Semi-Supervised Learning forWearable-based Momentary Stress Detection in the Wild
PROCEEDINGS OF THE ACM ON INTERACTIVE MOBILE WEARABLE AND UBIQUITOUS TECHNOLOGIES-IMWUT(2023)
摘要
Physiological and behavioral data collected from wearable or mobile sensors have been used to estimate self-reported stress levels. Since stress annotation usually relies on self-reports during the study, a limited amount of labeled data can be an obstacle to developing accurate and generalized stress-predicting models. On the other hand, the sensors can continuously capture signals without annotations. This work investigates leveraging unlabeled wearable sensor data for stress detection in the wild. We propose a two-stage semi-supervised learning framework that leverages wearable sensor data to help with stress detection. The proposed structure consists of an auto-encoder pre-training method for learning information from unlabeled data and the consistency regularization approach to enhance the robustness of the model. Besides, we propose a novel active sampling method for selecting unlabeled samples to avoid introducing redundant information to the model. We validate these methods using two datasets with physiological signals and stress labels collected in the wild, as well as four human activity recognition (HAR) datasets to evaluate the generality of the proposed method. Our approach demonstrated competitive results for stress detection, improving stress classification performance by approximately 7% to 10% on the stress detection datasets compared to the baseline supervised learning models. Furthermore, the ablation study we conducted for the HAR tasks supported the effectiveness of our methods. Our approach showed comparable performance to state-of-the-art semi-supervised learning methods for both stress detection and HAR tasks.
更多查看译文
关键词
Wearable Data,Stress Detection,Semi-Supervised Learning,Time-Series Learning
AI 理解论文
溯源树
样例
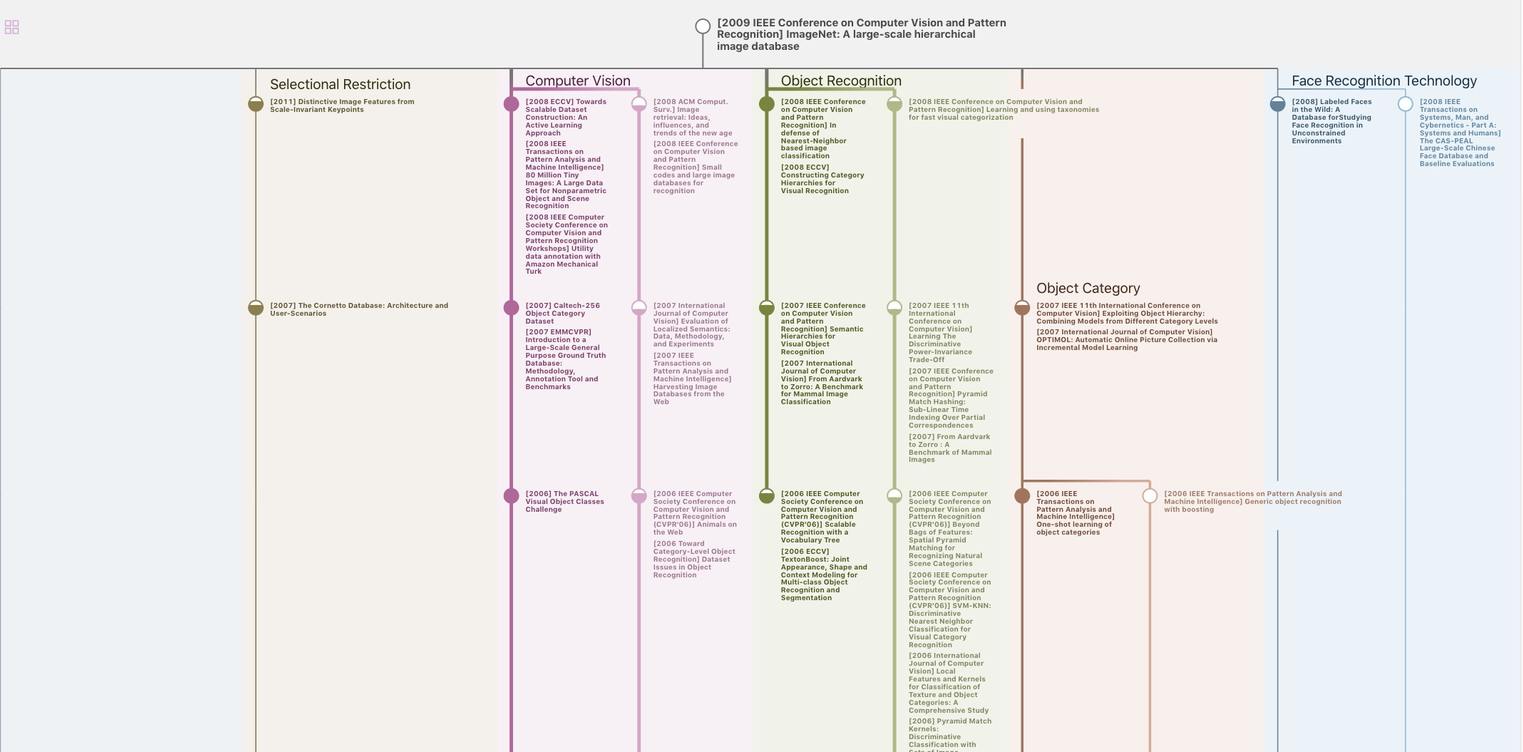
生成溯源树,研究论文发展脉络
Chat Paper
正在生成论文摘要