Optimized hybrid YOLOu-Quasi-ProtoPNet for insulators classification
IET GENERATION TRANSMISSION & DISTRIBUTION(2023)
摘要
To ensure the electrical power supply, inspections are frequently performed in the power grid. Nowadays, several inspections are conducted considering the use of aerial images since the grids might be in places that are difficult to access. The classification of the insulators' conditions recorded in inspections through computer vision is challenging, as object identification methods can have low performance because they are typically pre-trained for a generalized task. Here, a hybrid method called YOLOu-Quasi-ProtoPNet is proposed for the detection and classification of failed insulators. This model is trained from scratch, using a personalized ultra-large version of YOLOv5 for insulator detection and the optimized Quasi-ProtoPNet model for classification. For the optimization of the Quasi-ProtoPNet structure, the backbones VGG-16, VGG-19, ResNet-34, ResNet-152, DenseNet-121, and DenseNet-161 are evaluated. The F1-score of 0.95165 was achieved using the proposed approach (based on DenseNet-161) which outperforms models of the same class such as the Semi-ProtoPNet, Ps-ProtoPNet, Gen-ProtoPNet, NP-ProtoPNet, and the standard ProtoPNet for the classification task.
更多查看译文
关键词
insulators
AI 理解论文
溯源树
样例
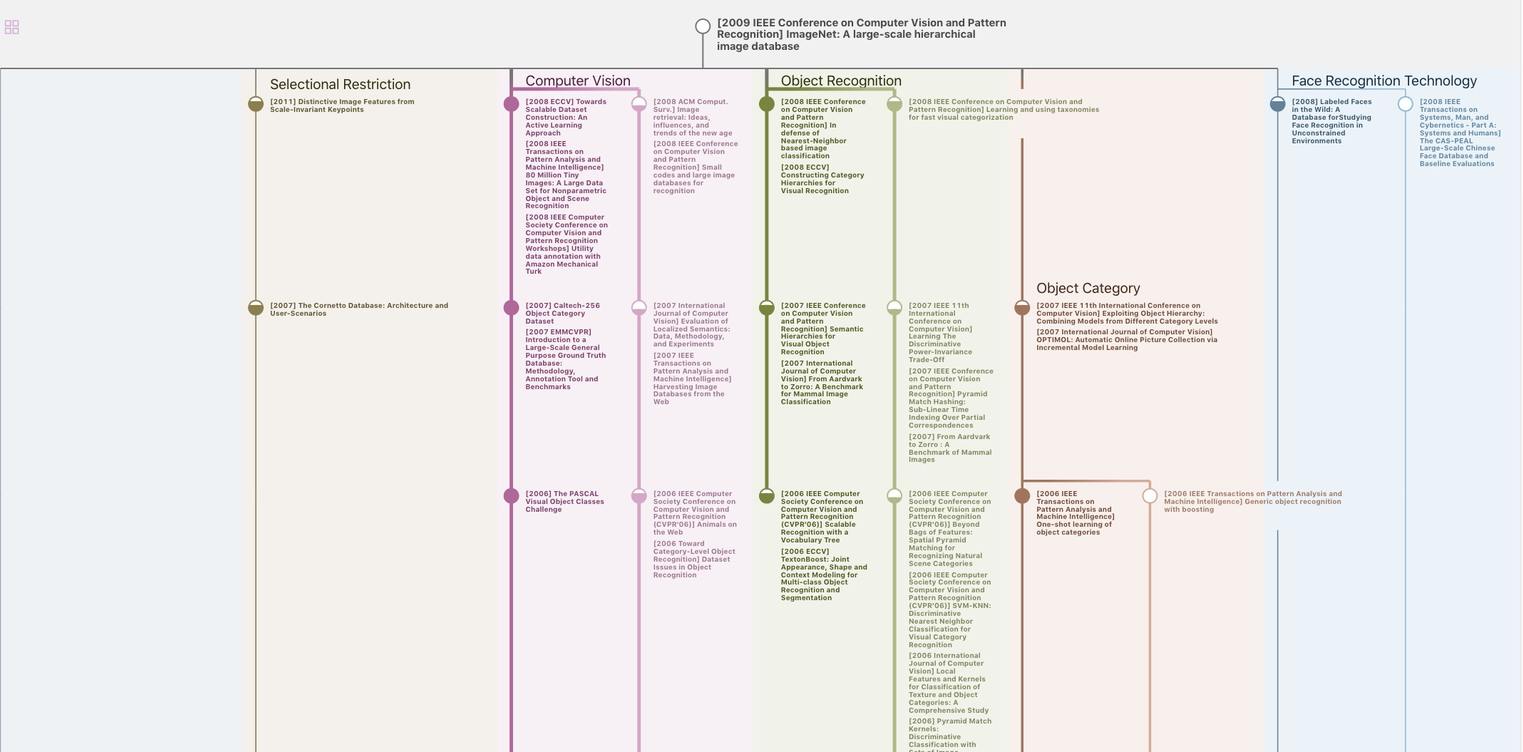
生成溯源树,研究论文发展脉络
Chat Paper
正在生成论文摘要