A Unified Diagnostic Framework Via Symmetrized Data Aggregation
IISE TRANSACTIONS(2024)
摘要
In statistical process control of high-dimensional data streams, in addition to online monitoring of abnormal changes, fault diagnosis of responsible components has become increasingly important. Existing diagnostic procedures have been designed for some typical models with distribution assumptions. Moreover, there is a lack of systematic approaches to provide a theoretical guarantee of significance in estimating shifted components. In this article, we introduce a new procedure to control the False Discovery Rate (FDR) of fault diagnosis. The proposed method formulates the fault diagnosis as a variable selection problem and utilizes the symmetrized data aggregation technique via sample splitting, data screening, and information pooling to control the FDR. Under some mild conditions, we show that the proposed method can achieve FDR control asymptotically. Extensive numerical studies and two real-data examples demonstrate satisfactory FDR control and remarkable diagnostic power in comparison to existing methods.
更多查看译文
关键词
Fault diagnosis,false discovery rate,variable selection,symmetrized data aggregation
AI 理解论文
溯源树
样例
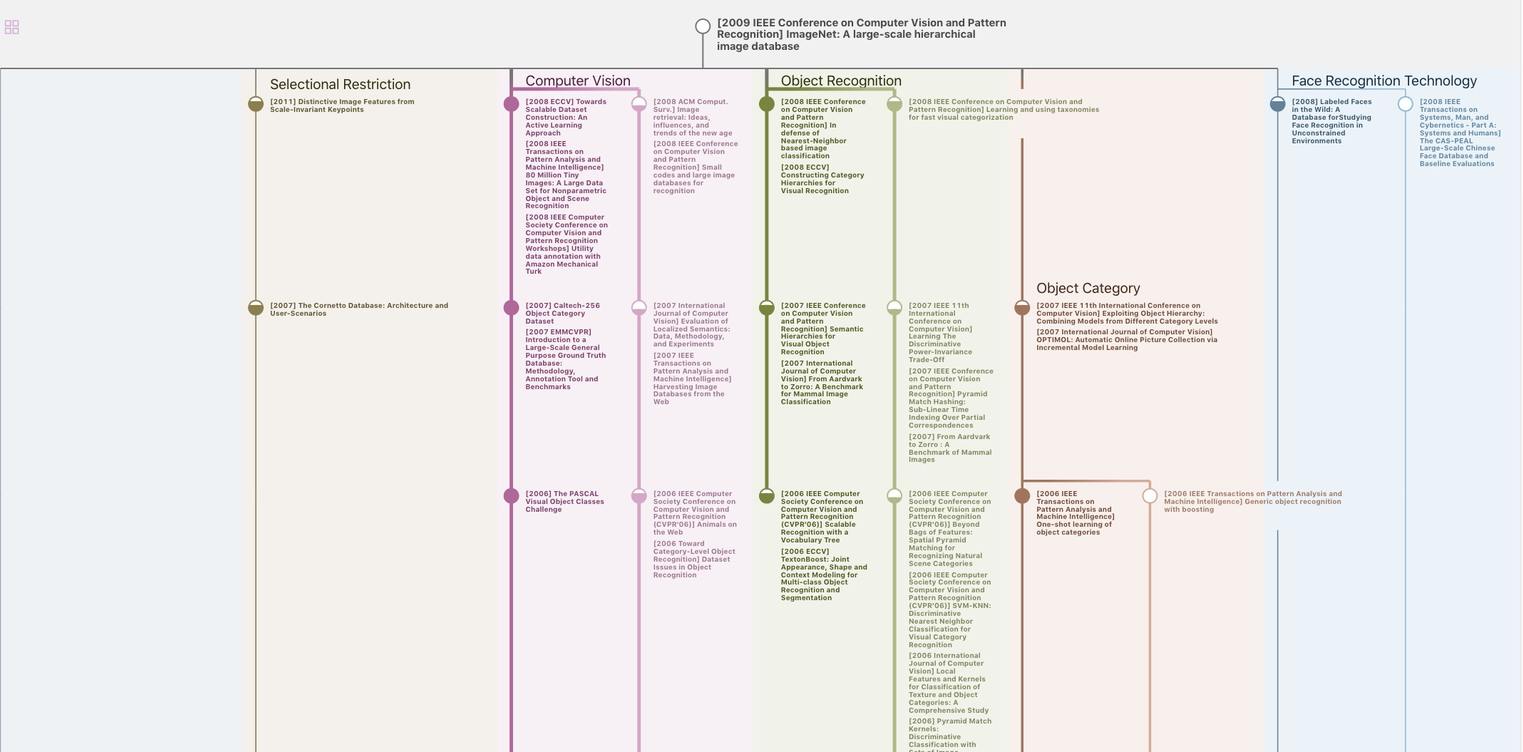
生成溯源树,研究论文发展脉络
Chat Paper
正在生成论文摘要