HEU-Net: hybrid attention residual block-based network with external skip connections for metal corrosion semantic segmentation
VISUAL COMPUTER(2024)
摘要
Regularly detect the corrosion of metal structures and take countermeasures according to the degree of corrosion, which can reduce the potential safety hazards and avoid unnecessary economic losses. This paper presents a deep learning-based metal corrosion detection method, which is used to accurately segment the corrosion regions. This model incorporates Hybrid Attention Residual Block (HARB) and External Skip Connections (ESC) in the basic architecture of U-Net. HARB can reweight the features so that the network pays more attention to the corrosion regions and ignores other irrelevant regions. Shallow features and deep features are fused using ESC to make the features of network learning more effective and comprehensive, which is conducive to improving the segmentation accuracy. In order to further improve the semantic segmentation performance, the Attention Gate Residual Block (AGRB) is proposed, which can optimize segmentation by strengthening the regions to be segmented and reducing the activation value of the background, while overcoming the semantic and scale inconsistencies between input features. In this paper, comprehensive comparative experiments are conducted on the Metal Corrosion dataset. The experimental results show that the F1 and mIoU of the proposed method are 91.21% and 89.56%, respectively. Compared with U-Net, HEU-Net achieves a 7.91% improvement in F1 and a 10.16% improvement in mIoU. These results show that our method outperforms state-of-the-art models and can achieve better semantic segmentation results.
更多查看译文
关键词
Corrosion detection,Semantic segmentation,Attention,Residual,Skip connections
AI 理解论文
溯源树
样例
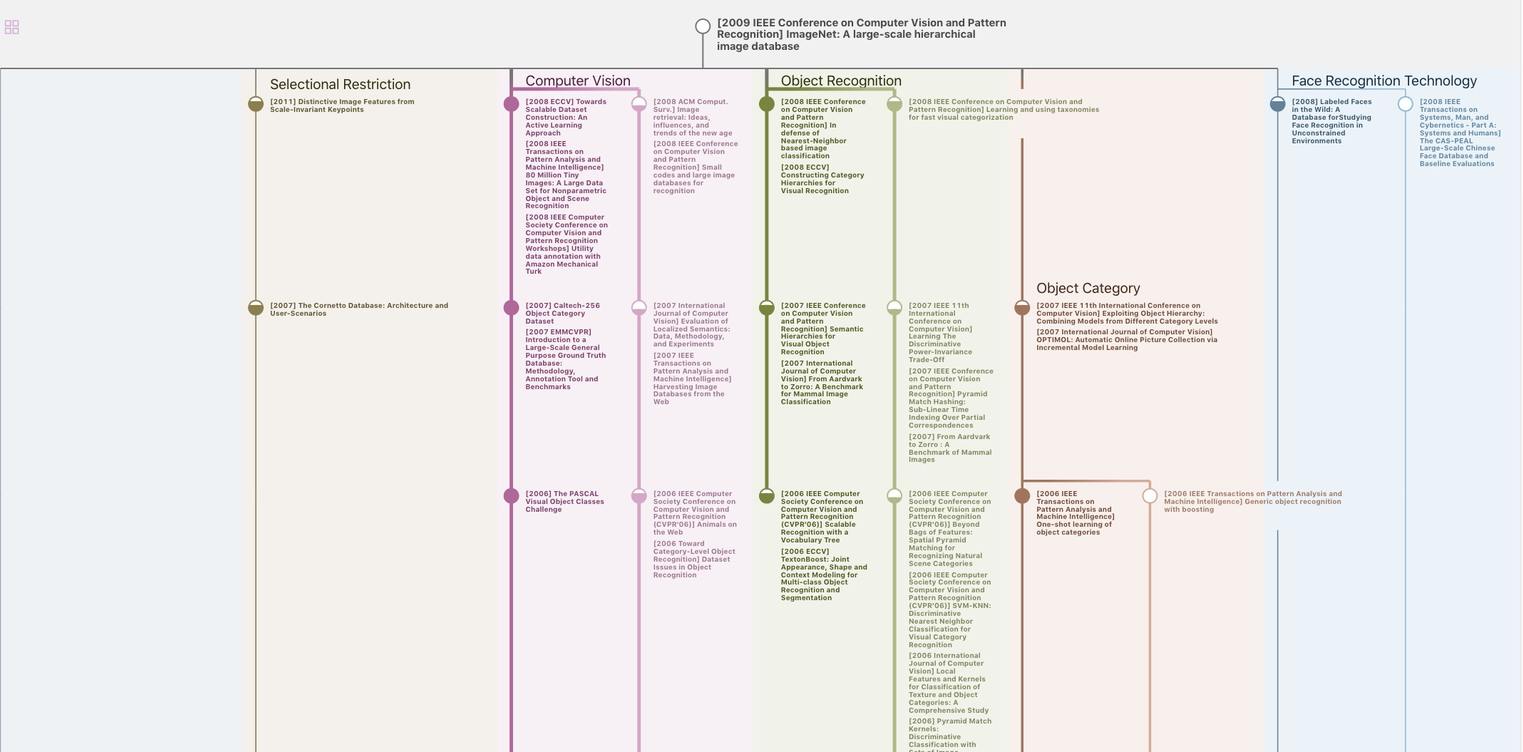
生成溯源树,研究论文发展脉络
Chat Paper
正在生成论文摘要