Early Prediction of Ion Transport Properties in Solid Polymer Electrolytes Using Machine Learning and System Behavior-Based Descriptors of Molecular Dynamics Simulations
MACROMOLECULES(2023)
摘要
Molecular dynamics simulations are useful tools to screensolidpolymer electrolytes with suitable properties applicable to Li-ionbatteries. However, due to the vast design space of polymers, it ishighly desirable to accelerate the screening by reducing the computationaltime of ion transport properties from simulations. In this study,we show that with a judicious choice of descriptors we can predictthe equilibrium ion transport properties in LiTFSI-homopolymersystems within the first 0.5 ns of the production run of simulations.Specifically, we find that descriptors that include information aboutthe behavior of the system, such as ion clustering and time evolutionof ion transport properties, have several advantages over polymerstructure-based descriptors, as they encode system (polymer and salt)behavior rather than just the class of polymers and can be computedat any time point during the simulations. These characteristics increasethe applicability of our descriptors to a wide range of polymer systems(e.g., copolymers, blend of polymers, salt concentrations, and temperatures)and can be impactful in significantly shortening the discovery pipelinefor solid polymer electrolytes.
更多查看译文
关键词
solid polymer electrolytes,prediction transport properties,machine learning,behavior-based
AI 理解论文
溯源树
样例
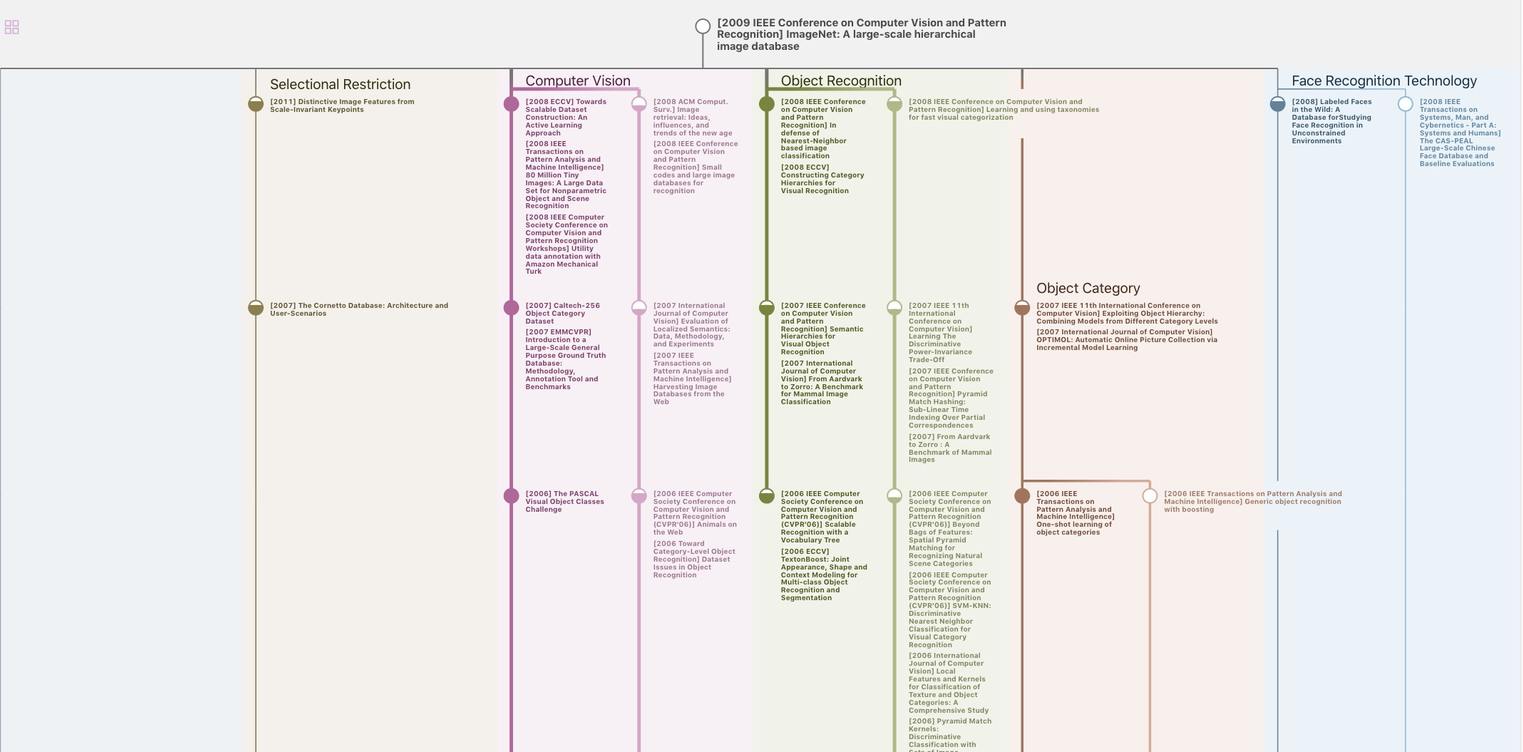
生成溯源树,研究论文发展脉络
Chat Paper
正在生成论文摘要