Dryland farming wheat yield prediction using the Lasso regression model and meteorological variables in dry and semi-dry region
STOCHASTIC ENVIRONMENTAL RESEARCH AND RISK ASSESSMENT(2023)
摘要
The risk of climate change and international market fluctuations complicate crop production. Wheat is considered one of the most strategic products in food security. Dryland farming of wheat is prevalent in many parts of the world, and it occupies a large part of the cultivated land. However, its performance is highly dependent on weather conditions and changes. Yield prediction models could be used for planning purposes when dealing with changes in yield. This study analyzed the effectiveness of the least absolute shrinkage and selection operator (LASSO) model in selecting variables for predicting dryland wheat yield in southwestern Iran. The model was used with 45 weather-based variables across annual, seasonal, and monthly time frames. The results showed that temperature, evaporation, and extreme temperatures followed by radiation and precipitation variables categories, are effective meteorological variables in estimating dryland farming wheat yield in the study area. Monthly timescale could estimate yield with minimum error compared to other timescales. However, considering all selected variables regardless of their timescale (total) results, the best estimation in most districts with the model’s R 2 and normalized root mean square error (NRMSE) varied between 57.98–99.50 and 1.46–21.94, respectively. Therefore, the LASSO regression could be used reliably for each district considering the most effective meteorological parameters in that region for accurate decision-makers policies.
更多查看译文
关键词
lasso regression model,wheat,meteorological variables,farming,semi-dry
AI 理解论文
溯源树
样例
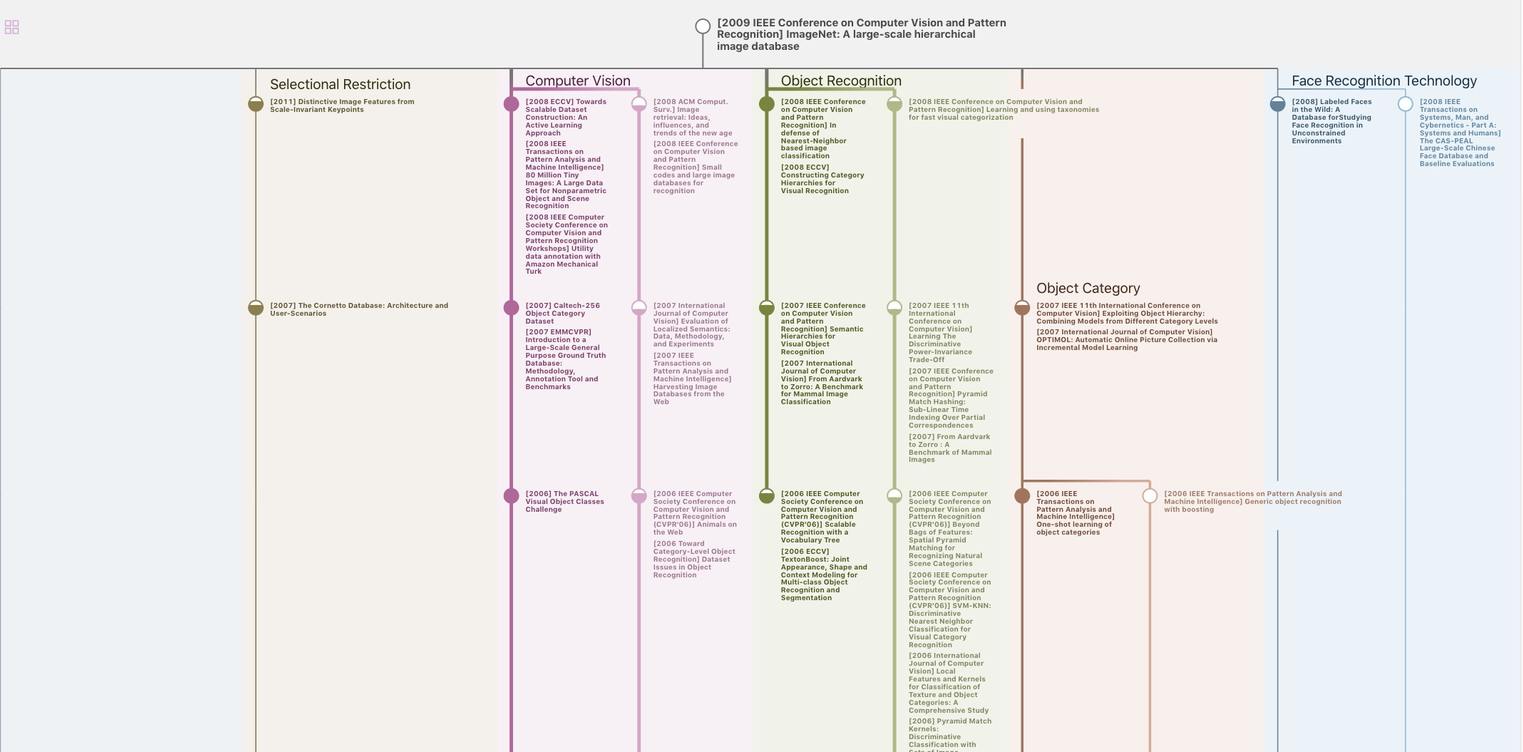
生成溯源树,研究论文发展脉络
Chat Paper
正在生成论文摘要