Self-guided attention denoising network for pre-stack seismic data: from coarse to fine
JOURNAL OF SEISMIC EXPLORATION(2023)
摘要
Background noise attenuation is one of the most essential steps in seismic data processing. Residual background noise is likely to cause some artifacts in the following seismic imaging, thus bringing huge difficulties to the final interpretation. In recent years, deep-learning (DL) methods based on data driven strategy, especially the convolutional neural network (CNN), work well in seismic noise attenuation. In addition, it is applied automatically without parameter fine-tuning after training. To further improve their performance, we propose a novel architecture: self-guided attention network (SGA-Net) by combining self-guided strategy and spatial attention mechanism. Different from most of the conventional CNNs, this proposed SGA-Net can capture multi-scale features by performing the convolution operation on seismic data with different resolutions. In this network, the self-guided strategy is adopted to take full advantage of the multi-scale features; specifically, we utilize the global coarse features extracted at low resolution to guide the extraction process of local finer features at higher resolution. Furthermore, we design a spatial attention module with two inputs to fuse the global coarse and local fine features. We set up four competitive methods for SGA-Net including two traditional seismic denoising methods and two existing DL denoising methods in both synthetic and real experiments and experimental results demonstrate the advantage of SGA-Net both in noise attenuation and signal preservation.
更多查看译文
关键词
deep-learning, seismic noise attenuation, convolutional neural network, signal recovery
AI 理解论文
溯源树
样例
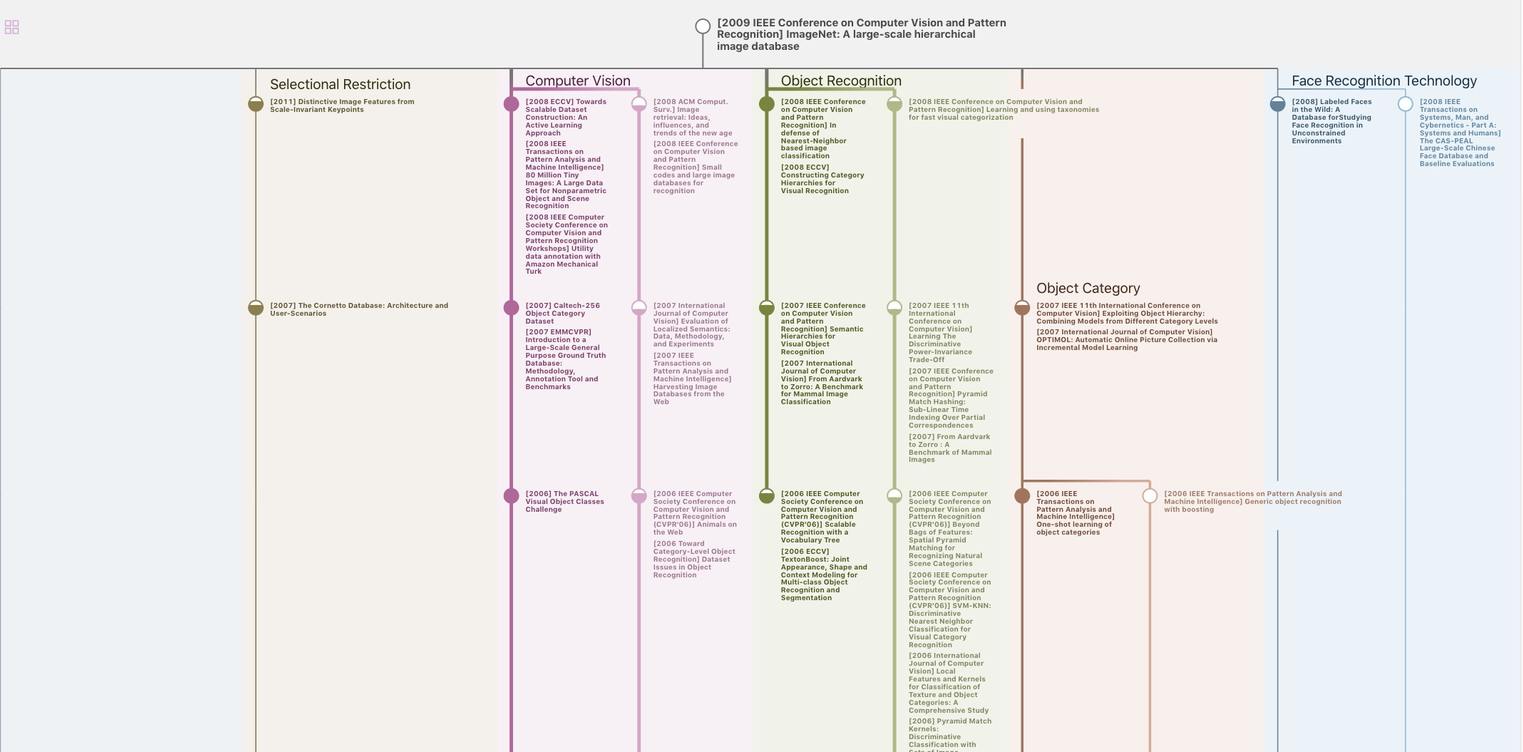
生成溯源树,研究论文发展脉络
Chat Paper
正在生成论文摘要