A transformer-augmented deep learning algorithm, cystonet-t, for improved cystoscopic bladder cancer detection
JOURNAL OF UROLOGY(2023)
摘要
You have accessJournal of UrologyCME1 Apr 2023PD01-09 A TRANSFORMER-AUGMENTED DEEP LEARNING ALGORITHM, CYSTONET-T, FOR IMPROVED CYSTOSCOPIC BLADDER CANCER DETECTION Eugene Shkolyar, Xiao Jia, Mark Laurie, Okyaz Eminaga, Lei Xing, and Joseph Liao Eugene ShkolyarEugene Shkolyar More articles by this author , Xiao JiaXiao Jia More articles by this author , Mark LaurieMark Laurie More articles by this author , Okyaz EminagaOkyaz Eminaga More articles by this author , Lei XingLei Xing More articles by this author , and Joseph LiaoJoseph Liao More articles by this author View All Author Informationhttps://doi.org/10.1097/JU.0000000000003218.09AboutPDF ToolsAdd to favoritesDownload CitationsTrack CitationsPermissionsReprints ShareFacebookLinked InTwitterEmail Abstract INTRODUCTION AND OBJECTIVE: Adequate tumor detection is critical in cystoscopy for bladder cancer (BCa) diagnosis, risk stratification and treatment. The adoption of adjunct imaging technologies remains modest. Previously, we developed a deep learning algorithm, CystoNet, that holds the potential to improve performance of white-light cystoscopy (WLC) in a non-invasive and cost-effective manner. Herein we report CystoNet-T which incorporates a transformer-augmented deep learning algorithm to further improve detection of BCa on WLC. METHODS: CystoNet-T was developed with a transformer-augmented pyramidal convolutional neural network architecture to improve automated BCa detection during WLC. A training set consisting of 510 tumor-containing frames (54 patients) and a test set of 101 tumor-containing frames (13 patients) were used. Training and test datasets were annotated by a urologist and pathologically confirmed to be BCa. Recall and precision were determined using intersection-over-union as the metric of interest. Harmonic precision-recall mean (F1 score) and average precision (AP) were determined. Performance was then compared against other benchmarks algorithms using the same training and test sets. RESULTS: CystoNet-T detected BCa with a recall of 97.3%, precision of 95.6%, F1 of 96.4% and AP of 91.4% (Figure 1), outperforming the original CystoNet and benchmark models of Faster R-CNN and YOLO by 7.3% in F1 and 3.8% points in AP. CONCLUSIONS: We have developed a deep-learning algorithm that improves on prior models in accurately detecting bladder tumors on WLC. Transformer-augmented AI platforms may aid in diagnostic decision making by improving diagnostic yield and target identification. Source of Funding: NIH R01 CA260426, Urology Care Foundation Research Scholar Award © 2023 by American Urological Association Education and Research, Inc.FiguresReferencesRelatedDetails Volume 209Issue Supplement 4April 2023Page: e66 Advertisement Copyright & Permissions© 2023 by American Urological Association Education and Research, Inc.MetricsAuthor Information Eugene Shkolyar More articles by this author Xiao Jia More articles by this author Mark Laurie More articles by this author Okyaz Eminaga More articles by this author Lei Xing More articles by this author Joseph Liao More articles by this author Expand All Advertisement PDF downloadLoading ...
更多查看译文
关键词
transformer-augmented
AI 理解论文
溯源树
样例
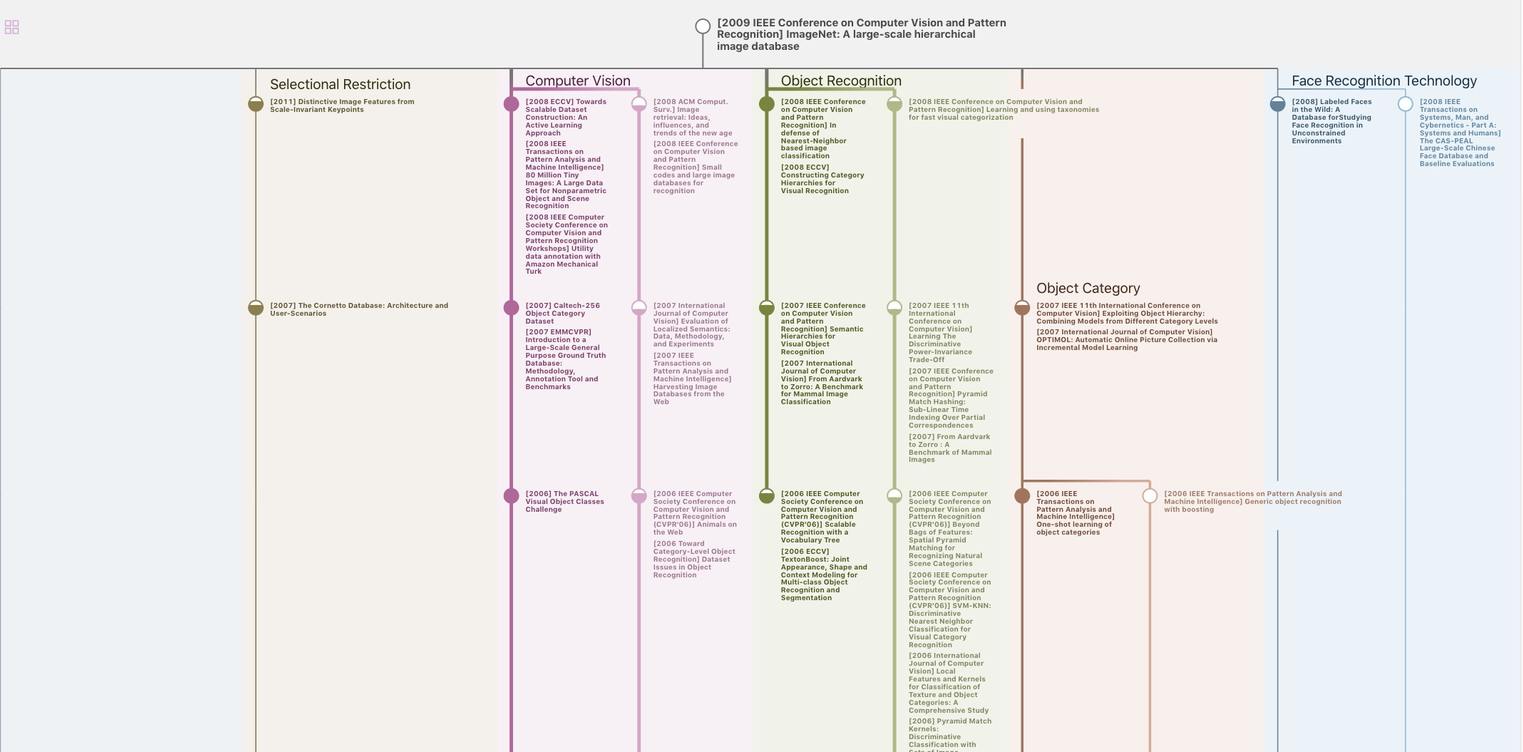
生成溯源树,研究论文发展脉络
Chat Paper
正在生成论文摘要