MP18-20 AUTOMATED URINARY TRACT DILATION GRADING OF HYDRONEPHROSIS ON ULTRASOUND IMAGING USING A CONVOLUTIONAL NEURAL NETWORK
JOURNAL OF UROLOGY(2023)
摘要
You have accessJournal of UrologyCME1 Apr 2023MP18-20 AUTOMATED URINARY TRACT DILATION GRADING OF HYDRONEPHROSIS ON ULTRASOUND IMAGING USING A CONVOLUTIONAL NEURAL NETWORK David Ostrowski, Joseph Logan, Maria Antony, Reilly Broms, Dana Weiss, Jason van Batavia, Christopher Long, Ariana Smith, Stephen Zderic, Rebecca Edwins, Raymond Pominville, Jessica Hannick, Lynn Woo, Yong Fan, Gregory Tasian, and John Weaver David OstrowskiDavid Ostrowski More articles by this author , Joseph LoganJoseph Logan More articles by this author , Maria AntonyMaria Antony More articles by this author , Reilly BromsReilly Broms More articles by this author , Dana WeissDana Weiss More articles by this author , Jason van BataviaJason van Batavia More articles by this author , Christopher LongChristopher Long More articles by this author , Ariana SmithAriana Smith More articles by this author , Stephen ZdericStephen Zderic More articles by this author , Rebecca EdwinsRebecca Edwins More articles by this author , Raymond PominvilleRaymond Pominville More articles by this author , Jessica HannickJessica Hannick More articles by this author , Lynn WooLynn Woo More articles by this author , Yong FanYong Fan More articles by this author , Gregory TasianGregory Tasian More articles by this author , and John WeaverJohn Weaver More articles by this author View All Author Informationhttps://doi.org/10.1097/JU.0000000000003238.20AboutPDF ToolsAdd to favoritesDownload CitationsTrack CitationsPermissionsReprints ShareFacebookLinked InTwitterEmail Abstract INTRODUCTION AND OBJECTIVE: The grading of antenatal hydronephrosis (ANH) on postnatal renal ultrasounds dictates management of patients with ANH. Multiple grading systems attempt to standardize hydronephrosis grading, yet poor inter-observer reliability persists. Machine learning methods may provide tools to improve accuracy and efficiency of hydronephrosis grading. We sought to develop an automated convolutional neural network (CNN) model to classify renal ultrasounds according to the Urinary Tract Dilation (UTD) system as an adjunct for hydronephrosis grading. METHODS: A retrospective, single-institution cohort of postnatal renal ultrasounds from patients with and without hydronephrosis with radiologist UTD grading was obtained. An algorithm used image labels to select sagittal renal images from each study. These preprocessed images were analyzed by a VGG16 pre-trained ImageNet CNN model. 3K-fold stratified cross-validation was used to predict on the dataset. The model classified images into five UTD classes (normal, mild hydronephrosis not meeting criteria for P1, P1, P2, or P3). These predictions were compared to radiologist grading. Confusion matrices evaluated model performance. Gradient class activation mapping demonstrated image features driving model predictions. RESULTS: We identified 610 patients with 2,828 renal ultrasound series. Per radiologist grading, 847 were normal, 601 were mild hydronephrosis, 336 were P1, 662 were P2, and 442 were P3. The model predicted hydronephrosis grade with 79% overall accuracy and classified 97% of the studies correctly or within one grade of the radiologist grade. The model classified 94% of normal studies, 74% of mild hydronephrosis studies, 68% of P1 ultrasounds, 74% of P2 ultrasounds, and 90% of P3 ultrasounds accurately. Gradient class activation mapping demonstrated the renal collecting system drove the model’s predictions. CONCLUSIONS: An automated CNN model classified hydronephrosis on renal ultrasounds by the UTD system with promising accuracy compared to prior studies. The model graded images based on the UTD system using appropriate imaging features. These findings suggest a possible adjunctive role for machine learning systems in the grading of ANH. Source of Funding: Pennsylvania Department of Health Tobacco Grant Funding Agency Number SAP #4100085749 © 2023 by American Urological Association Education and Research, Inc.FiguresReferencesRelatedDetails Volume 209Issue Supplement 4April 2023Page: e231 Advertisement Copyright & Permissions© 2023 by American Urological Association Education and Research, Inc.MetricsAuthor Information David Ostrowski More articles by this author Joseph Logan More articles by this author Maria Antony More articles by this author Reilly Broms More articles by this author Dana Weiss More articles by this author Jason van Batavia More articles by this author Christopher Long More articles by this author Ariana Smith More articles by this author Stephen Zderic More articles by this author Rebecca Edwins More articles by this author Raymond Pominville More articles by this author Jessica Hannick More articles by this author Lynn Woo More articles by this author Yong Fan More articles by this author Gregory Tasian More articles by this author John Weaver More articles by this author Expand All Advertisement PDF downloadLoading ...
