Probabilistic Prediction of Remaining Useful Life of Lithium-ion Batteries
2022 4TH INTERNATIONAL CONFERENCE ON SMART POWER & INTERNET ENERGY SYSTEMS, SPIES(2022)
摘要
To alleviate the concern about the safety and reliability of lithium-ion batteries in electric vehicles, the prediction of remaining useful life (RUL) is attracting growing attention. General deterministic approaches focus more on estimating the expected values of RUL, while the inherent uncertainty in RUL has not been fully addressed. In this paper, two probabilistic prediction methods, linear quantile regression (LQR) and quantile regression random forest (QRRF), are proposed to address the above issues. Using a publicly available dataset from MIT, the performance of the proposed methods is validated, and the uncertainty of RUL is discussed. The results show that both methods achieve good performance in the probabilistic prediction while maintaining acceptable deterministic accuracy. However, due to the notable variations in the signal-to-noise ratio in the battery data at different aging cycles, LQR and QRRF exhibit their better prediction performance in the early and late stages of battery life, respectively.
更多查看译文
关键词
Lithium-ion battery,Remaining useful life,Probabilistic prediction,quantile regression
AI 理解论文
溯源树
样例
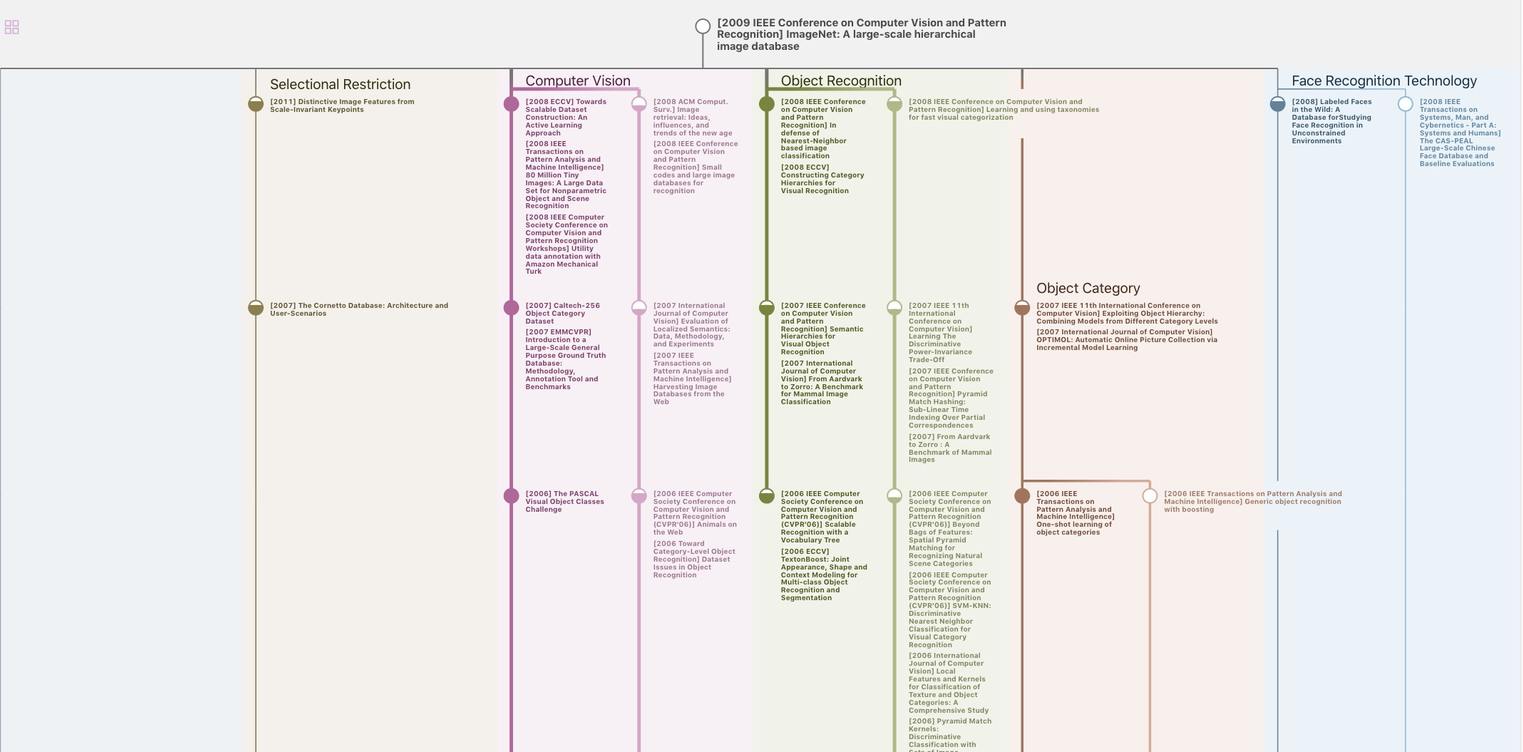
生成溯源树,研究论文发展脉络
Chat Paper
正在生成论文摘要