Maximum likelihood estimate sharing for collective perception in static environments for swarm robotics
ROBOTICA(2023)
摘要
Collective decision-making by a swarm of robots is of paramount importance. In particular, the problem of collective perception wherein a swarm of robots aims to achieve consensus on the prevalent feature in the environment. Recently, this problem has been formulated as a discrete collective estimation scenario to estimate their proportion rather than deciding about the prevalent one. Nevertheless, the performance of the existing strategies to resolve this scenario is either poor or depends on higher communication bandwidth. In this work, we propose a novel decision-making strategy based on maximum likelihood estimate sharing (MLES) to resolve the discrete collective estimation scenario. Experimentally, we compare the tradeoff speed versus accuracy of MLES with state-of-the-art methods in the literature, such as direct comparison (DC) and distributed Bayesian belief sharing (DBBS). Interestingly, MLES achieves an accurate consensus nearly 20% faster than DBBS, its communication bandwidth requirement is the same as DC but six times less than DBBS, and its computational complexity is $O(1)$ . Furthermore, we investigate how noisy sensors affect the effectiveness of the strategies under consideration, with MLES showing better sustainability.
更多查看译文
关键词
collective perception,maximum likelihood estimate,static environments
AI 理解论文
溯源树
样例
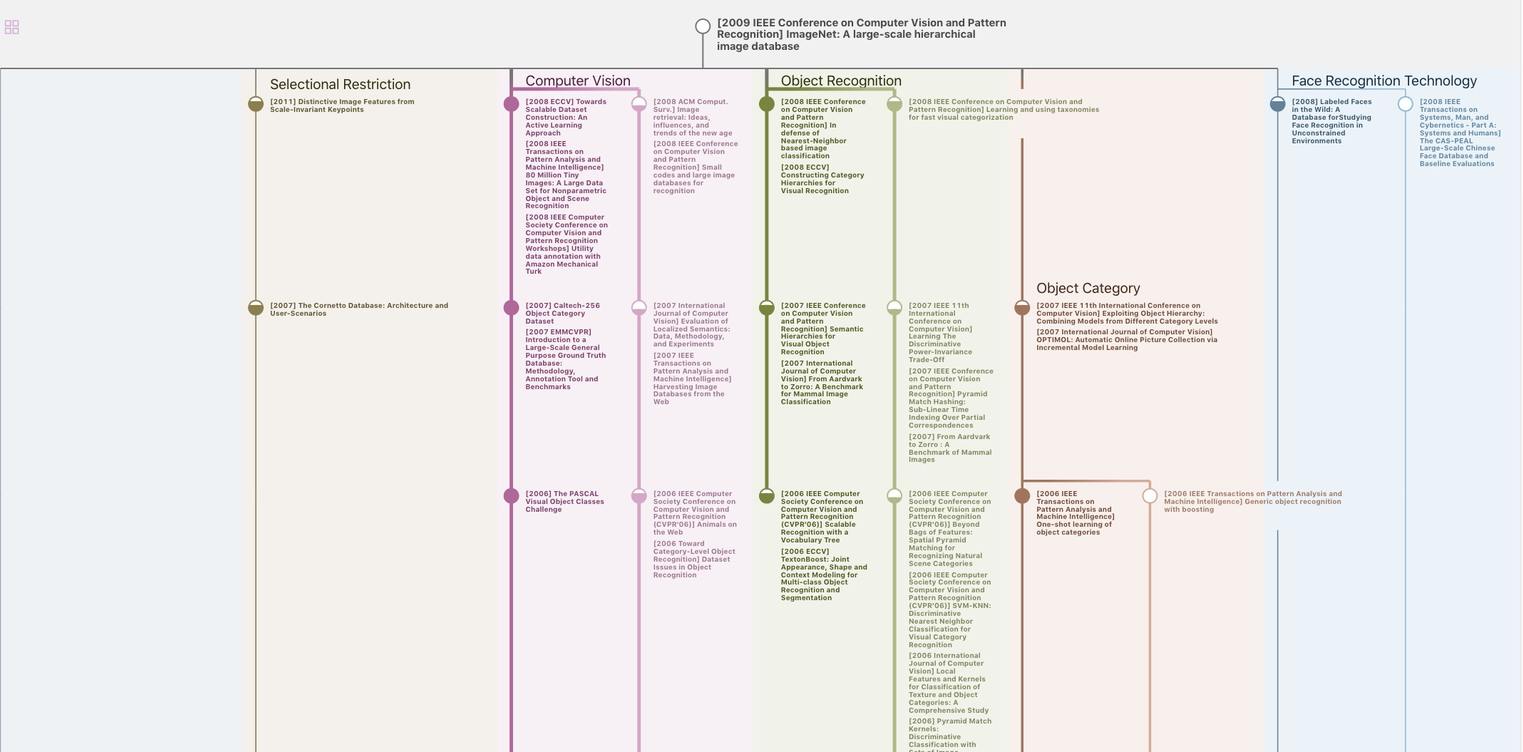
生成溯源树,研究论文发展脉络
Chat Paper
正在生成论文摘要