Predicting the Thermal Protective Performance of Smart Fabric Systems With a Shape Memory Layer Using Machine Learning Models
CLOTHING AND TEXTILES RESEARCH JOURNAL(2023)
摘要
The utilization of shape memory alloy (SMA) in shape memory fabric (SMF) has revolutionized thermal protective clothing, significantly enhancing its thermal protection. However, the cost- and time-consuming process of SMA shape memory training and performance testing can be optimized for improved efficiency. This study addresses this challenge by developing machine learning models to predict the thermal protection of a smart fabric system (SFS) with a SMF. The training data was sourced from the previous experimental studies, and six features significantly impacting thermal protection were identified. Results demonstrated that gradient boosting regressor (GBR) model exhibited the highest accuracy, with the SMA interval emerging as the most critical feature in determining thermal protection. Moreover, the GBR model predicted that SFS presented the best thermal protection when the dry SMF was woven by SMA of 2 cm interval and aramid 1414 of 20 roots/cm density, located between the moisture barrier and thermal liner vertically.
更多查看译文
关键词
smart fabric systems,thermal protective performance,shape memory layer,machine learning models
AI 理解论文
溯源树
样例
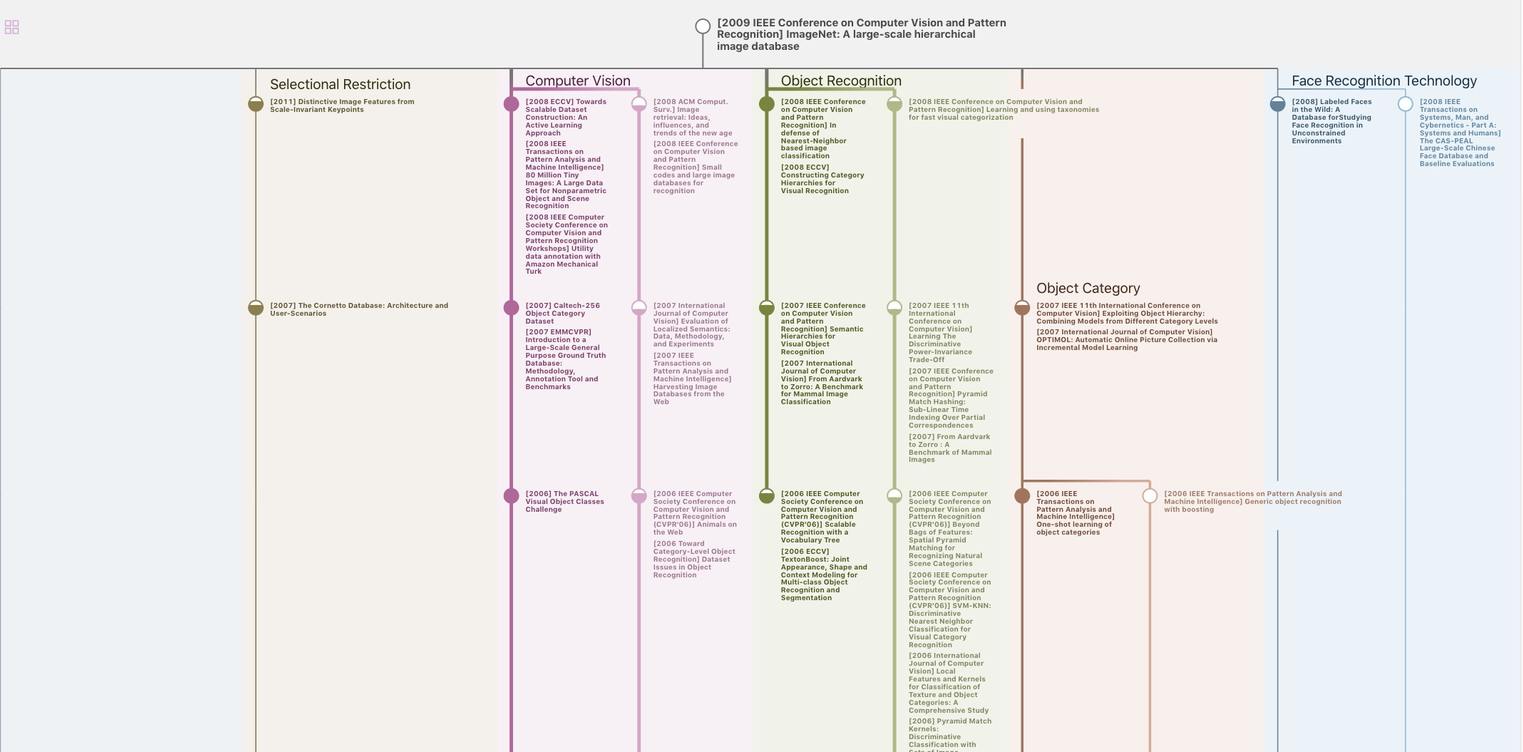
生成溯源树,研究论文发展脉络
Chat Paper
正在生成论文摘要