Multilayer Grad-CAM: An effective tool towards explainable deep neural networks for intelligent fault diagnosis
JOURNAL OF MANUFACTURING SYSTEMS(2023)
摘要
As a tool to explain deep neural networks using gradient information, Gradient-weighted Class Activation Map (Grad-CAM) provides a potential way for explainable artificial intelligence. However, for vibration signals in machine fault diagnosis, feature resolution of Grad-CAM decreases with the deepening of network layers, which weakens network explainability. To address this issue, a novel Multilayer Grad-CAM (MLG-CAM) is proposed as an effective tool to explain what networks have learned. Meanwhile, three indicators are defined to quantify explainability of deep neural networks. The MLG-CAM uses gradients flow of multiple convolutional layers to obtain activation maps in various resolutions. A comprehensive activation map is then produced by layerweighted summation of above activation maps. Experiments indicate MLG-CAM not only highlights cyclostationary impulses in time domain but also emphasizes fault characteristic frequency in frequency domain. These results prove MLG-CAM as an effective way to explain deep neural networks and build up trustworthiness of networks.
更多查看译文
关键词
Multilayer Grad -CAM, Explainable artificial intelligence, Intelligent fault diagnosis, Vibration signals
AI 理解论文
溯源树
样例
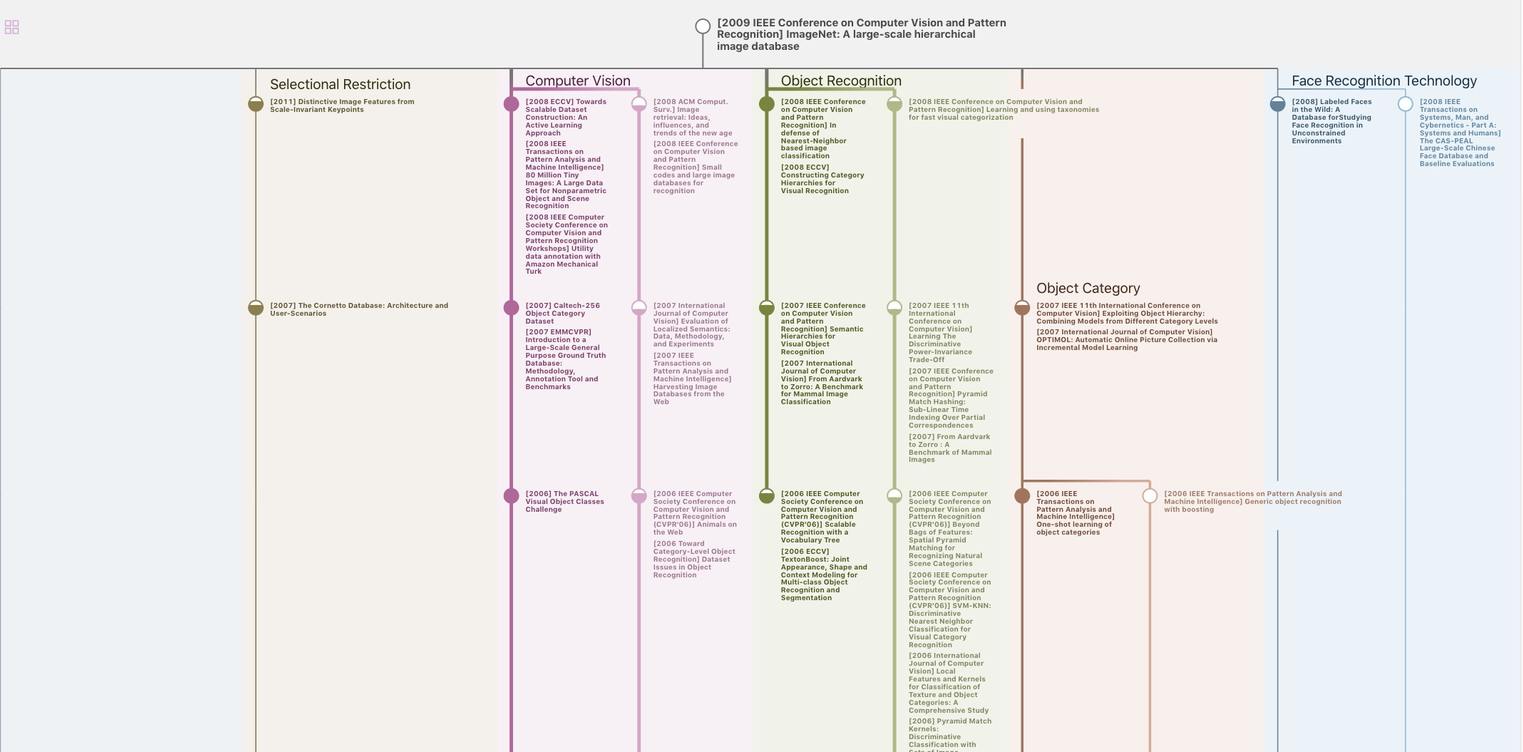
生成溯源树,研究论文发展脉络
Chat Paper
正在生成论文摘要