A Weakly-Supervised Method for Named Entity Recognition of Agricultural Knowledge Graph
INTELLIGENT AUTOMATION AND SOFT COMPUTING(2023)
Abstract
It is significant for agricultural intelligent knowledge services using knowledge graph technology to integrate multi-source heterogeneous crop and pest data and fully mine the knowledge hidden in the text. However, only some labeled data for agricultural knowledge graph domain training are available. Furthermore, labeling is costly due to the need for more data openness and standardization. This paper proposes a novel model using knowledge distillation for a weakly supervised entity recognition in ontology construction. Knowledge distillation between the target and source data domain is performed, where Bi-LSTM and CRF models are constructed for entity recognition. The experimental result is shown that we only need to label less than one-tenth of the data for model training. Furthermore, the agricultural domain ontology is constructed by BILSTM-CRF named entity recognition model and relationship extraction model. Moreover, there are a total of 13,983 entities and 26,498 relationships built in the neo4j graph database.
MoreTranslated text
Key words
Agricultural knowledge graph,entity recognition,knowledge distillation,transfer learning
AI Read Science
Must-Reading Tree
Example
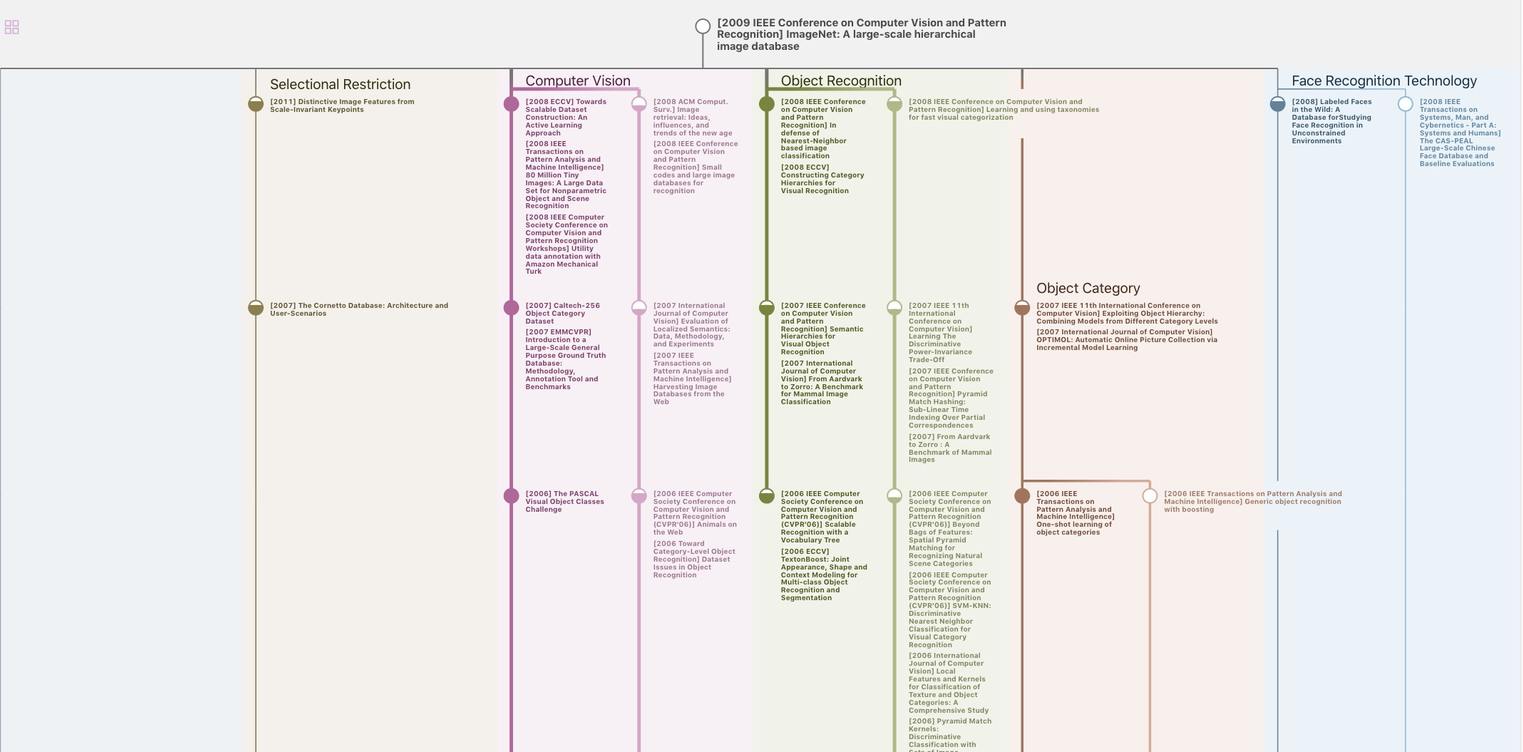
Generate MRT to find the research sequence of this paper
Chat Paper
Summary is being generated by the instructions you defined