Utilizing Contrastive Learning for Graph-Based Active Learning of SAR Data
ALGORITHMS FOR SYNTHETIC APERTURE RADAR IMAGERY XXX(2023)
摘要
Automatic target recognition with synthetic aperture radar (SAR) data is a challenging image classification problem due to the difficulty in acquiring the large labeled training sets required for conventional deep learning methods. Recent work(1) addressed this problem by utilizing powerful tools in graph-based semi-supervised learning and active learning, and achieved state of the art results on the Moving and Stationary Target Acquisition and Recognition (MSTAR) dataset with less labeled data compared to existing techniques. A key part of the previous work was the use of unsupervised deep learning, in particular, a convolutional variational autoencoder, to embed the MSTAR images into a meaningful feature space prior to constructing the similarity graph. In this paper, we develop a contrastive SimCLR framework for feature extraction from MSTAR images by using data augmentations specific to SAR imagery. We show that our contrastive embedding results in improved performance over the variational autoencoder similarity graph method in automatic target recognition on the MSTAR dataset. We also perform a comparative study of the quality of the autoencoder and contrastive embeddings by training support vector machines (SVM) at various label rates, applying spectral clustering, and evaluating graph-cut energies, all of which show that the contrastive learning embedding is superior to the autoencoder embedding.
更多查看译文
关键词
Contrastive Learning, Active Learning, Synthetic Aperture Radar, Graph-Based Learning
AI 理解论文
溯源树
样例
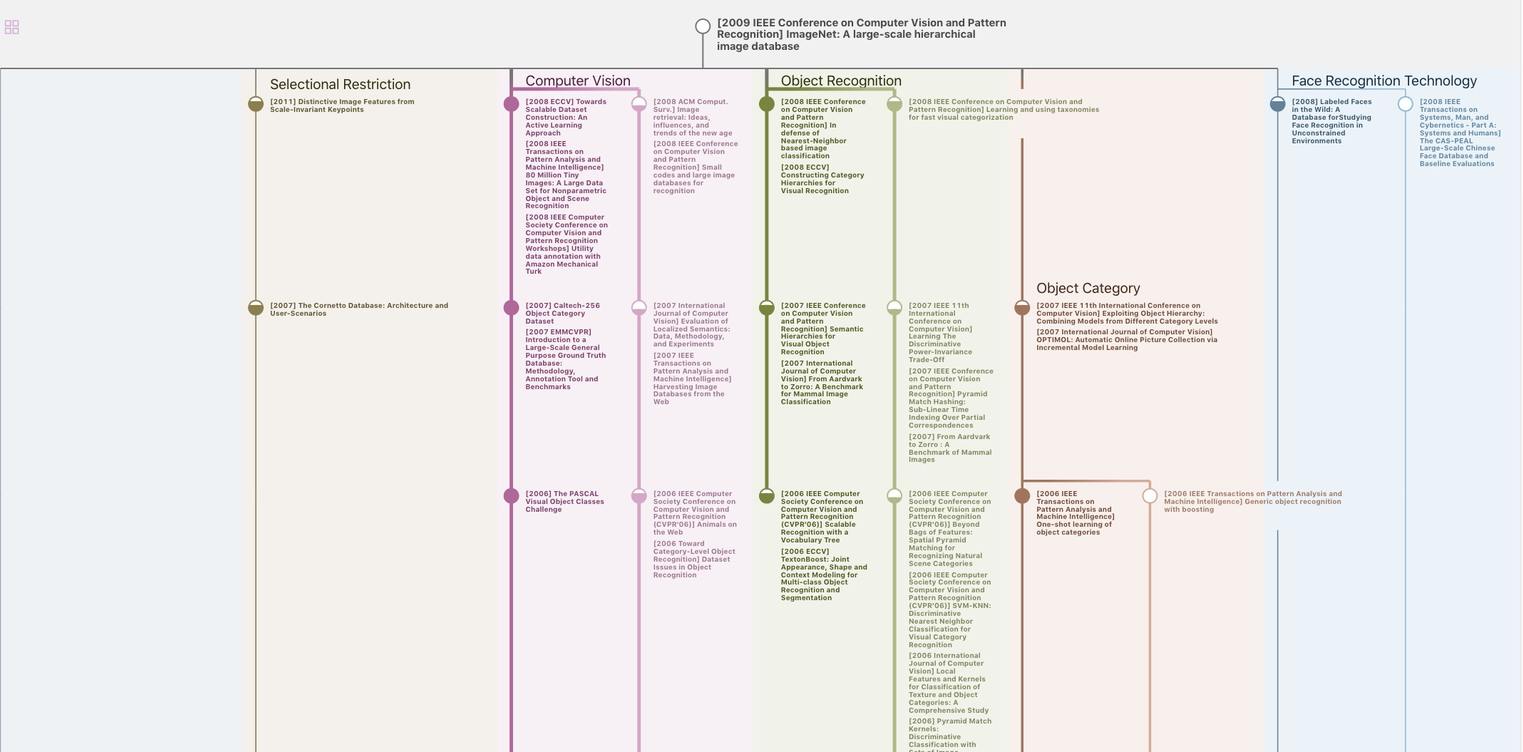
生成溯源树,研究论文发展脉络
Chat Paper
正在生成论文摘要