Distance function embedded genetic algorithm for maximizing the group efficacy measure of performance of machine-part cells
SOFT COMPUTING(2023)
摘要
Cell formation problems in cellular manufacturing deal with the processing of similar part families in a machine group with the aim of variation of cell load and exceptional element minimization. We propose a novel hybrid intelligent approach consisting of a genetic algorithm (GA) and distance function. The ideal and anti-ideal solutions are obtained by independently executing the GA two times. The solutions obtained at this phase are used in the subsequent phase. With the help of the input from the previous phase, the final machine-part cell formation is obtained using the GA by considering the two objectives simultaneously. A GUI-based software toolkit is also developed for numerical testing. We selected 30 benchmark test problems from the literature. The results show that the group efficacy (GE) measure is better for most problems than the existing literature. We develop a toolkit with GUI and assess the performance of 30 benchmark test problems. We obtain better performance measures for most of the problems compared to the latest available methods. The average improvement of the GE is 2.43 and 3.97, respectively, compared to the conventional and hybrid GAs.
更多查看译文
关键词
Manufacturing system design,Cell formation,Distance function,Genetic algorithms,Group efficacy
AI 理解论文
溯源树
样例
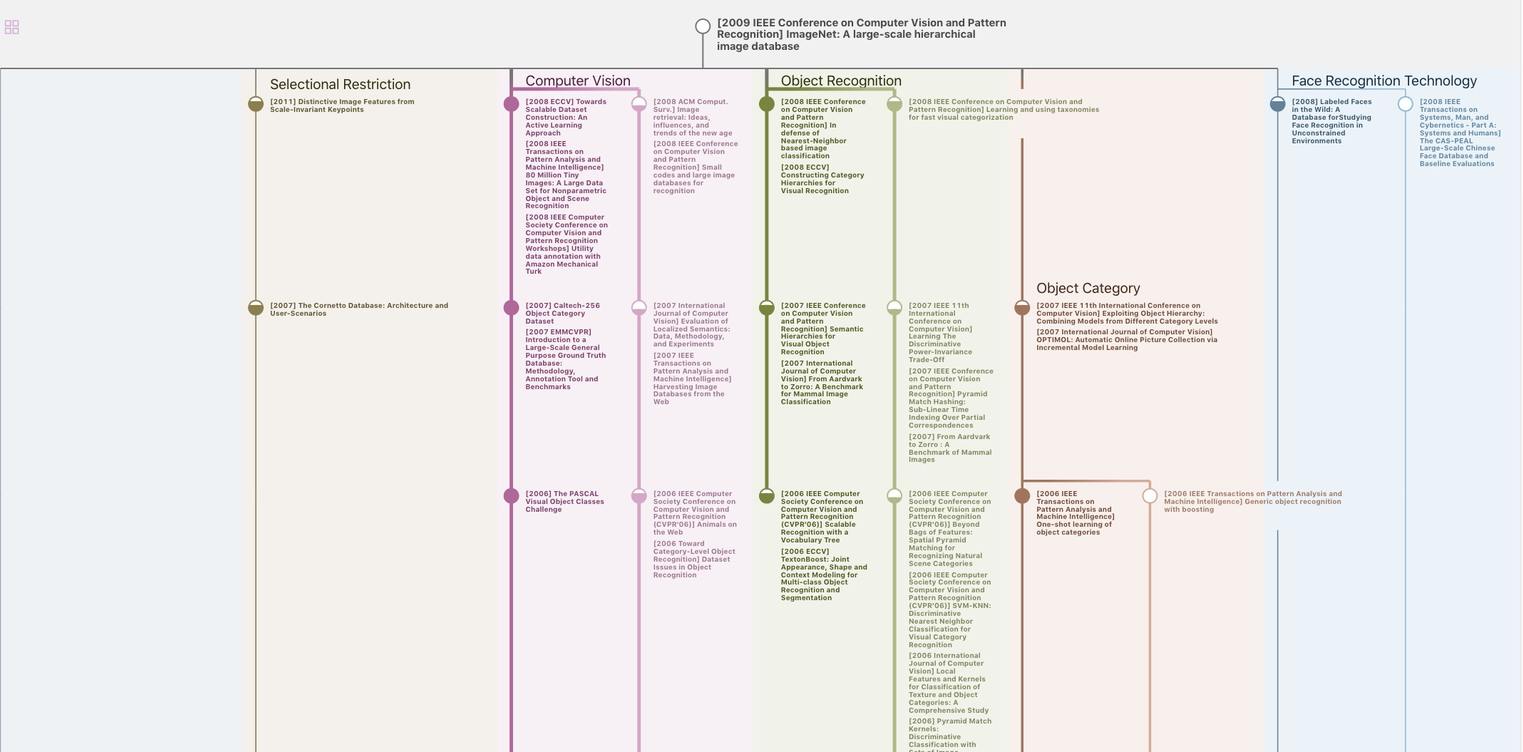
生成溯源树,研究论文发展脉络
Chat Paper
正在生成论文摘要