更多查看译文
AI 理解论文
溯源树
样例
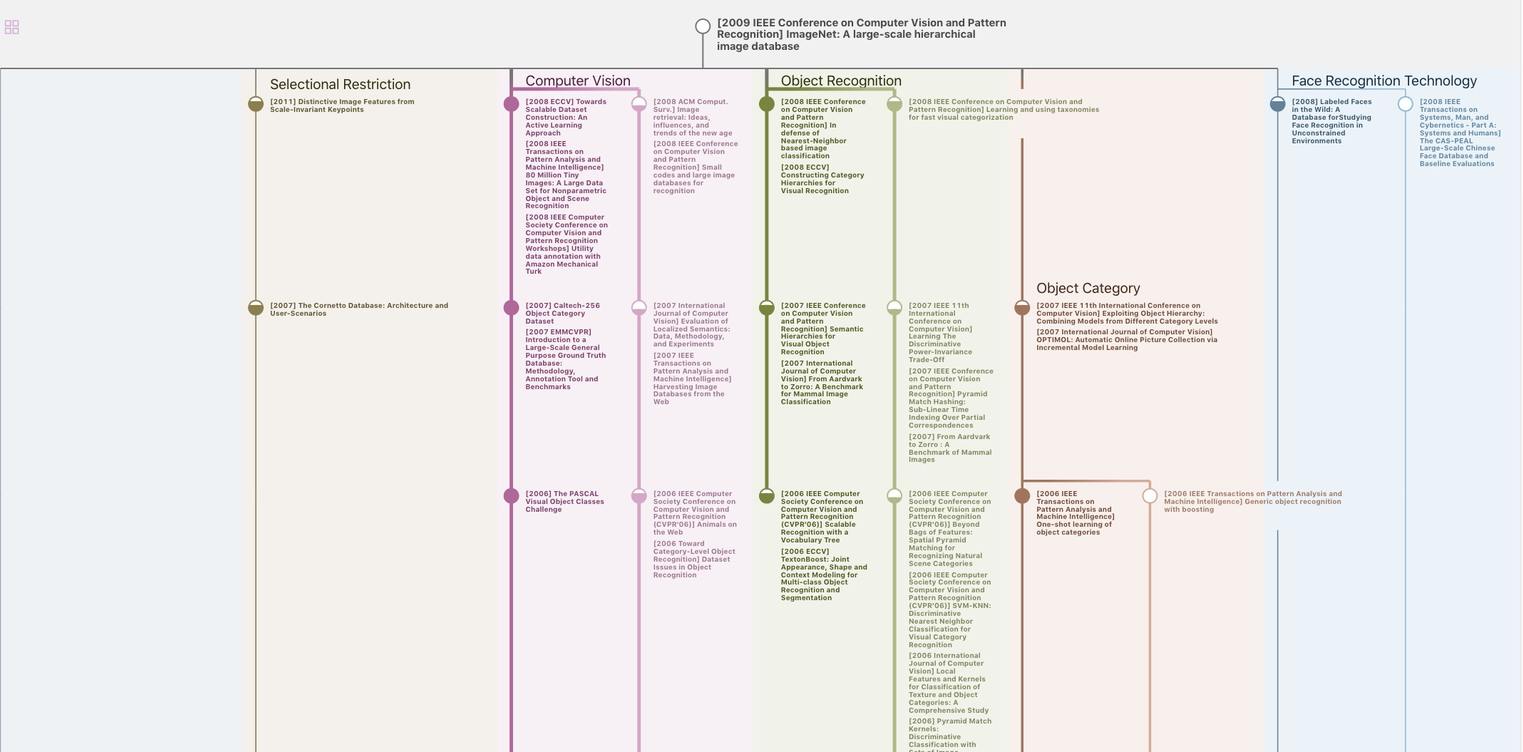
生成溯源树,研究论文发展脉络
Chat Paper
正在生成论文摘要