Generalizable Deep Neural Network Based Multi-Material Hysteresis Modeling
2022 IEEE 20TH BIENNIAL CONFERENCE ON ELECTROMAGNETIC FIELD COMPUTATION-LONG PAPERS, CEFC-LONG(2022)
摘要
Over the past couple of decades, dependence on magnetic materials has increased tremendously. Since every kind of magnetic material behaves differently, it is very difficult to create a universal model of these behaviors. While there exist models and simulation tools that can represent the behavior of these materials, it may take days or even an entire week to compute when embedded in an analysis system, which is highly inefficient. With a good machine learning (ML) model, the computation time can be reduced by a significant amount with minimal error. To determine performance parameters such as the efficiency of an electrical machine, the hysteretic behavior of the material is crucial, and the representation used can impact the performance of a simulation tool. The goal of this work is to explore several, different deep learning (DL) network architectures which might both provide a generalized representation methodology for hysteresis problems and reduce the computational effort needed to include the impact of hysteresis in a finite element-based simulation over existing approaches.
更多查看译文
关键词
Convolutional neural networks,deep learning,feedforward neural network,magnetic hysteresis,recurrent neural network,transformers
AI 理解论文
溯源树
样例
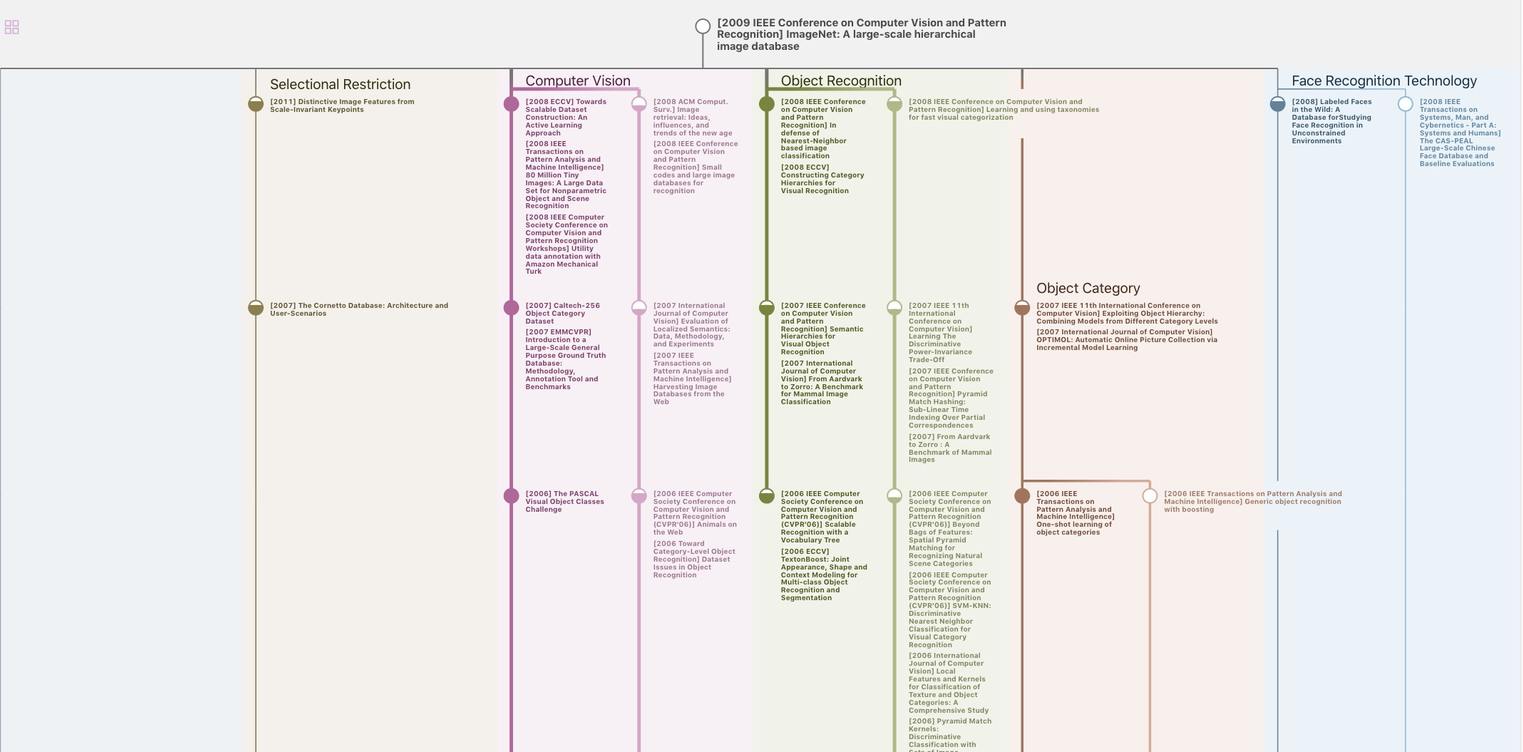
生成溯源树,研究论文发展脉络
Chat Paper
正在生成论文摘要