Inverse-designed growth-based cellular metamaterials
MECHANICS OF MATERIALS(2023)
摘要
Advancements in machine learning have sparked significant interest in designing mechanical metamaterials, i.e., materials that derive their properties from their inherent microstructure rather than just their constituent material. We propose a data-driven exploration of the design space of growth-based cellular metamaterials based on star-shaped distances. These two-dimensional metamaterials are based on periodically-repeating unit cells consisting of material and void patterns with non-trivial geometries. Machine learning models exploiting large datasets are then employed to inverse design growth-based metamaterials for tailored anisotropic stiffness. Firstly, a forward model is created to bypass the growth and homogenization process and accurately predict the mechanical properties given a finite set of design parameters. Secondly, an inverse model is used to invert the structure-property maps and enable the accurate prediction of designs for a given anisotropic stiffness query. We successfully demonstrate the frameworks' generalization capabilities by inverse designing for stiffness properties chosen from outside the domain of the design space.
更多查看译文
关键词
Cellular metamaterials,Machine learning,Inverse Design,Growth process
AI 理解论文
溯源树
样例
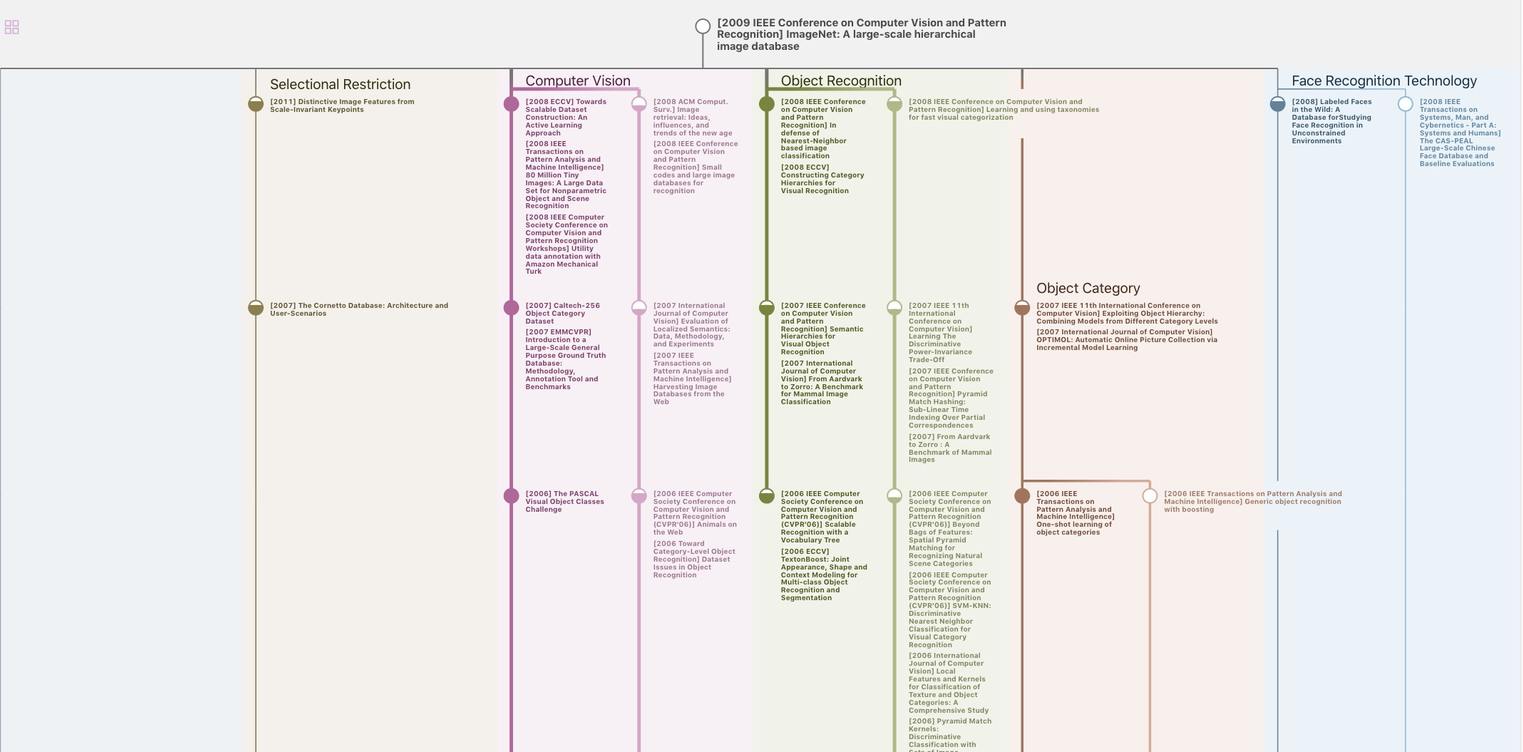
生成溯源树,研究论文发展脉络
Chat Paper
正在生成论文摘要