A deep learning approach for the inverse shape design of 2D acoustic scatterers
HEALTH MONITORING OF STRUCTURAL AND BIOLOGICAL SYSTEMS XVII(2023)
摘要
In this study, we develop an end-to-end deep learning-based inverse design approach to determine the scatterer shape necessary to achieve a target acoustic field. This approach integrates non-uniform rational B-spline (NURBS) into a convolutional autoencoder (CAE) architecture while concurrently leveraging (in a weak sense) the governing physics of the acoustic problem. By utilizing prior physical knowledge and NURBS parameterization to regularize the ill-posed inverse problem, this method does not require enforcing any geometric constraint on the inverse design space, hence allowing the determination of scatterers with potentially any arbitrary shape (within the set allowed by NURBS). A numerical study is presented to showcase the ability of this approach to identify physically-consistent scatterer shapes capable of producing user-defined acoustic fields.
更多查看译文
关键词
Remote sensing, Acoustic wavefront shaping, Deep learning, Geometric regularization
AI 理解论文
溯源树
样例
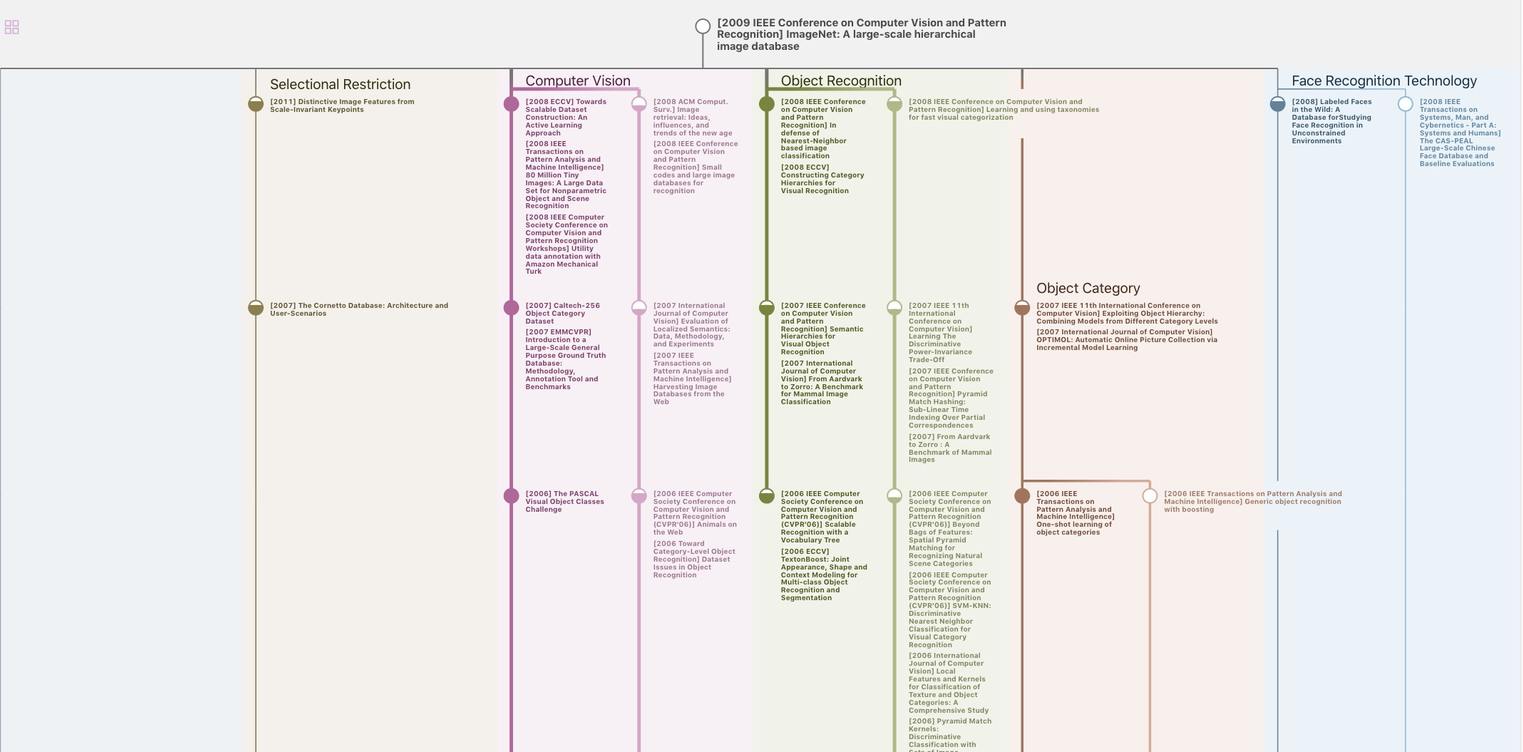
生成溯源树,研究论文发展脉络
Chat Paper
正在生成论文摘要