Visible-infrared person re-identification employing style-supervision and content-supervision
VISUAL COMPUTER(2024)
摘要
Cross-modal visible-infrared person re-identification (VI-ReID) aims to retrieve images of the same pedestrians captured by visible (VIS) cameras and infrared (IR) cameras and it is a challenging task in intelligent security systems. The differences in imaging principles between visible and infrared images lead to large cross-modal differences and intra-class differences, and such cross-modal image differences can be considered as special image style differences, while several intra-class differences can be considered as differences in the form of content expression between visible and infrared images. Some state-of-the-art methods improve the performance of the VI-ReID model by using additional feature enhancement or feature generation modules, however, these methods also introduce additional parameters and increase the training cost. In this paper, to mitigate the differences in image style and content between VIS and IR images, we design two objective functions based on content and style, which are style loss and content loss for the VI-ReID task, respectively. Our model can effectively mitigate the differences between modes by optimizing the objective function to map VIS and IR features into the same feature space without additional auxiliary modules. After extensive experiments, our model achieves competitive performance on two challenging datasets. Notably, under the visible2infrared setting on the RegDB dataset, our model achieves the state-of-the-art (SOTA) Rank-1/mAP/mINP = 96.13%/91.35%/83.67%.
更多查看译文
关键词
Visible-infrared person re-identification,Cross-modal,Instance normalization module,Spatial feature mapping,Image style and content
AI 理解论文
溯源树
样例
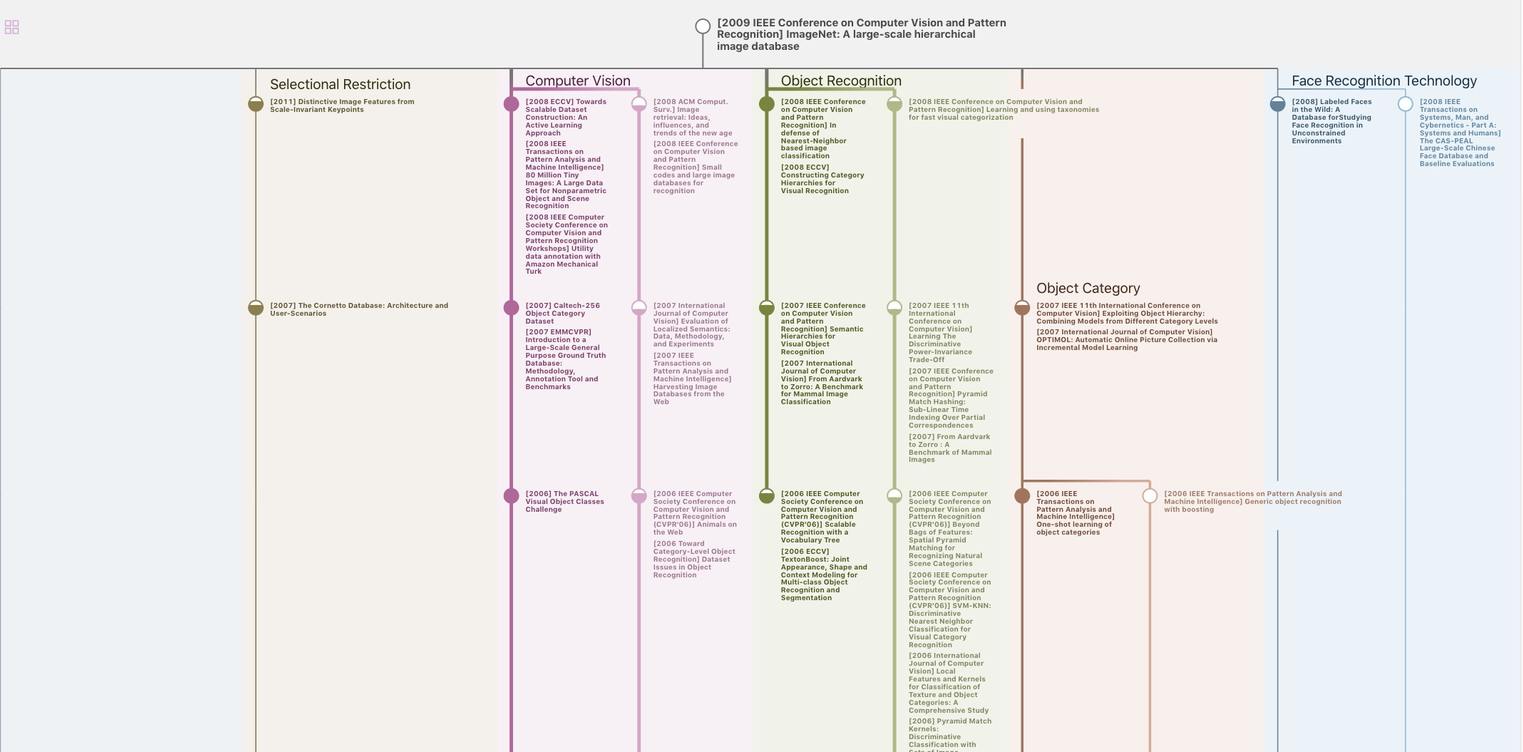
生成溯源树,研究论文发展脉络
Chat Paper
正在生成论文摘要