Application of multi-criteria decision analysis techniques and decision support framework for informing select agent designation for agricultural animal pathogens
JOURNAL OF CIRCUITS SYSTEMS AND COMPUTERS(2023)
摘要
Accurate river level prediction is vital in water resource management and flood mitigation. Recent advances in data-driven modeling, especially in deep learning, provide profound insights into predicting river levels when mechanistic hydrological knowledge is absent. However, they often do not capture predictive uncertainty well, making them less robust in the hydrological prediction as they overconfidently extrapolate. Moreover, they are not flexible in handling hydrological variables with heterogeneous characteristics. In this work, we present the Temporal Fusion Transformer-Gaussian Process (TFT-GP), a novel model for multi-horizon probabilistic river level prediction. We show how TFT-GP inherits the nice properties of the Gaussian process and deep neural networks, giving it excellent representative power and uncertainty quantification ability. The performance of TFT-GP is thoroughly compared with existing well-known deep learning models in three real-world hydrological datasets, and the results showed that TFT-GP is not only more accurate in point prediction but also more reasonable in uncertainty quantification.
更多查看译文
关键词
River level prediction, uncertainty quantification, scalable variational Gaussian process, temporal fusion transformer
AI 理解论文
溯源树
样例
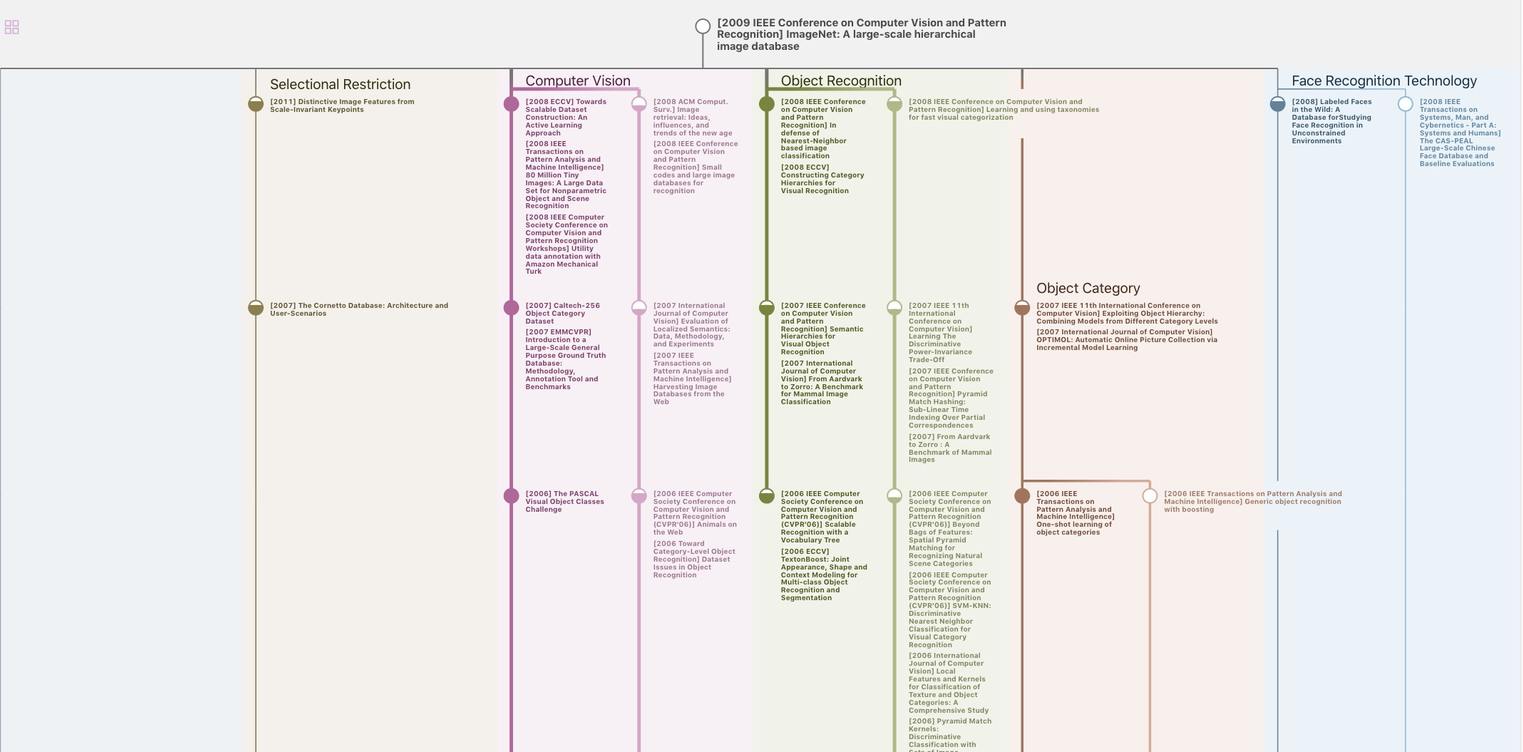
生成溯源树,研究论文发展脉络
Chat Paper
正在生成论文摘要