Deep Learning-Based Segmentation for Field Evaluation of Riprap and Large-Sized Aggregates
GEO-CONGRESS 2023: GEOTECHNICAL CHARACTERIZATION(2023)
摘要
Aggregate materials play essential roles in the sustainable performance of engineering applications. Size and shape properties of these aggregates determine the composition and packing of the aggregate assemblies, which impact layer strength, modulus, and deformation response under wheel loading. Current state-of-the-practice methods to evaluate aggregate properties rely on visual inspection, manual measurements, material sampling, and laboratory sieve analysis, as well as ground penetrating radar (GPR) for on-site inspection. Advanced aggregate imaging systems developed to date primarily focused on measurement of separated or slightly contacting aggregate particles, which is not applicable for densely stacked aggregate assemblies under field conditions. In this regard, development of a reliable, accurate, and cost-effective field evaluation technique is crucial. This paper presents an innovative approach using deep learning-based image segmentation techniques for evaluating aggregate materials in the field. The approach requires preparing an image data set comprised of aggregate images collected from various field and laboratory sites, where each image is manually labeled to identify individual particle locations by tracing their boundaries. Then, a state-of-the-art object detection and segmentation framework, called Mask R-CNN, is trained on the task-specific data set to develop the image segmentation kernel. Finally, the trained segmentation kernel is used to segment individual particles, and post-processing provides the morphological indices for the segmented particles. This segmentation approach has been utilized for the field evaluation of large-sized riprap materials and medium-sized ballast materials, with further performance tuning to address various field conditions. The segmentation results show good agreement with the ground-truth labeling and greatly improves the accuracy and efficiency of aggregate field evaluation procedures. Based on the presented findings, the deep learning-based segmentation approach can serve as an efficient and reliable tool for determining aggregate shape and morphological properties in field evaluation applications.
更多查看译文
AI 理解论文
溯源树
样例
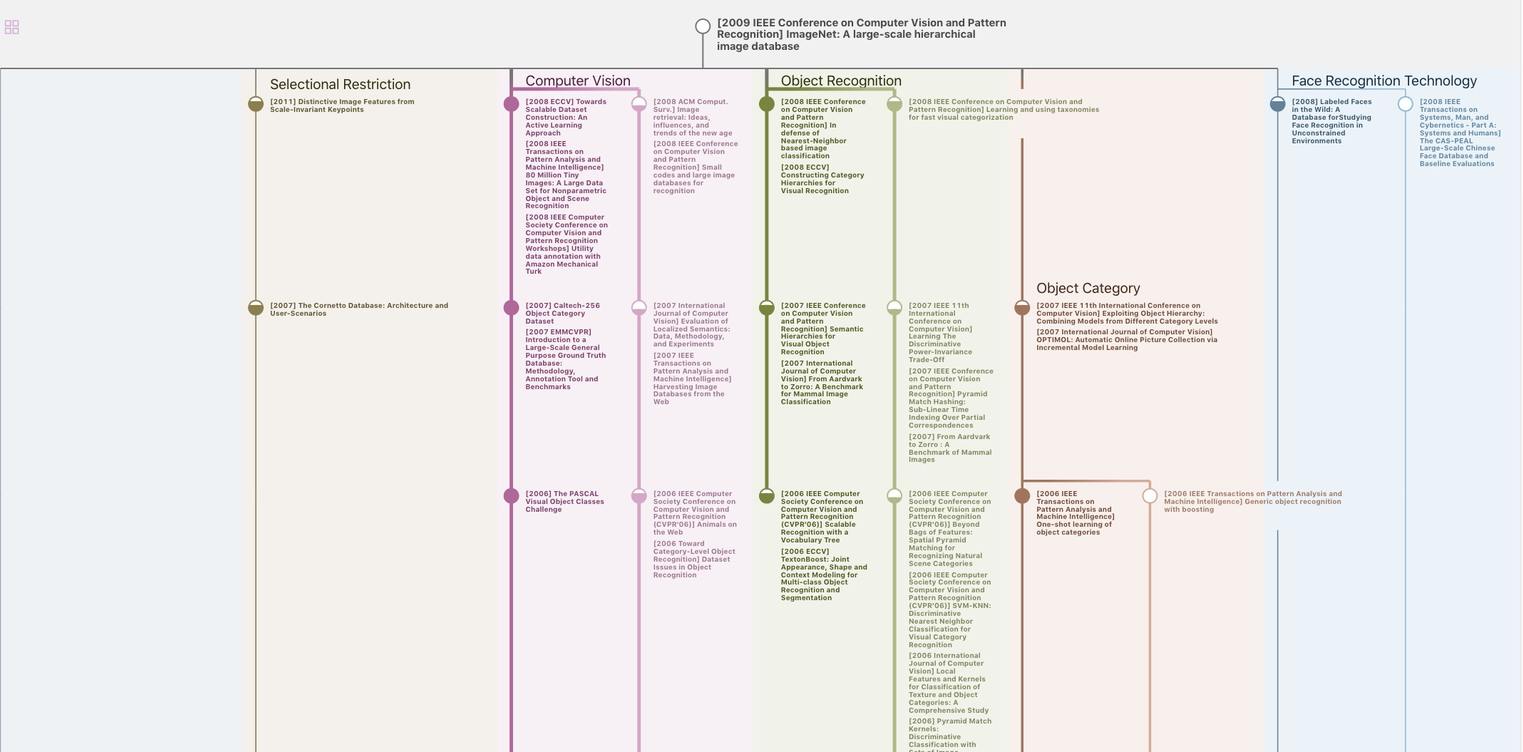
生成溯源树,研究论文发展脉络
Chat Paper
正在生成论文摘要