Classification-Detection of Metal Surfaces under Lower Edge Sharpness Using a Deep Learning-Based Approach Combined with an Enhanced LoG Operator
CMES-COMPUTER MODELING IN ENGINEERING & SCIENCES(2023)
摘要
Metal flat surface in-line surface defect detection is notoriously difficult due to obstacles such as high surface reflectivity, pseudo-defect interference, and random elastic deformation. This study evaluates the approach for detecting scratches on a metal surface in order to address a problem in the detection process. This paper proposes an improved Gauss-Laplace (LoG) operator combined with a deep learning technique for metal surface scratch identification in order to solve the difficulties that it is challenging to reduce noise and that the edges are unclear when utilizing existing edge detection algorithms. In the process of scratch identification, it is challenging to differentiate between the scratch edge and the interference edge. Therefore, local texture screening is utilized by deep learning techniques that evaluate and identify scratch edges and interference edges based on the local texture characteristics of scratches. Experiments have proven that by combining the improved LoG operator with a deep learning strategy, it is able to effectively detect image edges, distinguish between scratch edges and interference edges, and identify clear scratch information. Experiments based on the six categories of meta scratches indicate that the proposed method has achieved rolled-in crazing (100%), inclusion (94.4%), patches (100%), pitted (100%), rolled (100%), and scratches (100%), respectively.
更多查看译文
关键词
metal surfaces,lower edge sharpness,classification-detection,learning-based
AI 理解论文
溯源树
样例
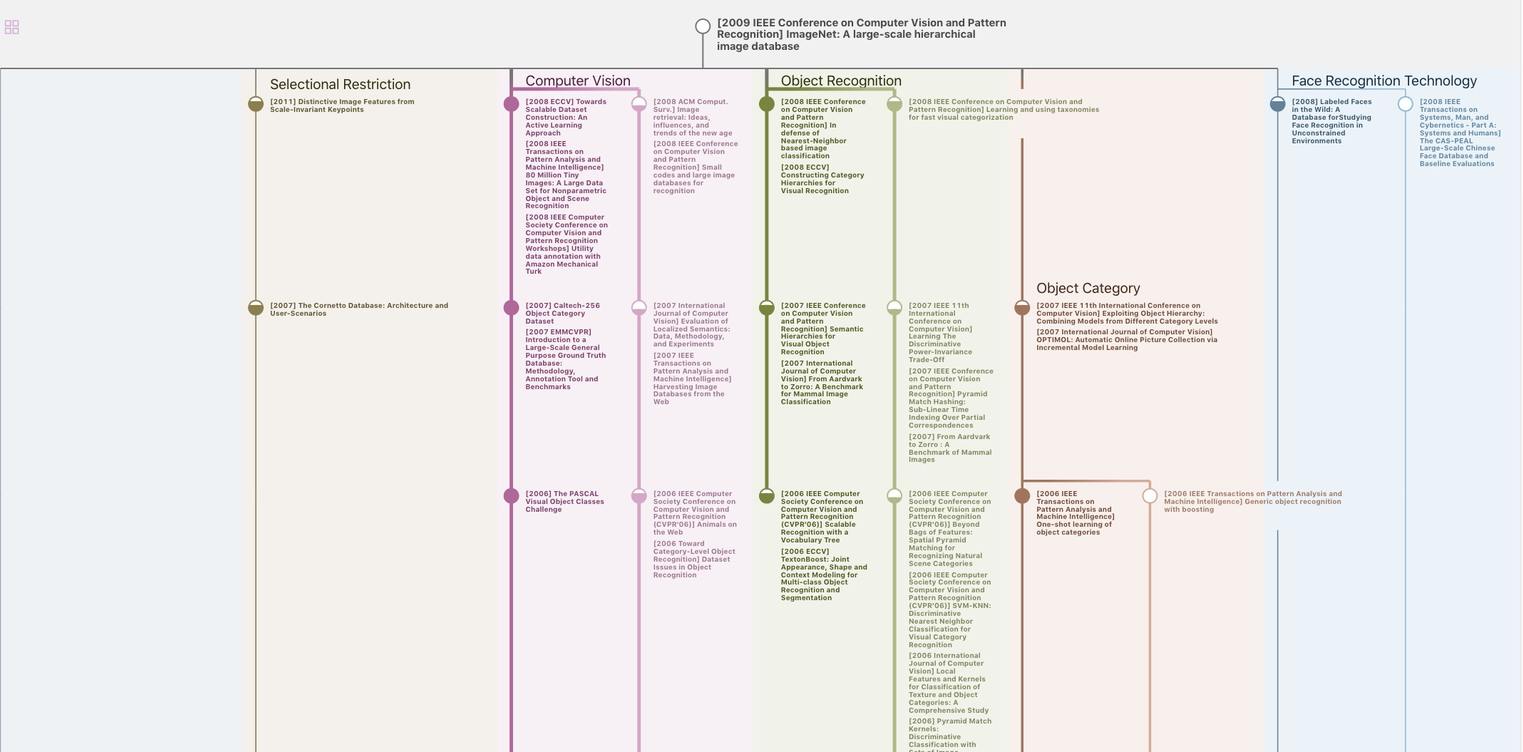
生成溯源树,研究论文发展脉络
Chat Paper
正在生成论文摘要