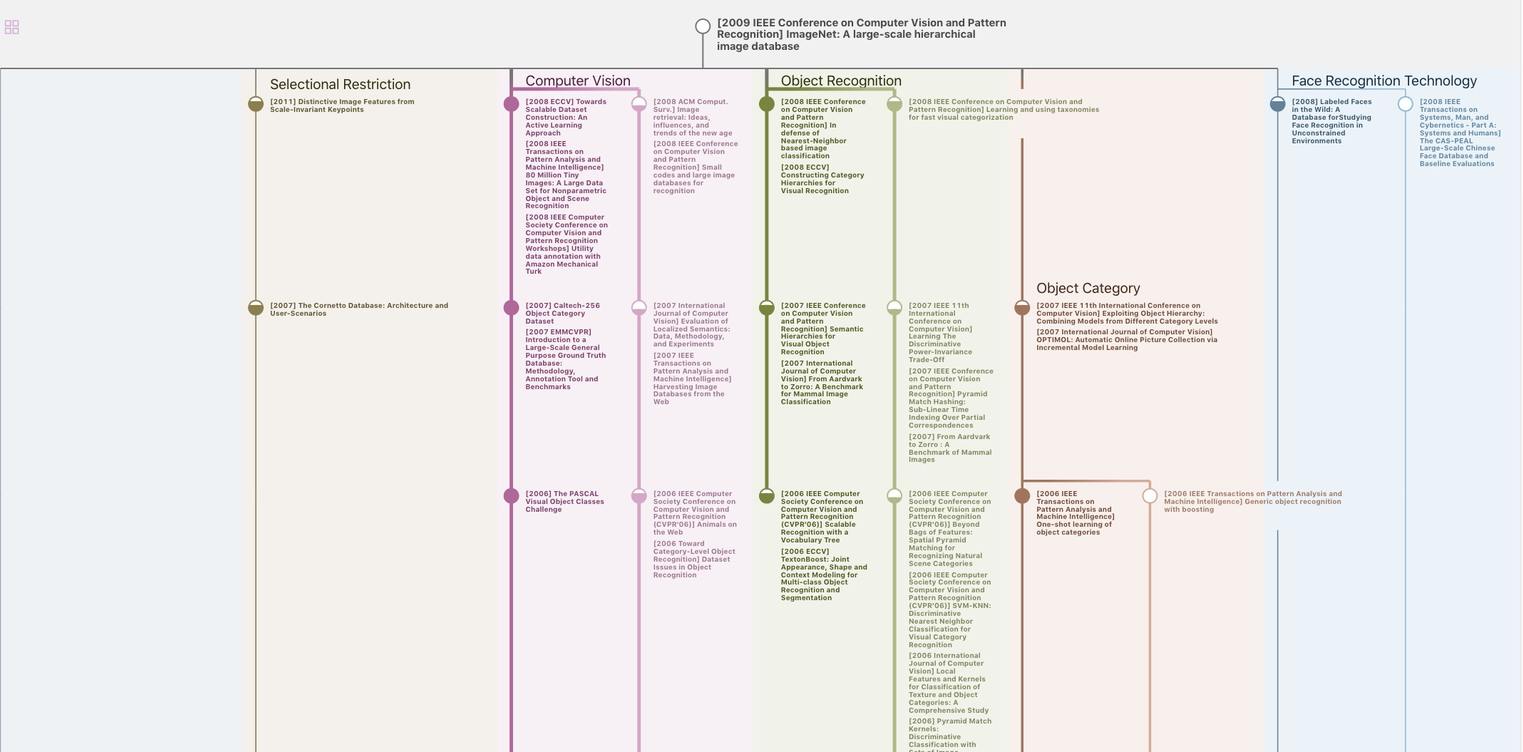
生成溯源树,研究论文发展脉络
Chat Paper
正在生成论文摘要